Unravelling the Impact of Metal Dopants and Oxygen Vacancies on Syngas Conversion over Oxides: A Machine Learning-Accelerated Study of CO Activation on Cr-Doped ZnO Surfaces
ACS CATALYSIS(2023)
摘要
As a critical component of the OX-ZEO composite catalysts toward syngas conversion, the Cr-doped ZnO ternary system can be considered as a model system for understanding oxide catalysts. However, due to the complexity of its structures, traditional approaches, both experimental and theoretical, encounter significant challenges. Herein, we employ machine learning-accelerated methods, including grand canonical Monte Carlo and genetic algorithm, to explore the ZnO(1010) surface with various Cr and oxygen vacancy (OV) concentrations. Stable surfaces with varied Cr and OV concentrations were then systematically investigated to examine their influence on the CO activation via density functional theory calculations. We observe that Cr tends to preferentially appear on the surface of ZnO(1010) rather than in its interior regions and Cr-doped structures incline to form rectangular islands along the [0001] direction at high Cr and OV concentrations. Additionally, detailed calculations of CO reactivity unveil an inverse relationship between the reaction barrier (E-a) for C-O bond dissociation and the Cr and OV concentrations, and a linear relationship is observed between OV formation energy and E-a for CO activation. Further analyses indicate that the C-O bond dissociation is much more favored when the adjacent OVs are geometrically aligned in the [1210] direction, and Cr is doped around the reactive sites. These findings provide a deeper insight into CO activation over the Cr-doped ZnO surface and offer valuable guidance for the rational design of effective catalysts for syngas conversion.
更多查看译文
关键词
oxygen vacancy,Cr doping,CO activation,machine learning potential,genetic algorithm,DFT,syngas conversion
AI 理解论文
溯源树
样例
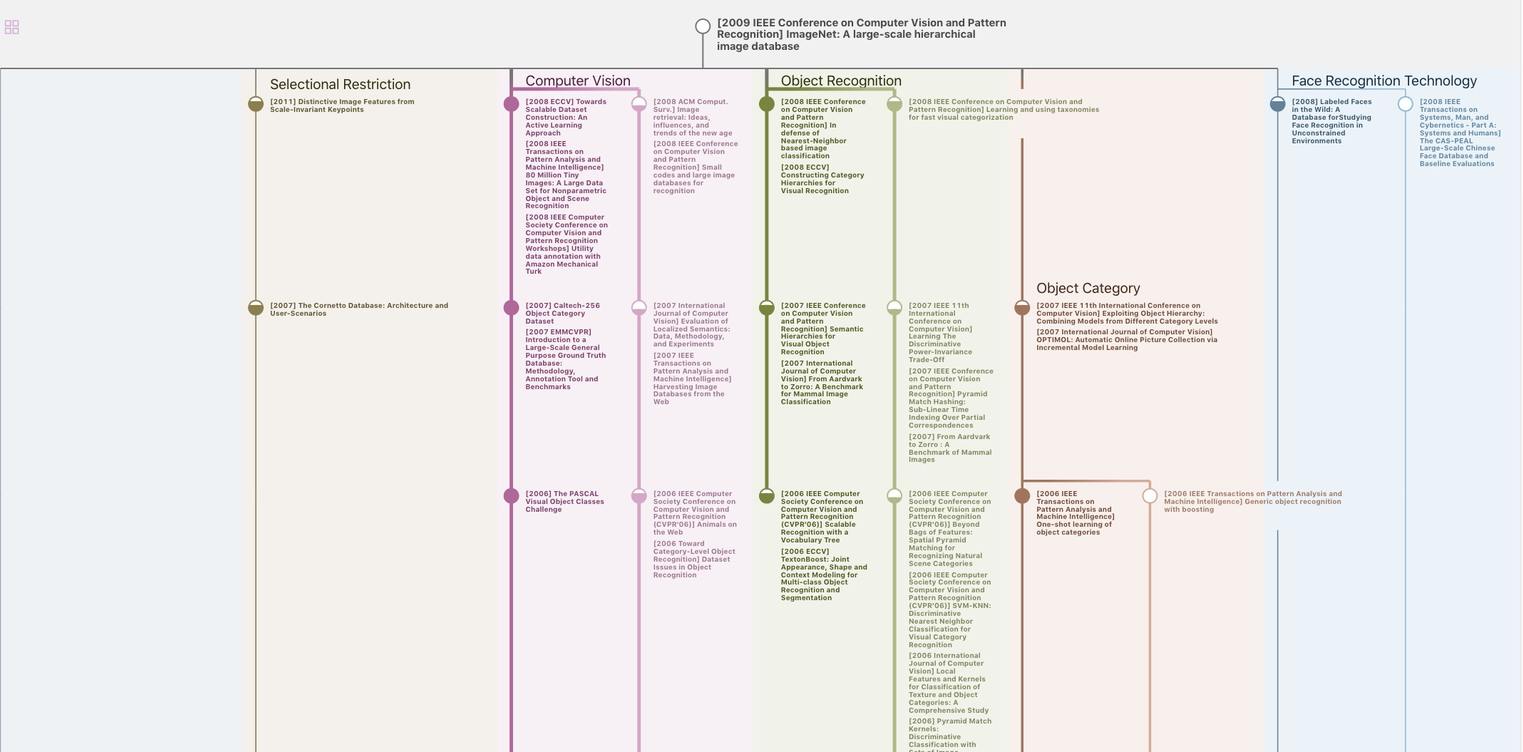
生成溯源树,研究论文发展脉络
Chat Paper
正在生成论文摘要