Fatigue Driving Detection of EEG Signals by LSTM Deep Neural Network with LPSD and DE
2023 IEEE International Conference on Mechatronics and Automation (ICMA)(2023)
摘要
Fatigue driving detection has always been an urgent social problem to be solved, and many complex factors increase the difficulty of detection. Nowadays, more scholars choose to use multi-channel EEG signals for fatigue detection, which is accompanied by the development of deep learning networks. Here, we propose the deep Long Short Term Memory (LSTM) neural network with the Differential Entropy (DE) and Logarithm Power Spectrum Density (LPSD). We first divide the EEG signal into 8-s Hanning windows with 50% overlap, and then use the Short Time Fourier Transform(STFT) on the extracted time window to obtain 50 frequency sub-bands. Finally, LPSD and DE features are extracted from the frequency offspring obtained from the STFT and input into the deep LSTM neural network. The network can choose to learn more task related information by adding soft attention and extract effective time information from EEG signals,thereby improving the model’s performance in detecting driving fatigue. In addition, we also collected EEG signals from 8 subjects in both awake and fatigued states,and used the PERCLOS index to judge the degree of fatigue. Using the collected dataset, we compared the effectiveness of the deep LSTM neural network with five other methods. The results indicate that the deep LSTM neural network has a better classification accuracy of 93.12%. It proves that the network has good performance in fatigue detection of EEG signals.
更多查看译文
关键词
EEG,Fatigue Detection,LSTM,DE,LPSD
AI 理解论文
溯源树
样例
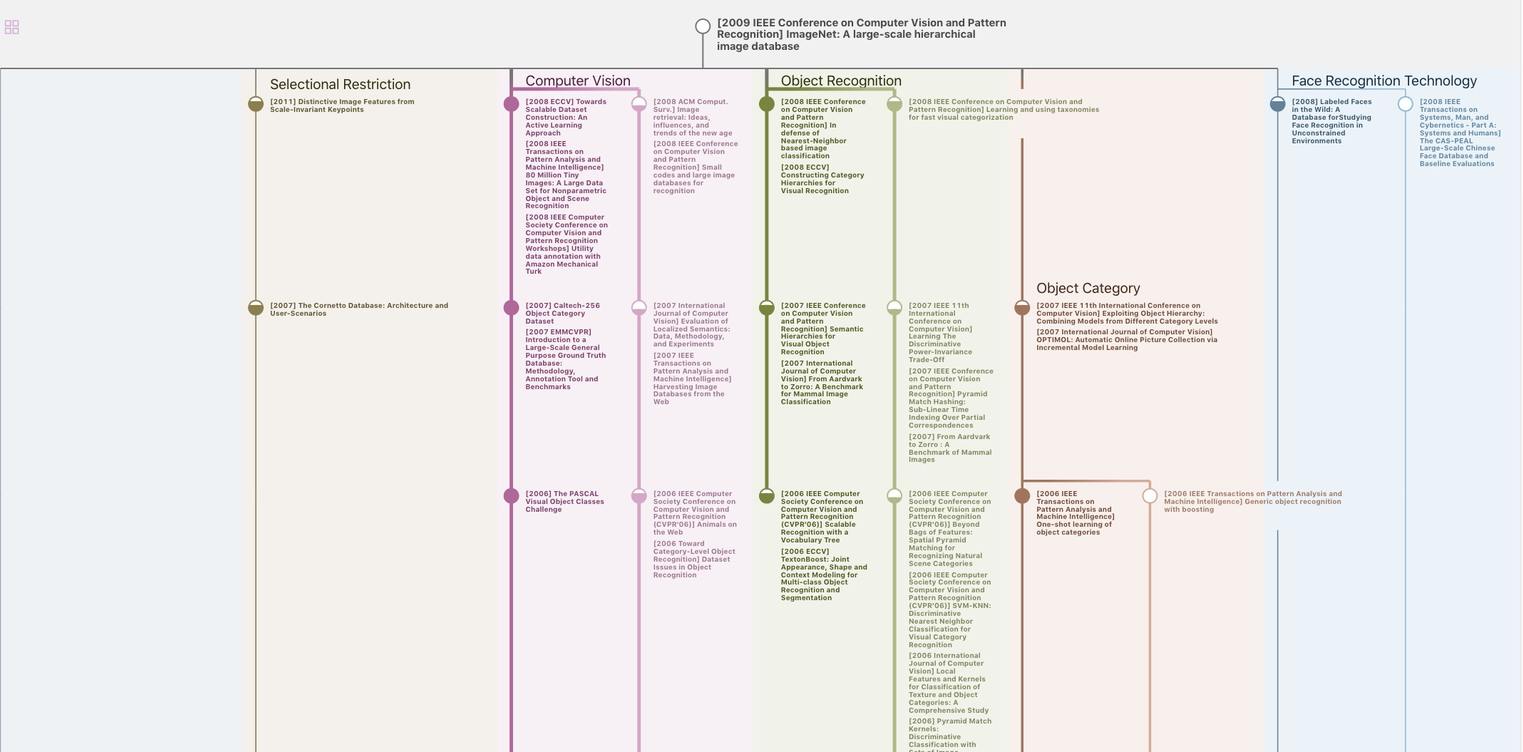
生成溯源树,研究论文发展脉络
Chat Paper
正在生成论文摘要