RMFENet: Refined Multiscale Feature Enhancement Network for Arbitrary-Oriented Sonar Object Detection
IEEE SENSORS JOURNAL(2023)
摘要
Automatic underwater vehicle (AUV) object detection in sonar images has drawn widespread interest for its practical applications. Nevertheless, accurate detection remains challenging due to the scale imbalance and arbitrary orientations of underwater objects with extreme aspect ratios. In this article, we introduce a refined multiscale feature enhancement network (RMFENet) framework as a novel approach to achieve high-precision performance in detecting arbitrary-oriented underwater objects. First, we develop a data preprocessing module to adaptively adjust large-size sonar images to the appropriate size and direction angle. Second, we construct a composite backbone network (CB-Net) that refines the integration of high-level and low-level features in sonar images and enhances the correlation of different features. Third, the shuffle convolutional block attention mechanism (Shuffle-CBAM) mechanism and the diverse multiscale feature fusion (DMSFF) block further enhance the model feature extraction ability. Finally, to generate more accurate rotation region proposals and anchors, we propose a rotated intersection over union (RIoU) to solve inaccurate object region localization problems and high computational complexity in existing methods. Besides, our model is constructed on two sonar image datasets for oriented underwater object detection with different spatial resolutions, diverse object categories, and bounding box annotation. Extensive experiments demonstrate that our method can achieve state-of-the-art performance on the constructed sonar image object detection datasets while keeping better robustness.
更多查看译文
关键词
Attention mechanism,feature enhancement,feature fusion,object detection,sonar images
AI 理解论文
溯源树
样例
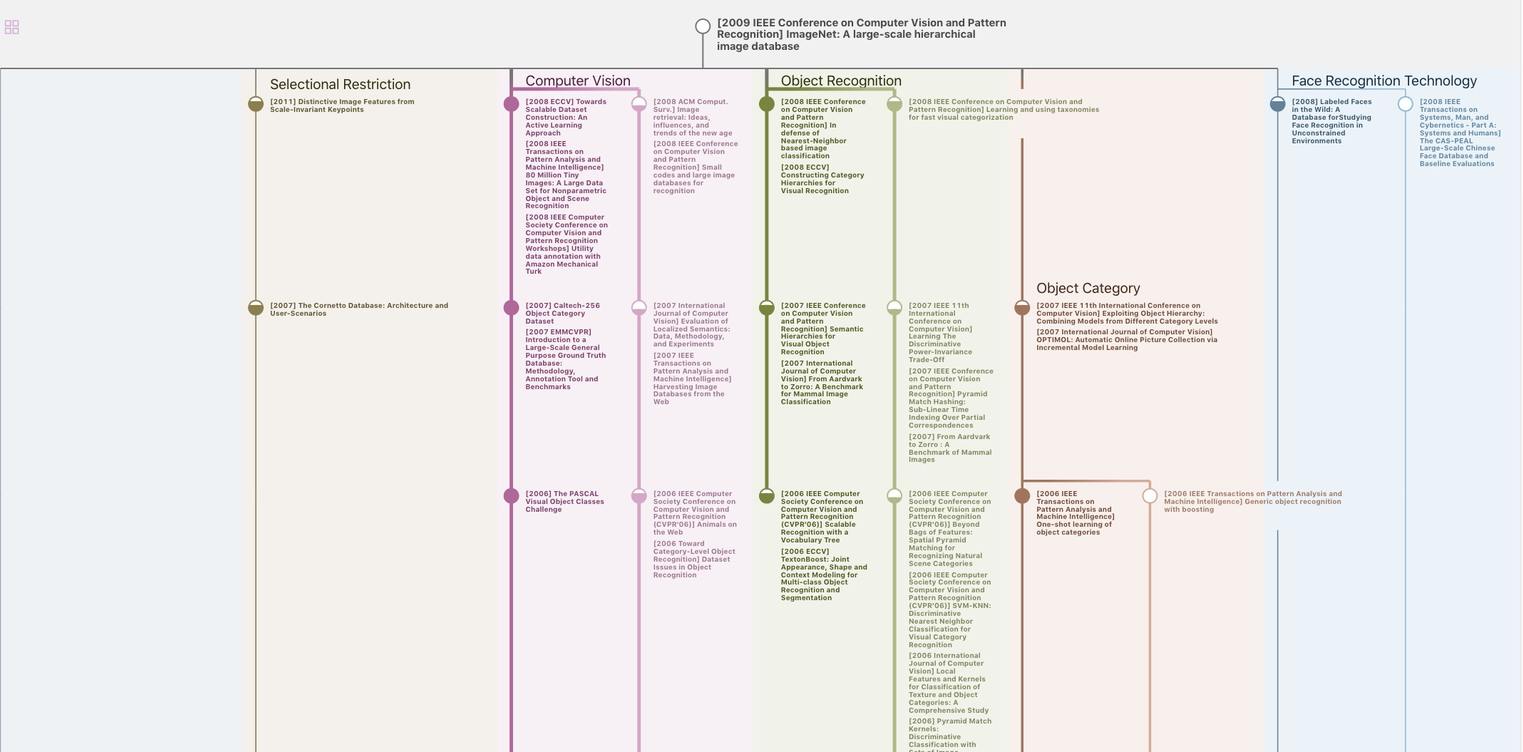
生成溯源树,研究论文发展脉络
Chat Paper
正在生成论文摘要