TinyML optimization for activity classification on the resource-constrained body sensor BI-Vital
2023 IEEE 19TH INTERNATIONAL CONFERENCE ON BODY SENSOR NETWORKS, BSN(2023)
摘要
Addressing the demand for scalable and efficient deployment of machine learning (ML) models on mobile devices and especially microcontrollers, this research introduces a hardware-in-the-loop (HIL) deployment setup within the producer-consumer software architecture of the BI-Vital, a chest-mounted body sensor designed by our research group. The sensor provides real-time monitoring of various physiological and environmental parameters, enhanced by the integration of tiny machine learning (TinyML). Leveraging the UCI-HAR dataset for Human Activity Recognition (HAR), this study focuses on optimizing ML models' hyperparameters to balance inference time, memory, accuracy, and power consumption. An efficiency score E is proposed to assess models in this unique context. The results illustrate the trade-offs between the models: Decision Trees offer reduced power usage, Multilayer Perceptrons ensure high accuracy with minimal memory requirements, while Convolutional Neural Networks present limitations due to extended inference times. The results emphasize the potential of TinyML in wearable physiological monitoring, especially for optimizing models for resource-limited devices in real-world applications.
更多查看译文
关键词
wireless body sensor node,TinyML,human activity recognition,producer-consumer architecture,wearable
AI 理解论文
溯源树
样例
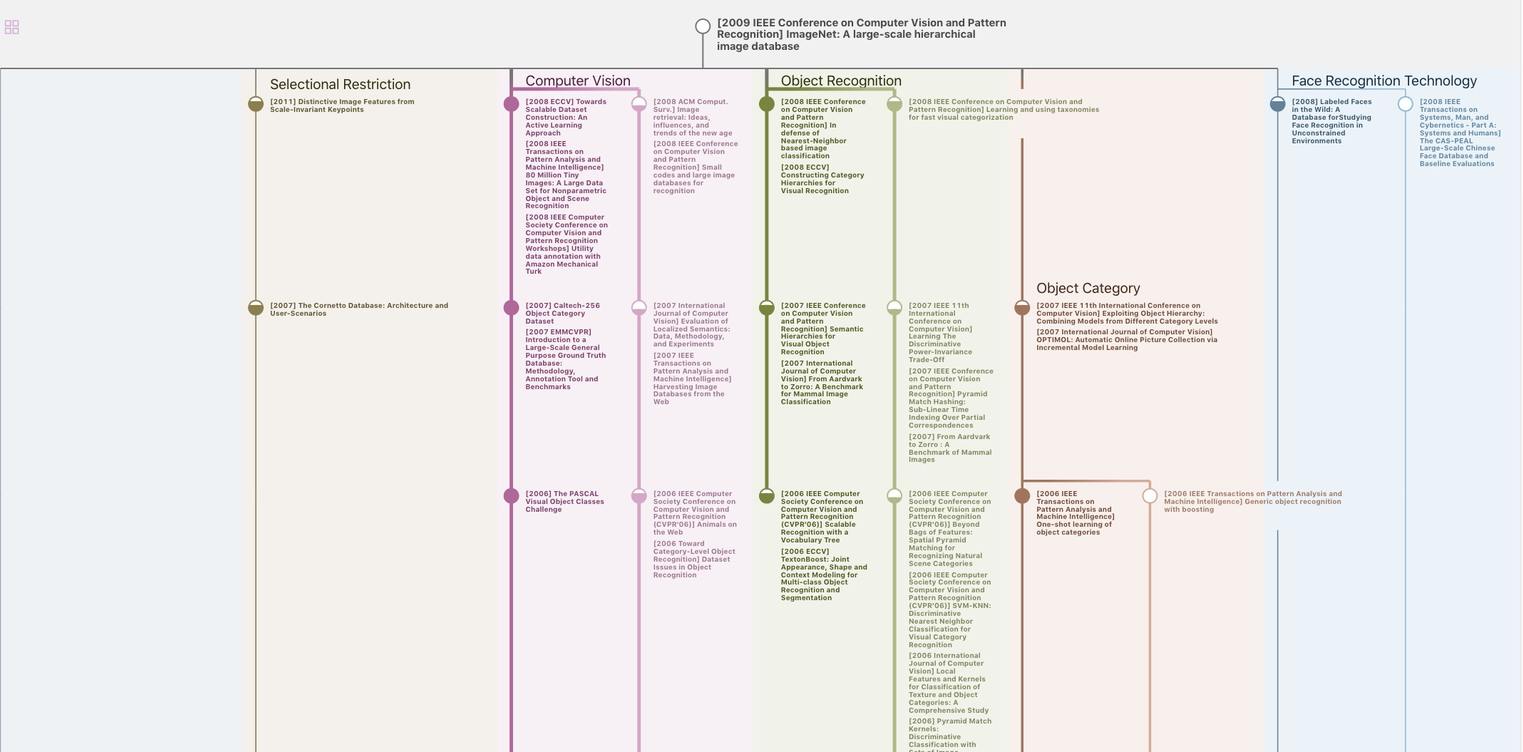
生成溯源树,研究论文发展脉络
Chat Paper
正在生成论文摘要