Departure Scheduling for Multi-airport System using Multi-agent Reinforcement Learning
2023 IEEE/AIAA 42ND DIGITAL AVIONICS SYSTEMS CONFERENCE, DASC(2023)
摘要
The escalation in air traffic demand has precipitated the establishment of the multi-airport systems aimed at effectively handling the influx of flights. Nevertheless, these multi-airport systems encounter airspace resource conflicts during the departure phase, rendering resolution challenging when airports operate on separate schedules. Collaborative departure scheduling has been identified as a promising and effective solution to address these challenges. However, the collaborative departure scheduling presents inherent complexity as an NP-hard problem. In sight of this, this paper proposes a two-step optimization framework to address this typical NP-hard optimization problem. In the first step, the take-off time of each flight is optimized in the first step, so as to minimize flight delays and scheduling chaos. Subsequently, the framework proceeds to optimize the standard instrument departure (SID) based on the optimal take-off time. A multi-agent reinforcement learning (MARL) approach is implemented in both of the two steps to optimize take-off times and select SIDs, respectively. The trained model can quickly collaborate departure scheduling in various situations, reducing flight delays, scheduling chaos, and departure distances compared to solutions generated by First Come First Served (FCFS) principle. The proposed framework and approach are tested on real air traffic data from the Beijing-Tianjin-Hebei region in Northern China. The results show that the MARL-based collaborative departure scheduling has promising applications in multi-airport systems and offers a scalable, adaptive, and efficient solution to this complex problem.
更多查看译文
关键词
Multi-Airport System,Departure Scheduling,Multi-agent Reinforcement Learning
AI 理解论文
溯源树
样例
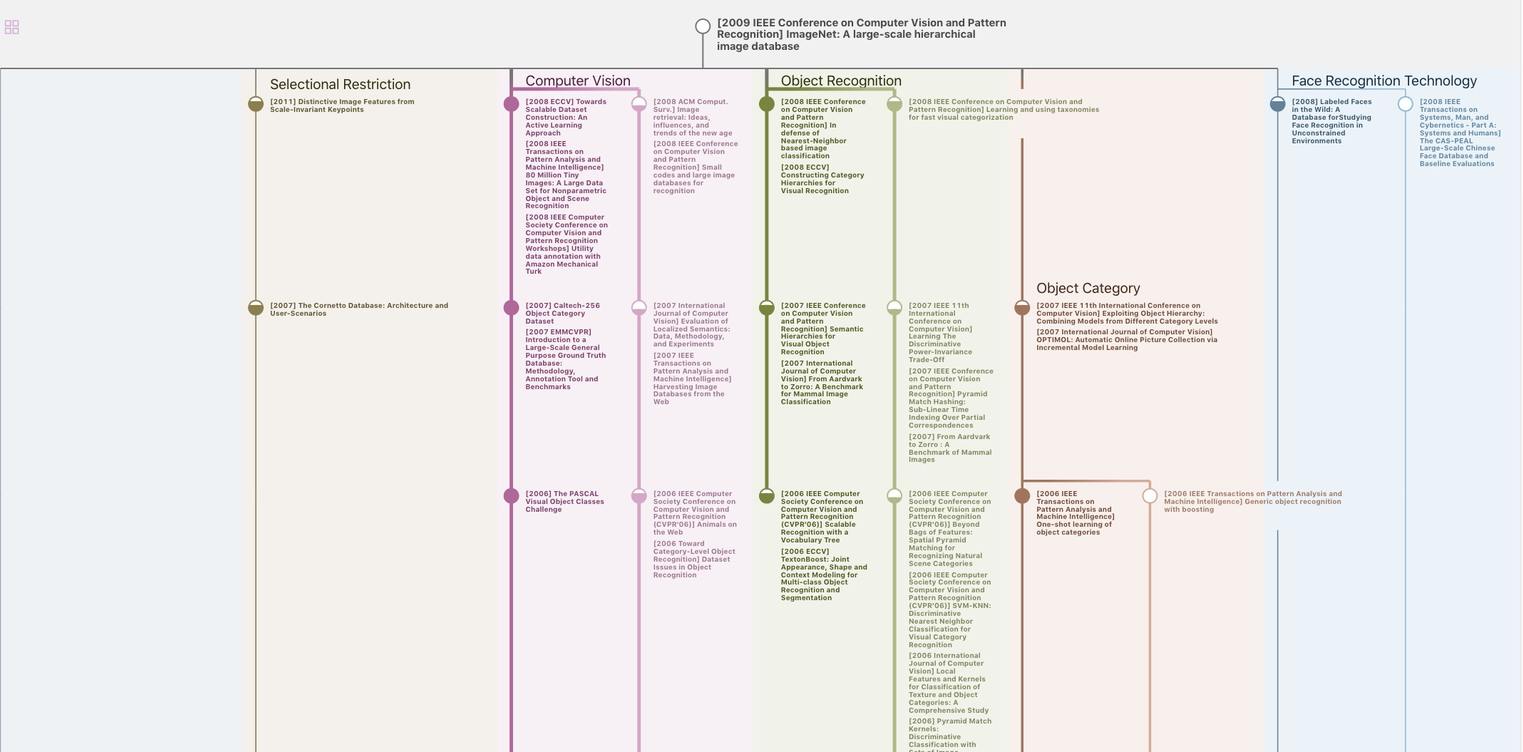
生成溯源树,研究论文发展脉络
Chat Paper
正在生成论文摘要