A Feature Selection Method based on Sparse Regularization Deep Neural Network
2023 4th International Conference on Computer Engineering and Application (ICCEA)(2023)
摘要
Data dimensionality reduction is a technology that converts high-dimensional data into low-dimensional data. With the continuous growth of data dimensions, data dimensionality reduction is essential in both industrial applications and scientific research. Feature selection is an effective method to achieve dimensionality reduction. However, most existing feature selection methods can only be applied to small datasets, and they cannot be used in big datasets or have poor performance. In order to overcome this dilemma, A novel feature selection method based on sparse regularization deep neural network, called Sparse Regularization Deep Feature Selection (SRDFS), is proposed. SRDFS utilizes deep neural network and sparse regularization to realize feature selection, which has a good performance on big datasets. In addition, this paper proposes an optimization algorithm based on soft threshold function for sparse regularization. Finally, we compare the proposed method with the related state-of-the-art feature selection methods on six big datasets, and the experimental results show the effectiveness and superiority of the method.
更多查看译文
关键词
Dimensionality reduction,feature selection,neural network,pattern classification
AI 理解论文
溯源树
样例
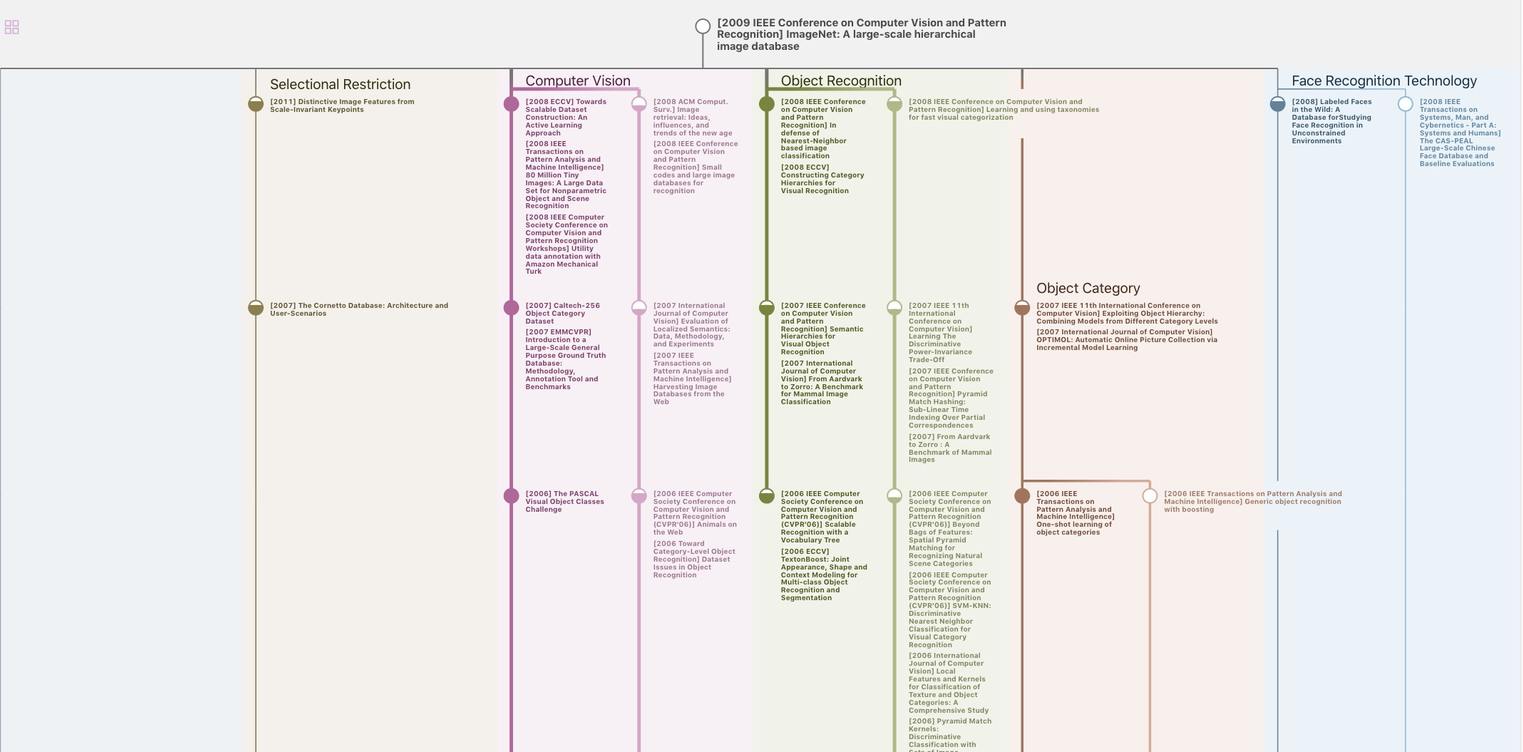
生成溯源树,研究论文发展脉络
Chat Paper
正在生成论文摘要