Sensor Data Representation with Transformer-Based Contrastive Learning for Human Action Recognition and Detection
2023 31st European Signal Processing Conference (EUSIPCO)(2023)
摘要
Feature extraction is an important process in human activity recognition (HAR) with wearable sensors. Recent studies have shown that learned features are more effective than manually engineered features in related fields. However, the scarcity and expensiveness of labeled data are limiting the development of sensor data representation learning. Our work focuses on this issue and introduces a self-supervised learning method that uses unlabeled data to improve the quality of learned sensor representations. We hypothesize that unlabeled wearable sensor data in human activities have long-term and short-term temporal contextual correlations and exploit such correlations with Transformer and Contrastive Predictive Coding (CPC) framework. The learned representation is evaluated on human activity recognition and detection tasks in real-life scenarios. The experiments show that our method outperforms previous state-of-the-art methods on MotionSense and MobiAct datasets on the HAR task and gets a remarkable performance on the EVARS dataset on the action detection task.
更多查看译文
关键词
IMU sensor,self-supervised learning,representation learning,human activity recognition,temporal action localization
AI 理解论文
溯源树
样例
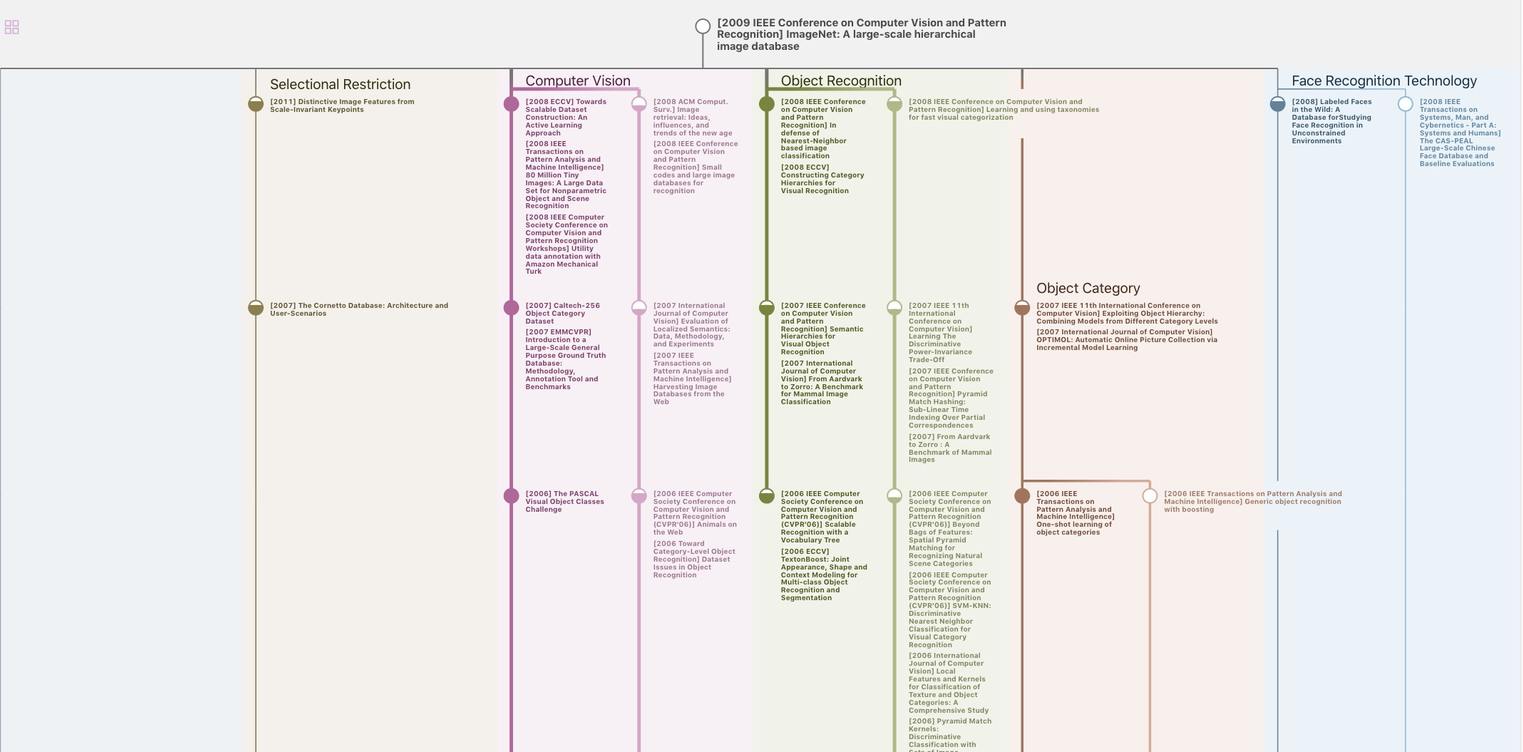
生成溯源树,研究论文发展脉络
Chat Paper
正在生成论文摘要