Augmenting IoT Healthcare Security and Reliability with Early Detection of IoT Botnet Attacks
2023 4th International Conference for Emerging Technology (INCET)(2023)
摘要
The rapid development and growth in Internet of Things technologies inspire the research community to utilize these devices for numerous types of applications. The Internet of Things with healthcare is one of the emerging domains that is motivated to enhance healthcare services by multiple Internet of Things devices in the healthcare center. The task of patient monitoring, fetching the medical laboratory results, doctor prescriptions etc. can be easily handled using Internet of things devices and gathered data can be aggregated at a server machine or using cloud services. On the other side, the Internet of Things healthcare is highly vulnerable to cyber-attacks that can lead to the compromise to the information of patients, doctors and other concerned teams. In this research, a machine learning-based framework is proposed to enhance the security and reliability of the Internet of Things healthcare by early detection of botnet attacks. The attackers target to hack Internet of things devices to generate denial-of-service attacks on critical information technology assets. The goal of the proposed methodology is to secure all internet of things devices used in the healthcare center so that the information and identity of these devices should not be breached. The Internet of Things botnet attacks can be detected using Machine learning models and with the integration of a small chip inside the Internet of Things devices, so that the entire IoT healthcare process can be secured. The proposed approach is analyzed using the random forest classifier machine learning technique as the dataset taken for Internet of things botnet attack detection contains unbalanced data. The results are evaluated using estimation metrics like precision, recall, accuracy and F1 score.
更多查看译文
关键词
Healthcare Monitoring,Sensor Network,Botnet Attack,Security,Random Forest Classifier
AI 理解论文
溯源树
样例
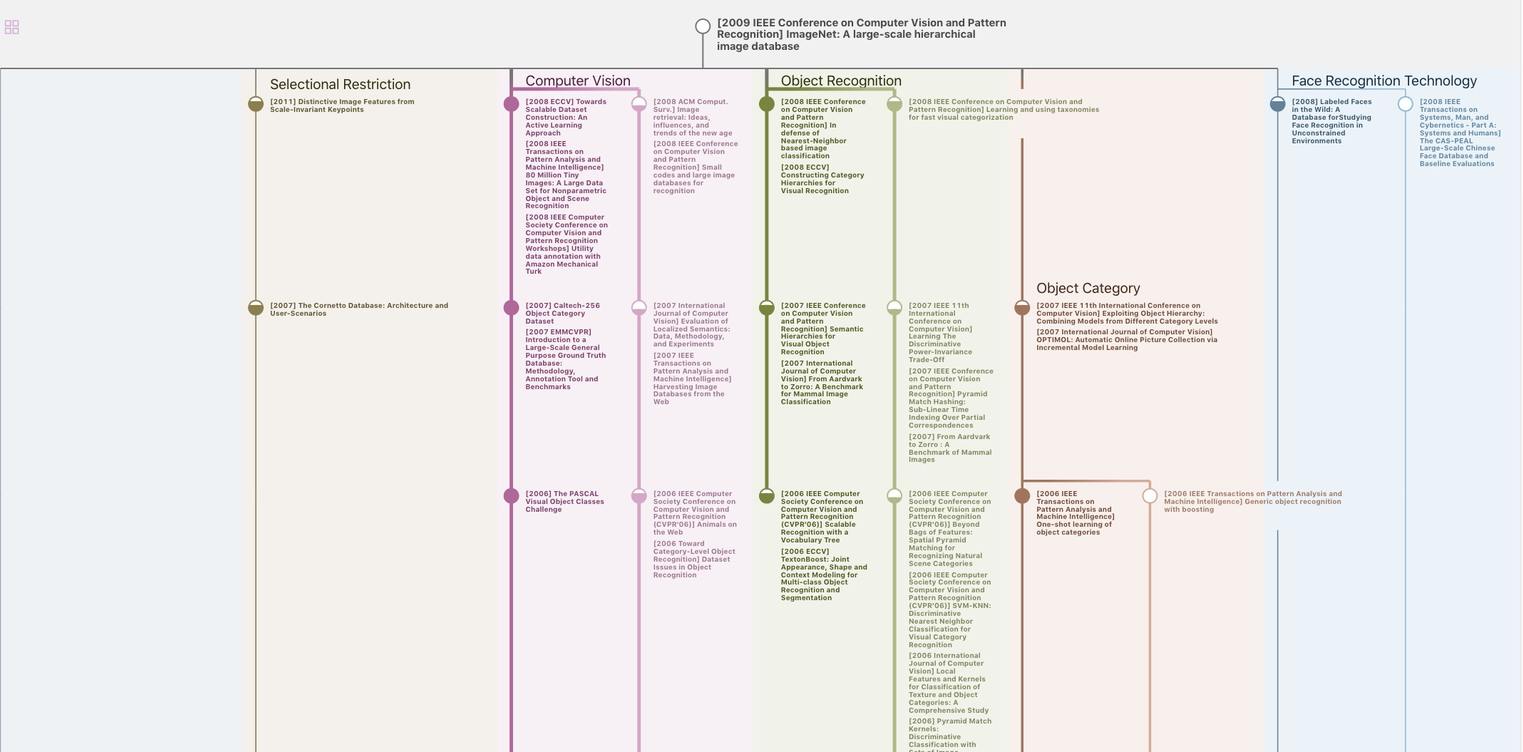
生成溯源树,研究论文发展脉络
Chat Paper
正在生成论文摘要