Predictive function of tumor burden-incorporated machine-learning algorithms for overall survival and their value in guiding management decisions in patients with locally advanced nasopharyngeal carcinoma
JOURNAL OF THE NATIONAL CANCER CENTER(2023)
摘要
Objective: Accurate prognostic predictions and personalized decision-making on induction chemotherapy (IC) for individuals with locally advanced nasopharyngeal carcinoma (LA-NPC) remain challenging. This research examined the predictive function of tumor burden-incorporated machine-learning algorithms for overall survival (OS) and their value in guiding treatment in patients with LA-NPC.Methods: Individuals with LA-NPC were reviewed retrospectively. Tumor burden signature-based OS prediction models were established using a nomogram and two machine-learning methods, the interpretable eXtreme Gradient Boosting (XGBoost) risk prediction model, and DeepHit time-to-event neural network. The models' prediction performances were compared using the concordance index (C-index) and the area under the curve (AUC). The patients were divided into two cohorts based on the risk predictions of the most successful model. The efficacy of IC combined with concurrent chemoradiotherapy was compared to that of chemoradiotherapy alone.Results: The 1 221 eligible individuals, assigned to the training ( n = 813) or validation ( n = 408) set, showed significant respective differences in the C-indices of the XGBoost, DeepHit, and nomogram models (0.849 and 0.768, 0.811 and 0.767, 0.730 and 0.705). The training and validation sets had larger AUCs in the XGBoost and DeepHit models than the nomogram model in predicting OS (0.881 and 0.760, 0.845 and 0.776, and 0.764 and 0.729, P < 0.001). IC presented survival benefits in the XGBoost-derived high-risk but not low-risk group.Conclusion: This research used machine-learning algorithms to create and verify a comprehensive model integrating tumor burden with clinical variables to predict OS and determine which patients will most likely gain from IC. This model could be valuable for delivering patient counseling and conducting clinical evaluations.
更多查看译文
关键词
Nasopharyngeal carcinoma,Machine learning,Tumor burden,Prognostic model,Induction chemotherapy
AI 理解论文
溯源树
样例
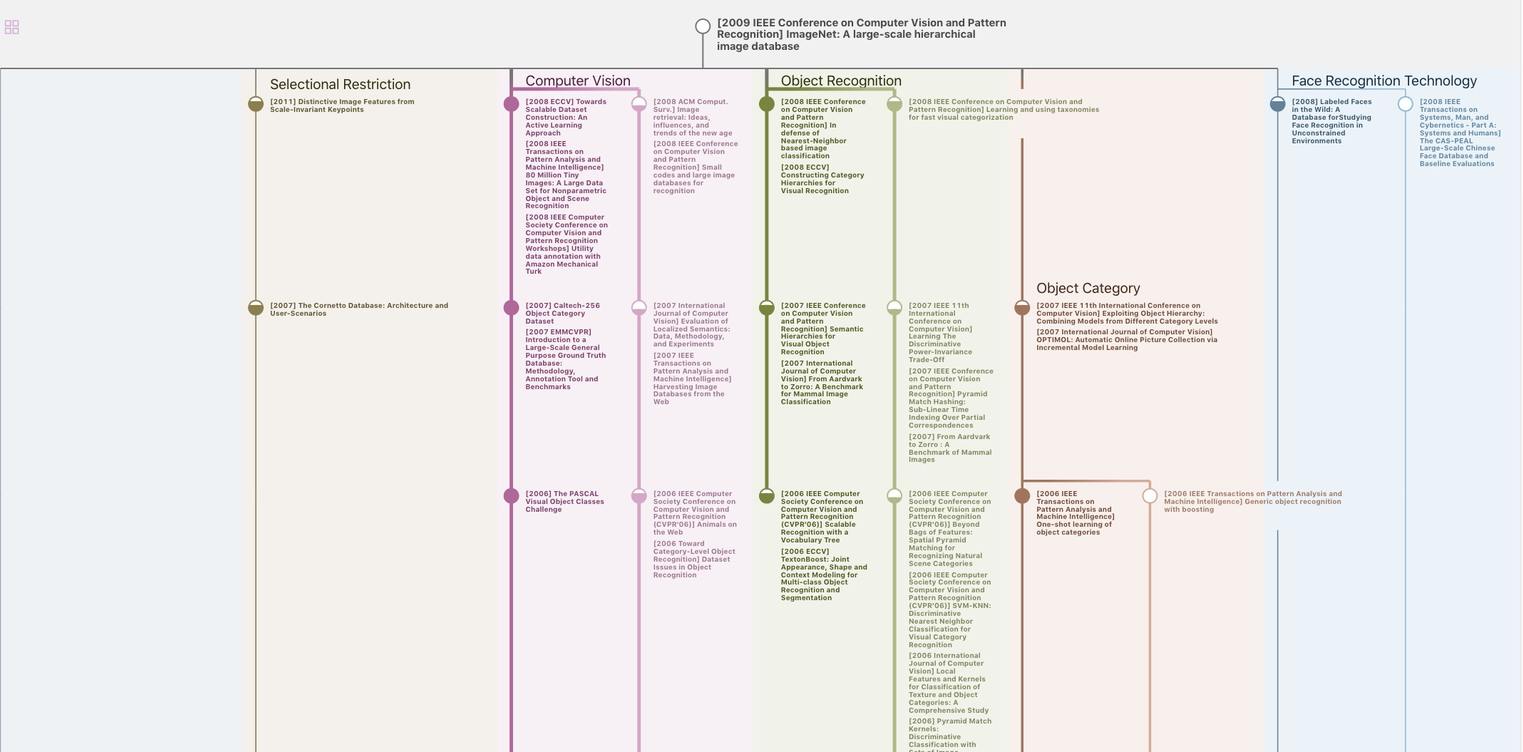
生成溯源树,研究论文发展脉络
Chat Paper
正在生成论文摘要