A Transfer Learning Framework for High-Accurate Cross-Workload Design Space Exploration of CPU
2023 IEEE/ACM INTERNATIONAL CONFERENCE ON COMPUTER AIDED DESIGN, ICCAD(2023)
摘要
To perform cross-workload design space exploration of CPU, previous works implicitly transfer knowledge from several existing source workloads and try to make predictions on the target one. However, they do not fully explore the transferability across workloads and their single basic prediction models limit the prediction accuracy. In this paper, an open-source Transfer learning Ensemble Design Space Exploration framework (TrEnDSE) is proposed to perform cross-workload performance predictions. The black-box transferability between workloads is quantitatively dissected and explicitly utilized as sample weights for training. Moreover, an ensemble bagging learning model and an uncertainty-driven iterative optimization method are proposed to perform accurate and robust prediction, with these sample weights leveraged. Experiments on SPEC CPU 2017 demonstrate that TrEnDSE can reduce cycle per instruction prediction error by 54% and power prediction error by 34% compared with the state-of-the-art work.
更多查看译文
关键词
Design Space Exploration,Cross-workload,Prediction Model,Transfer Learning,CPU Microarchitecture
AI 理解论文
溯源树
样例
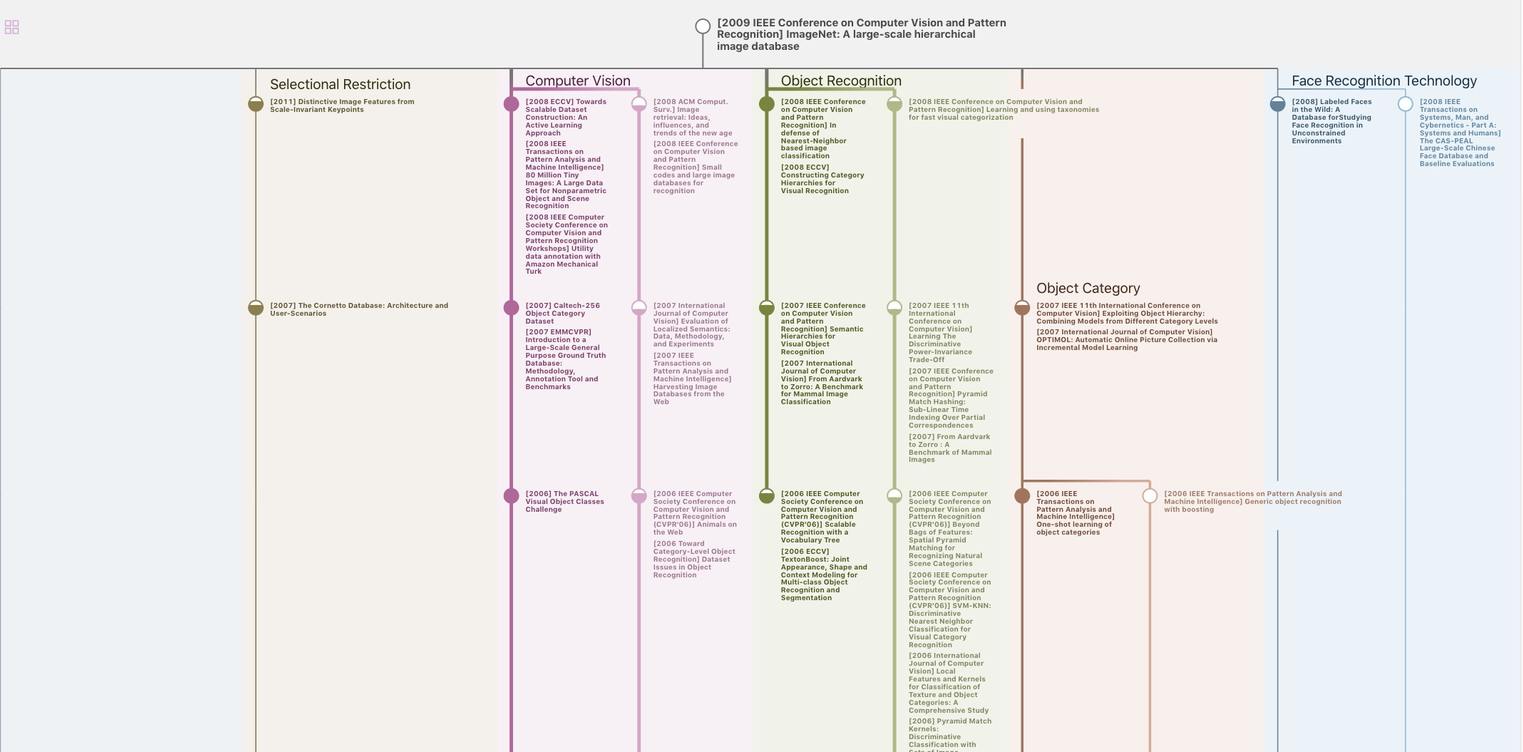
生成溯源树,研究论文发展脉络
Chat Paper
正在生成论文摘要