Blockchain-Enabled Federated Learning with Neuromorphic Edge Devices for Drone Identification and Flight Mode Detection
2023 IEEE/AIAA 42ND DIGITAL AVIONICS SYSTEMS CONFERENCE, DASC(2023)
摘要
Unmanned aerial vehicles (UAVs), also known as drones, are expected to play an integral role in next-generation wireless avionics networks. To help secure these networks from malicious activity, machine learning (ML) based approaches have been proposed to identify drones and their flight modes via direct radio frequency signals. These approaches frequently rely on cloudcentric methods for training, which present serious concerns, such as privacy leakage, resource burden, and undesirable latency. In response to these concerns, a distributed ML paradigm known as federated learning (FL) has been proposed that enables multiple drones to collaboratively train ML models by only exchanging model parameters and not the raw data itself. Unfortunately, the conventional FL framework is strongly dependent on a centralized aggregation server with multiple resource-constrained edge devices, making it susceptible to poisoning attacks and poor network utilization. Recently, it has been found that blockchain technology (BT) holds significant promise for securing and storing data in edge applications with high levels of trust. However, the expanding chain of security blocks consumes a significant amount of computing power, thus limiting its scalability. To bridge the gap between computational efficiency and security, we propose a blockchain-empowered and energy-efficient FL framework with neuromorphic edge devices. To assess the effectiveness of our proposed framework we perform a drone identification and flight mode detection task with a spiking neural network (SNN). Finally, we compare our neuromorphic approach with competitive alternatives to validate the energy and performance gains of our system.
更多查看译文
关键词
spiking neural network,blockchain,federated learning,neuromorphic computing,drone detection,edge computing
AI 理解论文
溯源树
样例
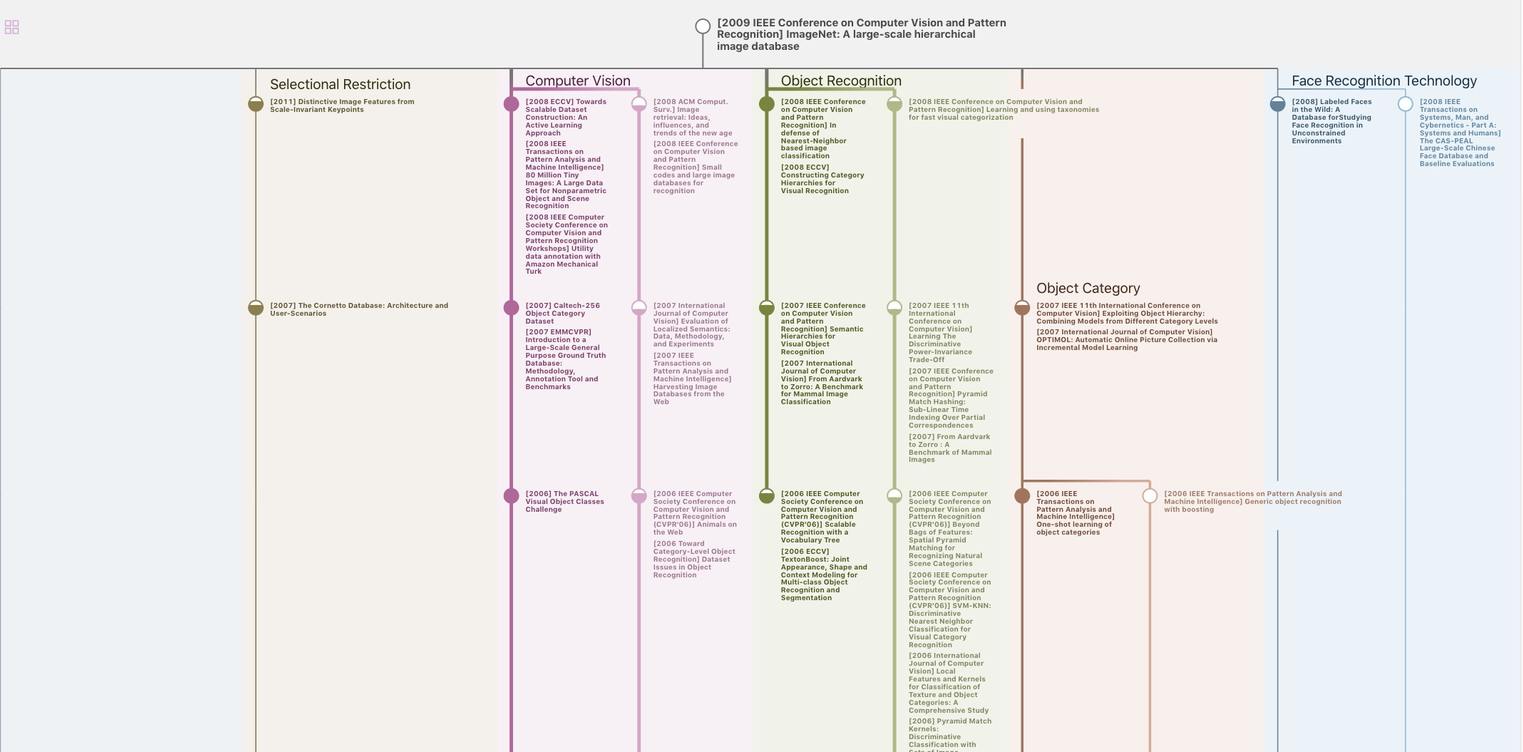
生成溯源树,研究论文发展脉络
Chat Paper
正在生成论文摘要