Supervised Change-Point Detection with Dimension Reduction
2023 31st European Signal Processing Conference (EUSIPCO)(2023)
摘要
This paper presents an automated approach for calibrating change point detection algorithms for high-dimensional time series. Our method leverages partial annotations provided by experts to learn a diagonal Mahalanobis metric, combined with a detection algorithm to replicate the expert's segmentation strategy on new signals. Our approach includes sparsity-inducing regularization to improve accuracy, which performs dimension selection and adapts to partial annotations. Our experiments on audio signals and physiological time series signals demonstrate that supervised learning improves detection accuracy significantly.
更多查看译文
关键词
change-point detection,metric learning,dimension reduction
AI 理解论文
溯源树
样例
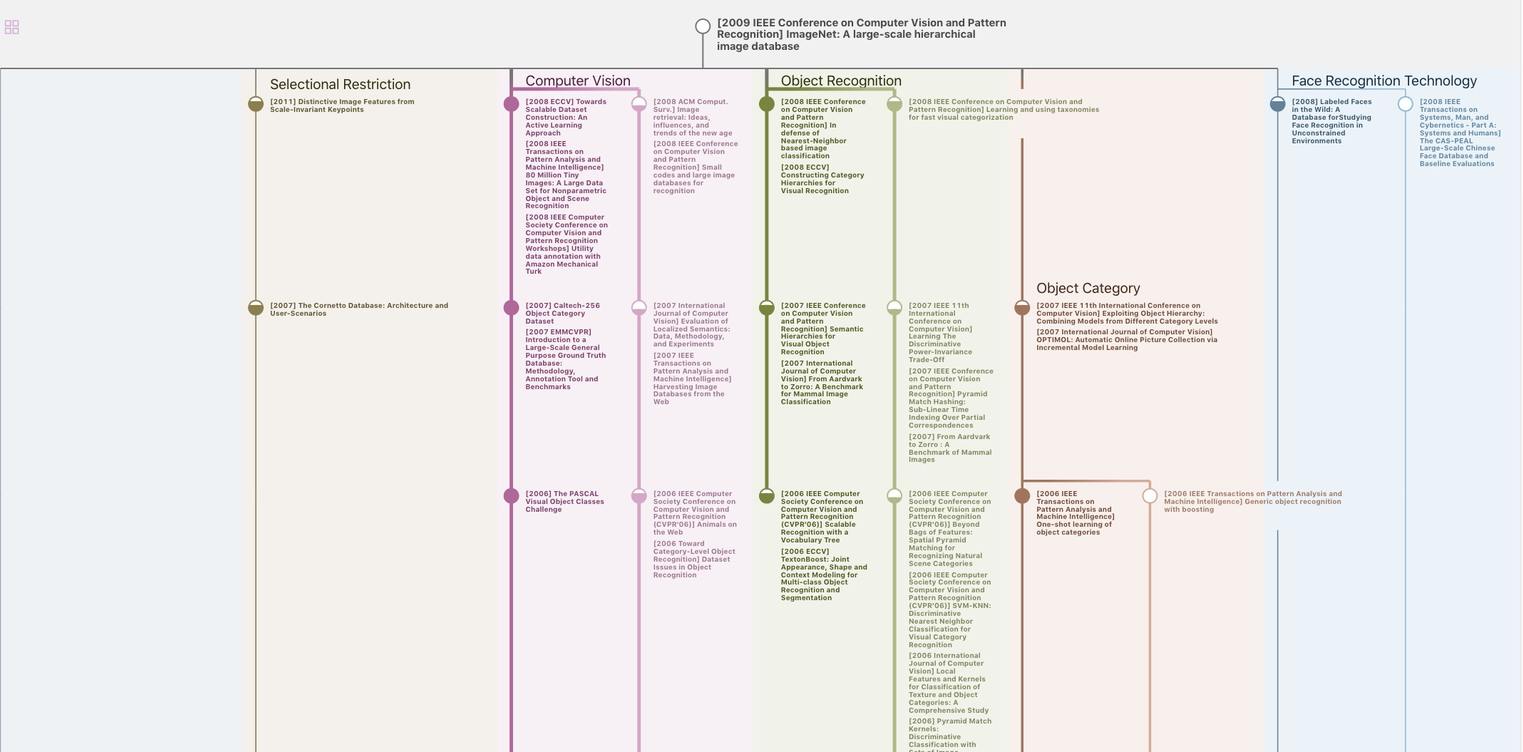
生成溯源树,研究论文发展脉络
Chat Paper
正在生成论文摘要