Multi-task Contexture Learning Network for automated vertebrae segmentation and tumor diagnosis from MRI
COMPUTERS & ELECTRICAL ENGINEERING(2024)
摘要
Automated vertebrae segmentation and tumor diagnosis from magnetic resonance imaging (MRI) scans play a crucial role in early detection of spinal tumors. However, the task is challenging due to the variability in fields of view across MRI images and the diverse appearances of tumors. To address these challenges, we propose a novel approach, the Multitask Contexture Learning Network (MCLN), which concurrently performs accurate vertebrae segmentation and precise identification of tumors. Specifically, MCLN first constructs a spatial pyramid graph convolutional network and a label attention network to effectively capture long-range contextual information and generate a precise segmentation mask. Then, MCLN incorporates the input MRI image and the segmentation mask to recognize vertebral tumors utilizing a mixed kernel multi-loss network. We evaluate our proposed MCLN on a challenging dataset consisting of 600 MRI images, achieving impressive results with a mean Dice coefficient of 90.03% for vertebrae segmentation and a mean accuracy of 95.11% for tumor diagnosis. These results demonstrate the remarkable performance of MCLN in addressing automated vertebrae segmentation and tumor diagnosis from MRI.
更多查看译文
关键词
MRI,Vertebrae tumor diagnosis,Vertebrae segmentation,Graph convolutional network,Long-range contextual information,Attention mechanism
AI 理解论文
溯源树
样例
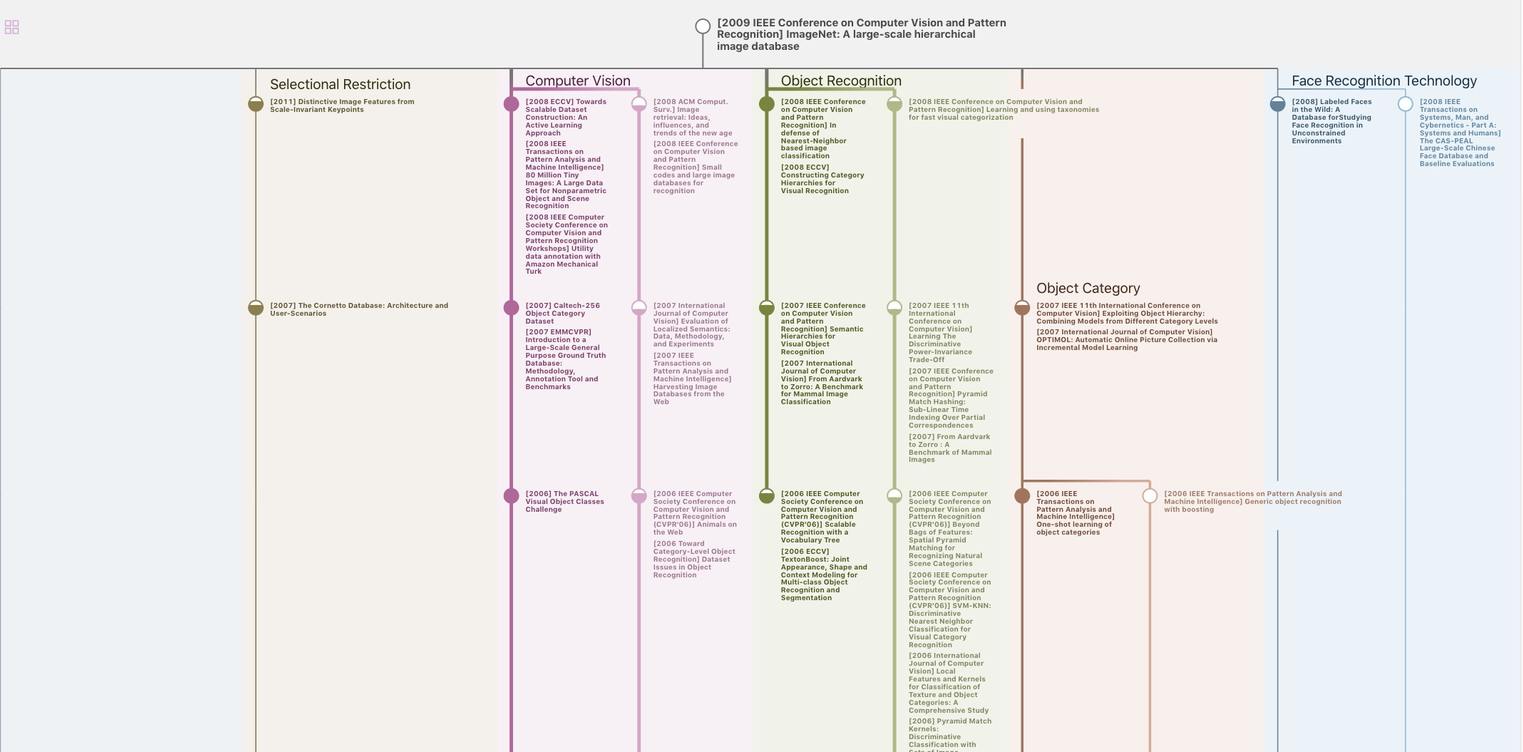
生成溯源树,研究论文发展脉络
Chat Paper
正在生成论文摘要