Deep Neural Network Based Object Detection Algorithm With optimized Detection Head for Small Targets
2023 IEEE International Conference on Mechatronics and Automation (ICMA)(2023)
摘要
Target detection is a significant research direction in the realm of computer vision, aimed at analyzing and localizing targets in input images to obtain their categories and locations. However, this task is faced with several challenges in complex scenarios, such as large variations in target scales, similarity to the background, dense number, and overlapping with each other, making it difficult for the target detection model to extract different scales and discriminative target features adaptively, leading to low detection accuracy and weak generalization ability. To tackle the aforementioned problems, this study proposes a novel approach by optimizing the detection head of the model, invoking decoupled detection heads, dividing the classification and localization tasks, and optimizing for small targets. This approach effectively reduces the false detection rate and the missed detection rate, enabling the network to better discriminate and recognize distant objects as well as objects of relatively small size. Moreover, the proposed approach outperforms the yolov7 model by 0.8% in the COCO dataset, not only improving the accuracy of detecting distant and small targets but also exhibiting better performance in conventional target detection. Additionally, this approach is capable of handling small targets, overlapping targets, or relatively dense targets, significantly expanding its practical use range.
更多查看译文
关键词
Target Detection Techniques,Small Target Detection,Yolov7,Decoupled detectors
AI 理解论文
溯源树
样例
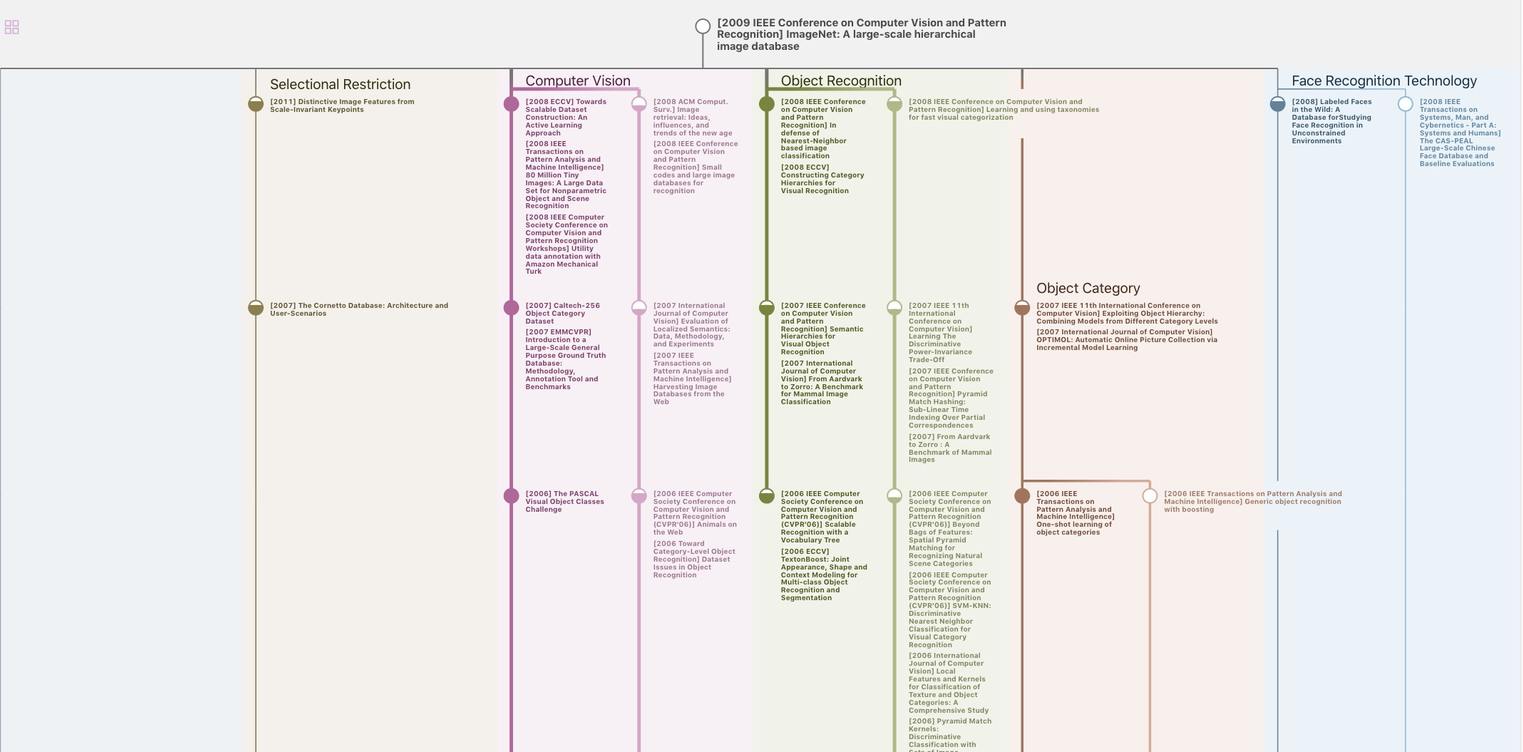
生成溯源树,研究论文发展脉络
Chat Paper
正在生成论文摘要