StyTr2: Image Style Transfer with Transformers
2022 IEEE/CVF Conference on Computer Vision and Pattern Recognition (CVPR)(2022)
摘要
The goal of image style transfer is to render an image with artistic features guided by a style reference while maintaining the original content. Owing to the locality in convolutional neural networks (CNNs), extracting and maintaining the global information of input images is difficult. Therefore, traditional neural style transfer methods face biased content representation. To address this critical issue, we take long-range dependencies of input images into account for image style transfer by proposing a transformer-based approach called StyTr2. In contrast with visual transformers for other vision tasks, StyTr2 contains two different transformer encoders to generate domain-specific sequences for content and style, respectively. Following the encoders, a multi-layer transformer decoder is adopted to stylize the content sequence according to the style sequence. We also analyze the deficiency of existing positional encoding methods and propose the content-aware positional encoding (CAPE), which is scale-invariant and more suitable for image style transfer tasks. Qualitative and quantitative experiments demonstrate the effectiveness of the proposed StyTr2 compared with state-of-the-art CNN-based and flow-based approaches. Code and models are available at https://github.com/diyiiyiii/StyTR-2.
更多查看译文
关键词
Image and video synthesis and generation, Deep learning architectures and techniques
AI 理解论文
溯源树
样例
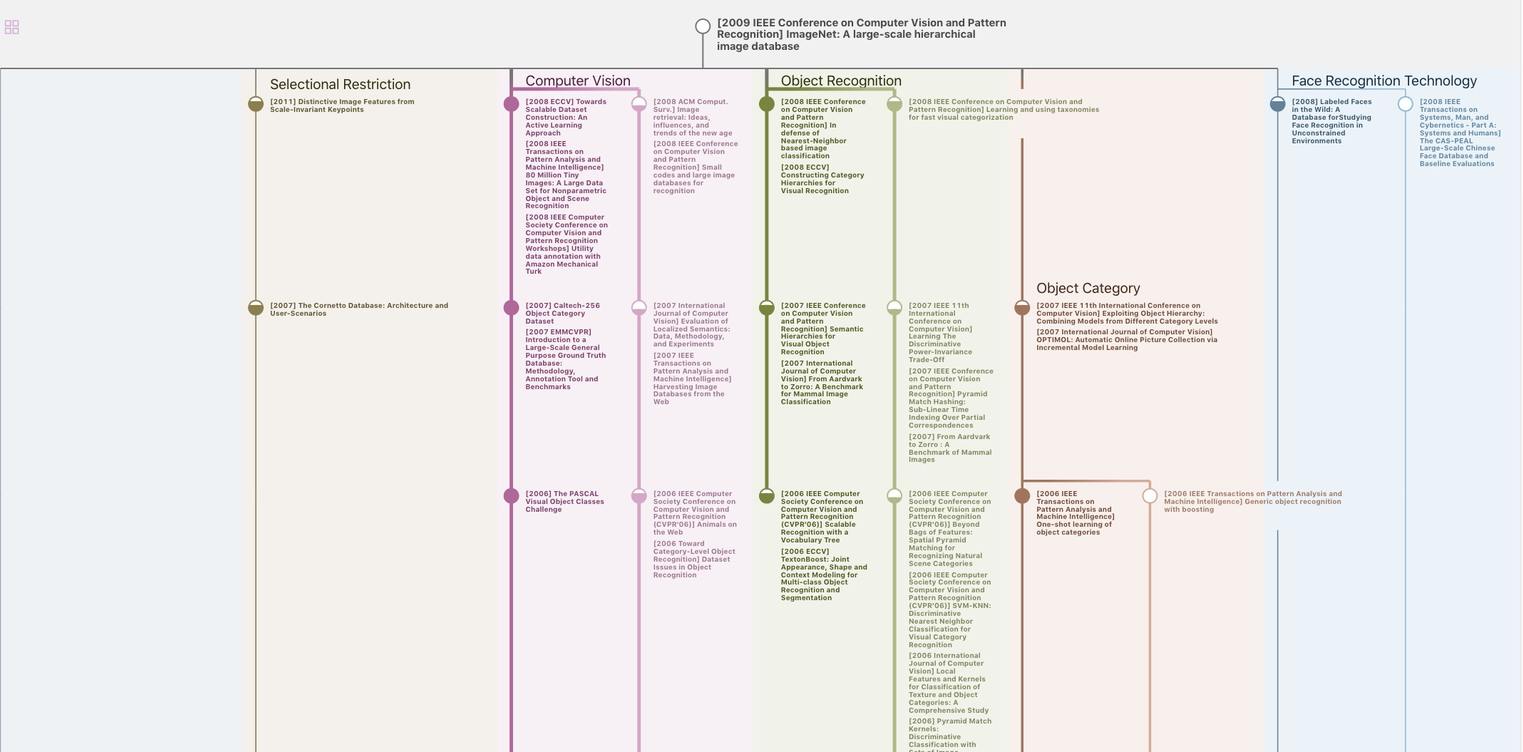
生成溯源树,研究论文发展脉络
Chat Paper
正在生成论文摘要