A Data-Driven Digital Demodulator Based on Deep Learning for Radio Over Fiber Transmission System
JOURNAL OF LIGHTWAVE TECHNOLOGY(2023)
摘要
data-driven digital demodulator based on a Fourier layer Transformer network (FTnet) for radio over fiber (RoF) transmission system with quadrature amplitude modulation (QAM) is developed and experimentally demonstrated. The FT net combines the Transformer encoder with the Fourier layer to learn the waveforms and directly recover the bitstreams from the impaired received signals. The FTnet-based demodulator does not rely on a series of digital demodulation algorithms such as frequency offset compensation, down-conversion, equalization, and decoding, making the process more efficient and accurate. The 10 GHz 2 Gsym/s 25 km RoF transmission systems are established to evaluate the proposed FTnet-based digital demodulator experimentally. The results show that the bit error rates (BERs) performance of the proposed demodulator for the 16-QAM RoF is better than the ones based on a fully connected neural network, Transformer, and traditional digital demodulator with the least mean square error equalizer (TDD-LMS). The optical receiving sensitivity for the 64-QAM RoF system based on the proposed demodulator is improved by 3 dB compared to TDD-LMS under a BER limit of 3.8 x 10(-3). Furthermore, our proposed demodulator outperforms other demodulators for the RoF system with wireless transmission at different received optical powers and wireless distances.
更多查看译文
关键词
Demodulation,Transformers,Optical fiber networks,Feature extraction,Optical receivers,Optical filters,Symbols,Data-driven,deep learning,digital demodulator,Fourier layer,radio over fiber,transformer,waveform learning
AI 理解论文
溯源树
样例
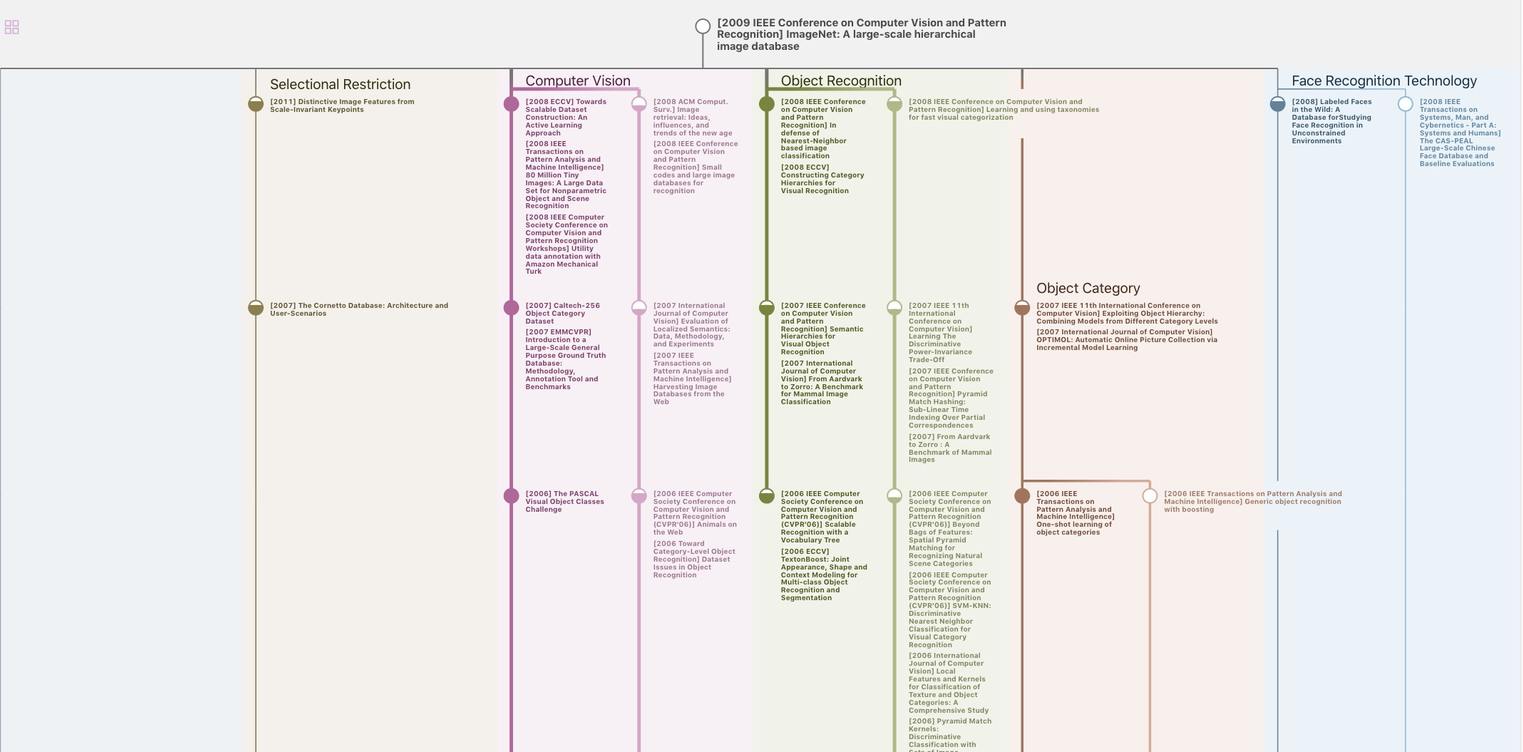
生成溯源树,研究论文发展脉络
Chat Paper
正在生成论文摘要