Lower Bounds for Over-the-Air Statistical Estimation
2021 IEEE International Symposium on Information Theory (ISIT)(2021)
摘要
We study lower bounds for minimax statistical estimation over a Gaussian multiple-access channel (MAC) under squared error loss, using techniques from both statistical estimation and information theory. We characterize these bounds in terms of the number of nodes
$n$
and the dimension of the parameter space
$d$
, showing that the risk must be
$\Omega(d/n\log n)$
. This is within a
$\log n$
factor of previous analog achievability results. While lower bounds for minimax statistical estimation have been previously studied under quantization constraints that abstract the physical layer as noiseless bit pipes, to our knowledge our paper provides the first lower bounds for statistical estimation over noisy multi-user channels. This adds to a body of works showing how analog schemes that consider the physical layer jointly with the estimation scheme, can outperform digital schemes that separate the two with an abstraction layer.
更多查看译文
关键词
Gaussian multiple-access channel,squared error loss,minimax over-the-air statistical estimation scheme,MAC,information theory,quantization constraints,noiseless bit pipe,noisy multiuser channels,abstraction layer
AI 理解论文
溯源树
样例
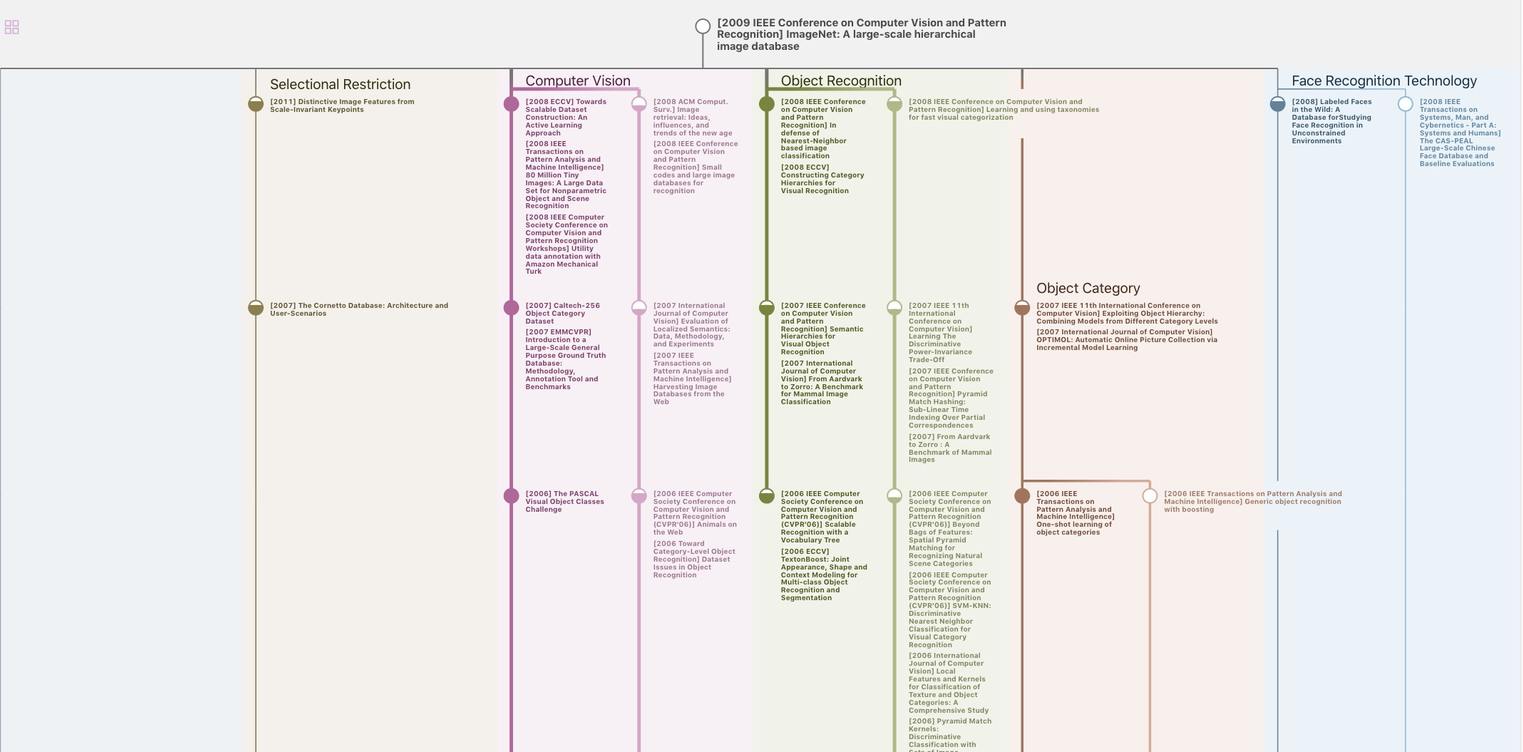
生成溯源树,研究论文发展脉络
Chat Paper
正在生成论文摘要