Delving Deeper Into Image Dehazing: A Survey
IEEE ACCESS(2023)
摘要
Images captured under foggy or hazy weather conditions are affected by the scattering of atmospheric particles, resulting in decreased contrast and color variation, thereby limiting their practical applications. In recent years, deep learning methods showcase significant advancements in image dehazing. However, the complexity and degradation factors in hazy images challenge the generalization capacity of dehazing methods. This paper comprehensively reviews the recent developments in single-image dehazing techniques based on deep learning. From the perspectives of Convolutional Neural Networks (CNN) and Generative Adversarial Networks (GAN), different models are introduced and classified into four categories: Encoder-Decoder, Multi-Module, Multi-Branch, and Dual-Generative Adversarial Networks. The robustness and effectiveness of deep learning models are analyzed by comparing their performance and model complexity on public datasets. Additionally, limitations of current benchmark datasets and evaluation metrics are identified, and unresolved issues and future research directions are discussed. Our efforts in this paper will serve as a comprehensive reference for future research and call for further development in deep learning-based image dehazing.
更多查看译文
关键词
Image dehazing,deep learning,convolutional neural networks (CNNs),generative adversarial networks (GANs)
AI 理解论文
溯源树
样例
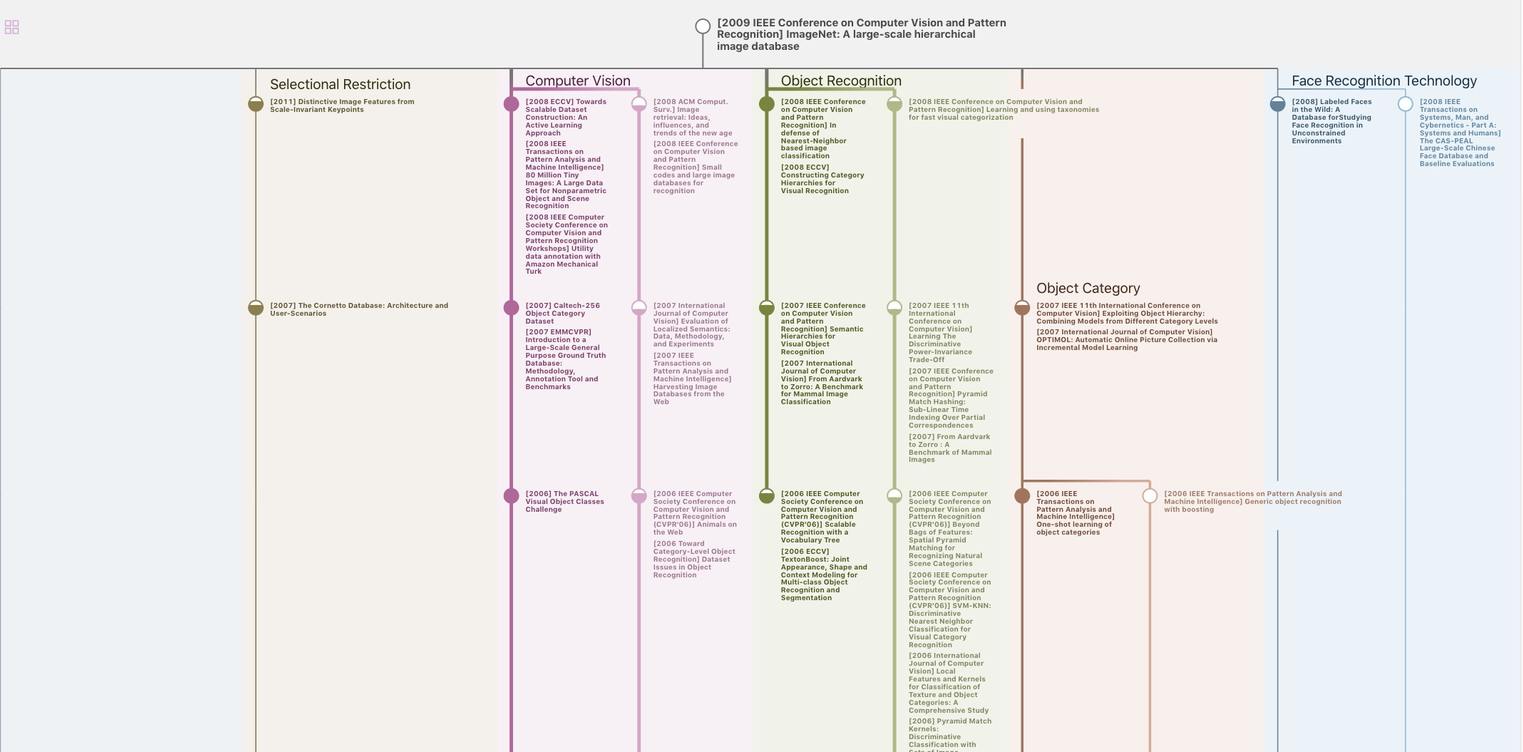
生成溯源树,研究论文发展脉络
Chat Paper
正在生成论文摘要