Proportion Inference Using Deep Neural Networks. Applications to X-Ray Diffraction and Hyperspectral Imaging
2023 31st European Signal Processing Conference (EUSIPCO)(2023)
摘要
Deep learning is considered as a disruptive method in the field of mineralogy and hyperspectral imaging. Many techniques exist to gain mineralogical information. Amongst them powder X-Ray diffraction (XRD) is very popular and powerful, while hyperspectral imaging is used in many applications such as Earth observation. A key issue for both XRD and hyperspectral imaging is not only to identify the endmembers constituting a mixture but also quantify the abundance of each endmember. In this study, we propose completely novel neural network (NN) training losses specifically designed for proportion inference. Extensive experiments illustrate that the proposed approach allows validated NN architectures to be trained to infer accurately on proportions.
更多查看译文
关键词
Proportion inference,Hyperspectral Unmixing,X-Ray Diffraction,Neural Networks,Dirichlet distribution
AI 理解论文
溯源树
样例
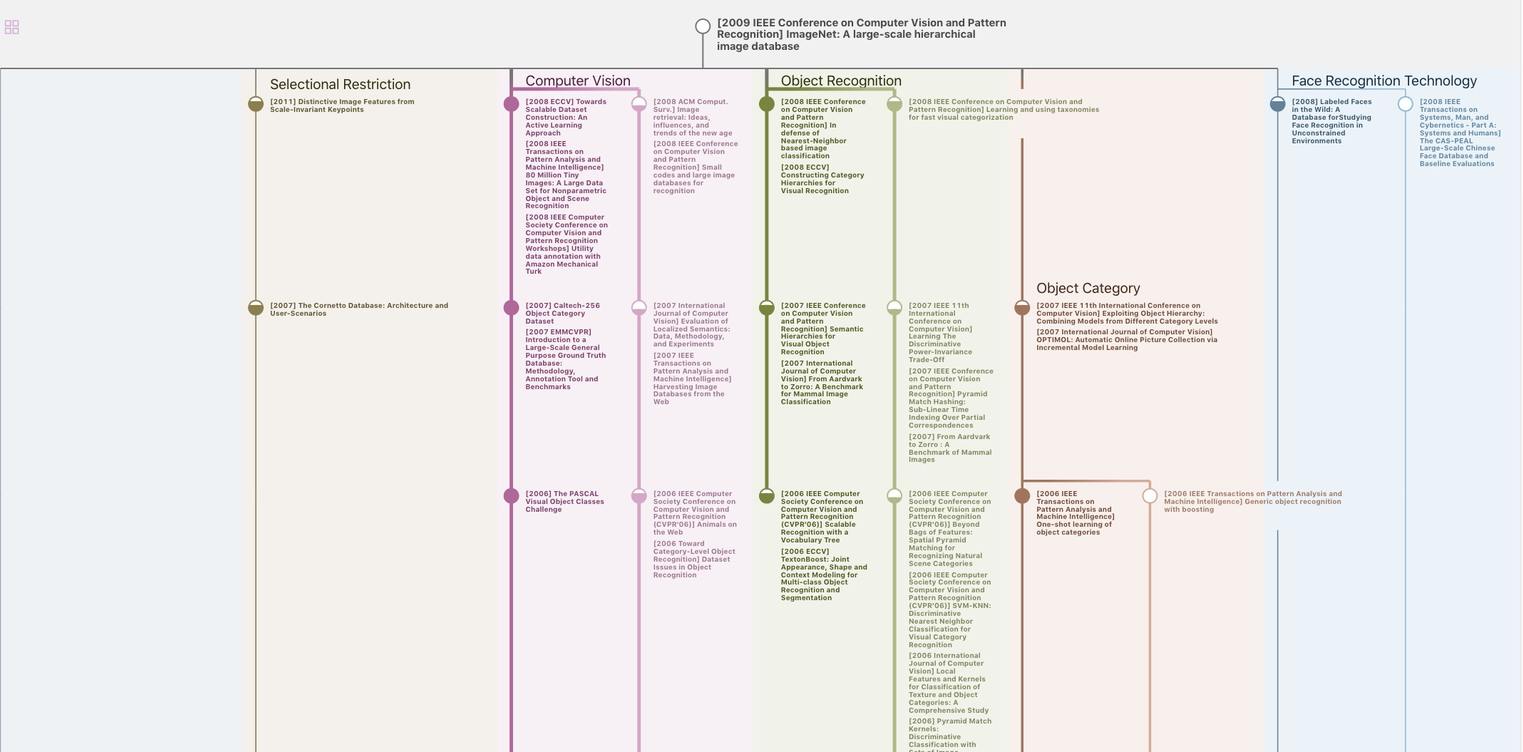
生成溯源树,研究论文发展脉络
Chat Paper
正在生成论文摘要