TRAIN: A Reinforcement Learning Based Timing-Aware Neural Inference on Intermittent Systems
2023 IEEE/ACM INTERNATIONAL CONFERENCE ON COMPUTER AIDED DESIGN, ICCAD(2023)
摘要
Intermittent systems become popular to be considered as the solutions of various application domains, thanks to the maturation of energy harvesting technology. Environmental monitoring is such an example and it is a time-sensitive application domain. In order to report the perceived environmental status in a timely manner, methods have been proposed to consider the freshness of the collected information on such systems with unstable power sources. Nevertheless, these methods cannot be applied to neural network workloads since these methods do not consider the delivered model accuracy. On the other hand, while there have been studies for deploying neural network applications on intermittent systems, they depend on branchy network architectures, each branch representing an energy-accuracy tradeoff, and do not take into account a time constraint, which tends to cause system failures because of the frequent generation of expired data. In this work, the first timing-aware framework TRAIN is proposed to deploy the neural network models on the intermittent systems by considering energy, time constraint, and delivered model accuracy. Compared with the prior studies that depend on branchy network architectures, TRAIN offers a broadened solution space representing various energy/time/accuracy tradeoffs. It is achieved by allowing to choose among different implementations of each model layer during the model inference at runtime, and the smart choices are made by the proposed reinforcement learning algorithm. Our results demonstrate TRAIN outperforms the prior study by 65%, regarding the delivered model accuracy. We believe that TRAIN paves the way for building complex applications on intermittent systems.
更多查看译文
关键词
intermittent systems,energy harvesting (EH),deep neural network (DNN),multi-exits,reinforcement learning (RL)
AI 理解论文
溯源树
样例
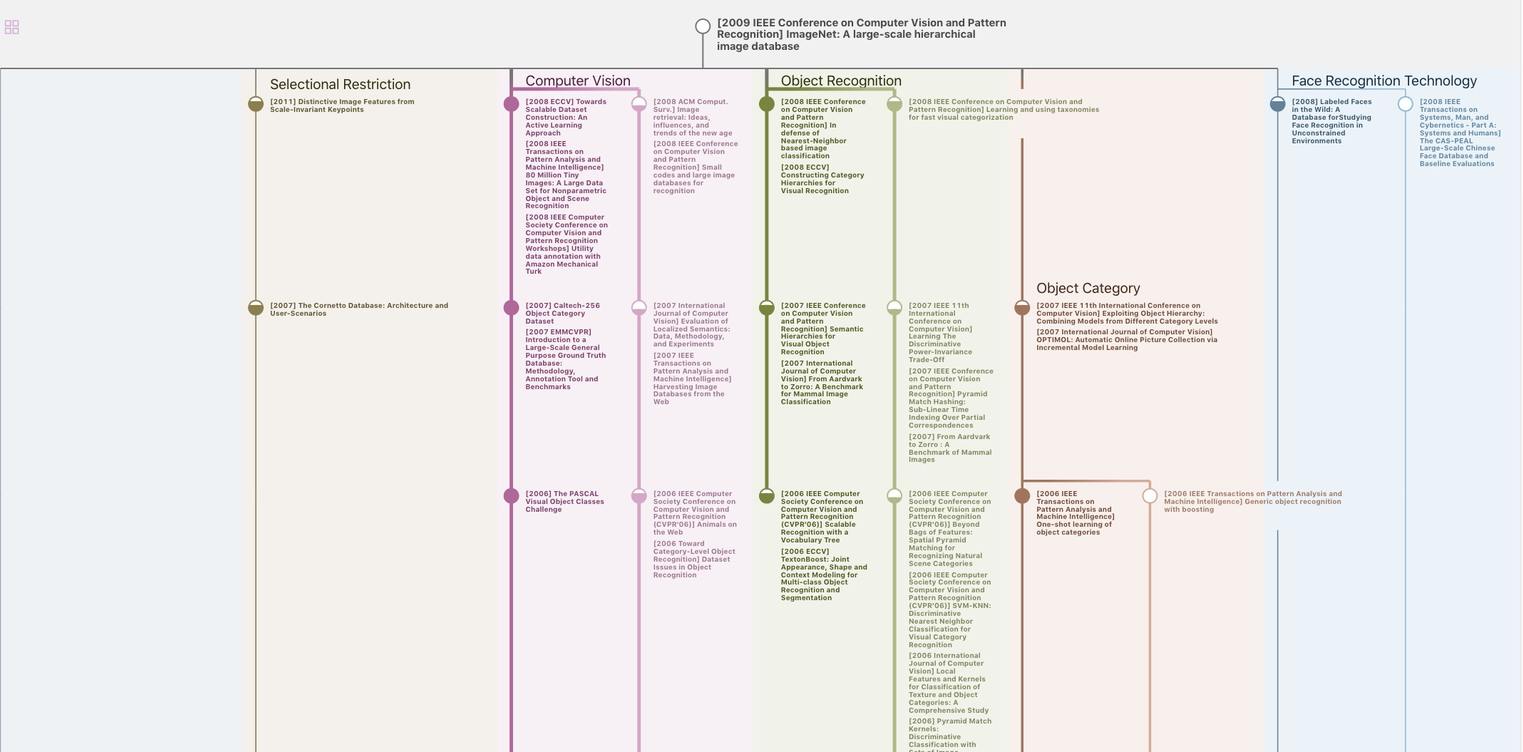
生成溯源树,研究论文发展脉络
Chat Paper
正在生成论文摘要