Application of CohereNet to Photoacoustic Data for Non-Invasive, In Vivo, Subcutaneous Imaging
2023 IEEE International Ultrasonics Symposium (IUS)(2023)
摘要
Short-lag spatial coherence (SLSC) beamforming of photoacoustic signals reduces acoustic clutter and enhances the contrast of underlying signals of interest. However, the original SLSC imaging algorithm is also known to be computationally expensive to implement. CohereNet, a custom deep neural network (DNN), was previously introduced to address the computational complexity of SLSC beamforming when applied to ultrasound data. Although it is conceivable that CohereNet can be translated to photoacoustic data, this translation is challenged by differences in data patterns and imaging depths when compared to ultrasound data, and it requires a similar diversity of photoacoustic training data to that present in ultrasound training data. Therefore, we propose to translate CohereNet to photoacoustic imaging after taking advantage of the diversity of signal-to-noise ratios (SNRs) available with transcutaneous in vivo data containing various levels of acoustic clutter. Raw channel data were acquired from the distal forearm of 18 volunteers with a variety of skin constitutive pigmentation, with 12 volunteers designated for training, 3 for validation, and the remaining 3 for testing. Time-delayed kernels and corresponding spatial coherence functions were computed and utilized as DNN training inputs and outputs, respectively. The correlation between standard central processing unit (CPU) SLSC and DNN SLSC images in the test set were 0.76, 0.78, and 0.84 when acquired with 750 nm, 810 nm, and 870 nm wavelengths, respectively. In addition, a mean SNR improvement of 2.5 dB was achieved with DNN SLSC imaging (relative to CPU SLSC imaging), due to the smoother coherence functions created with CohereNet. Results highlight the versatility of CohereNet, particularly when translating from ultrasound to photoacoustic data after appropriate steps are taken to achieve success, with no evidence of overfitting to training data.
更多查看译文
AI 理解论文
溯源树
样例
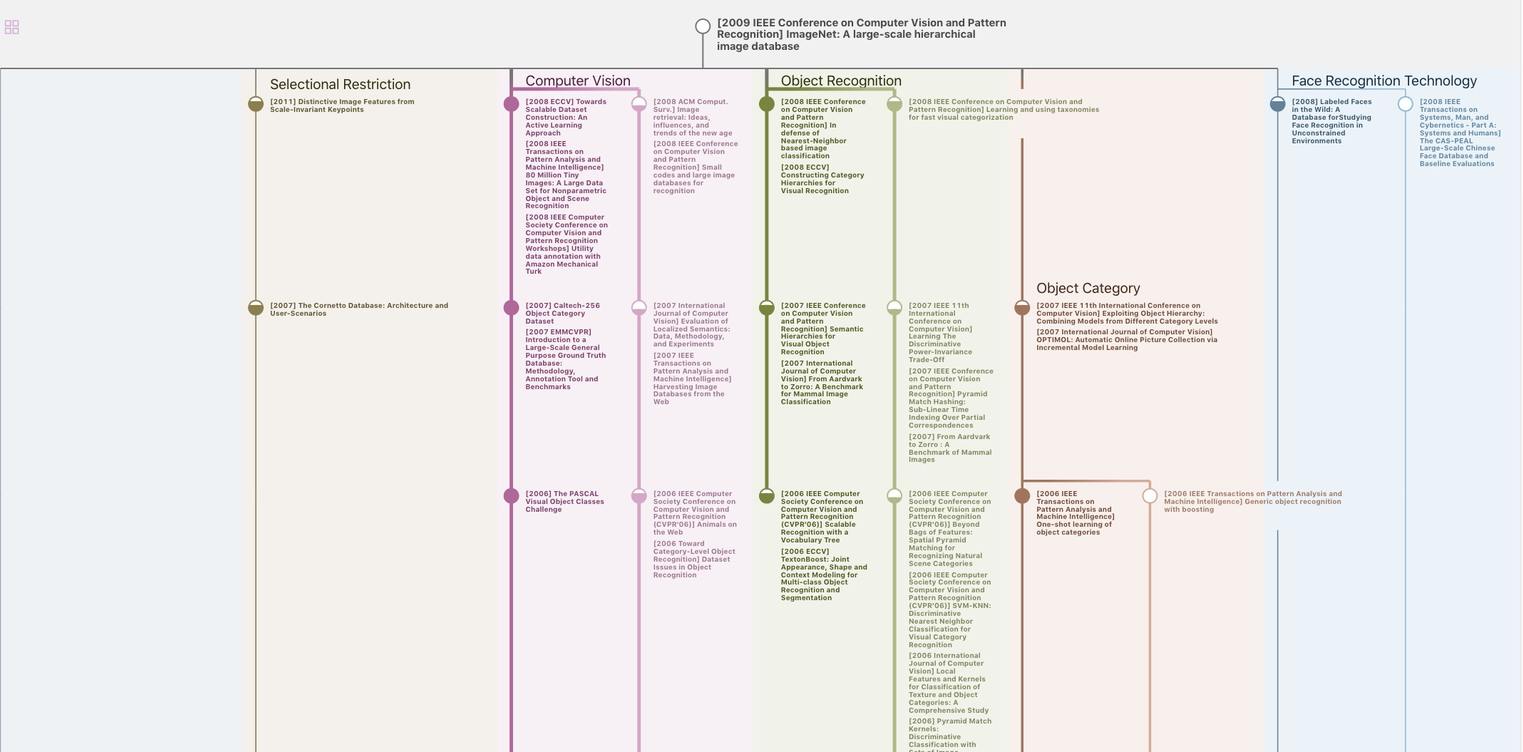
生成溯源树,研究论文发展脉络
Chat Paper
正在生成论文摘要