Adapting a Teachable Robot's Dialog Responses using Reinforcement Learning in Teaching Conversation
2023 32nd IEEE International Conference on Robot and Human Interactive Communication (RO-MAN)(2023)
摘要
Teachable robots can offer benefits to students through the use of social behaviours, such as speech, gaze, and gestures, to promote engagement and learning. Adapting these behaviours can deliver personalised interactions to better suit each individual. There is a growing body of research utilising reinforcement learning in social robotics, however there is limited research in the use of adaptive dialog behaviours for social robots. We propose an adaptive response-selection algorithm for a teachable robot which aims to improve user engagement in the teaching task. The proposed approach uses Q-learning to learn an individualised policy. The algorithm is rewarded according to the time taken per teaching input, and the amount paraphrasing in the user's response. A user study has been conducted to evaluate the algorithm, compared to a method of random response-selection. The results indicate that an adaptive approach learns to select more rewarding actions over time, and personalise to the individual user.
更多查看译文
AI 理解论文
溯源树
样例
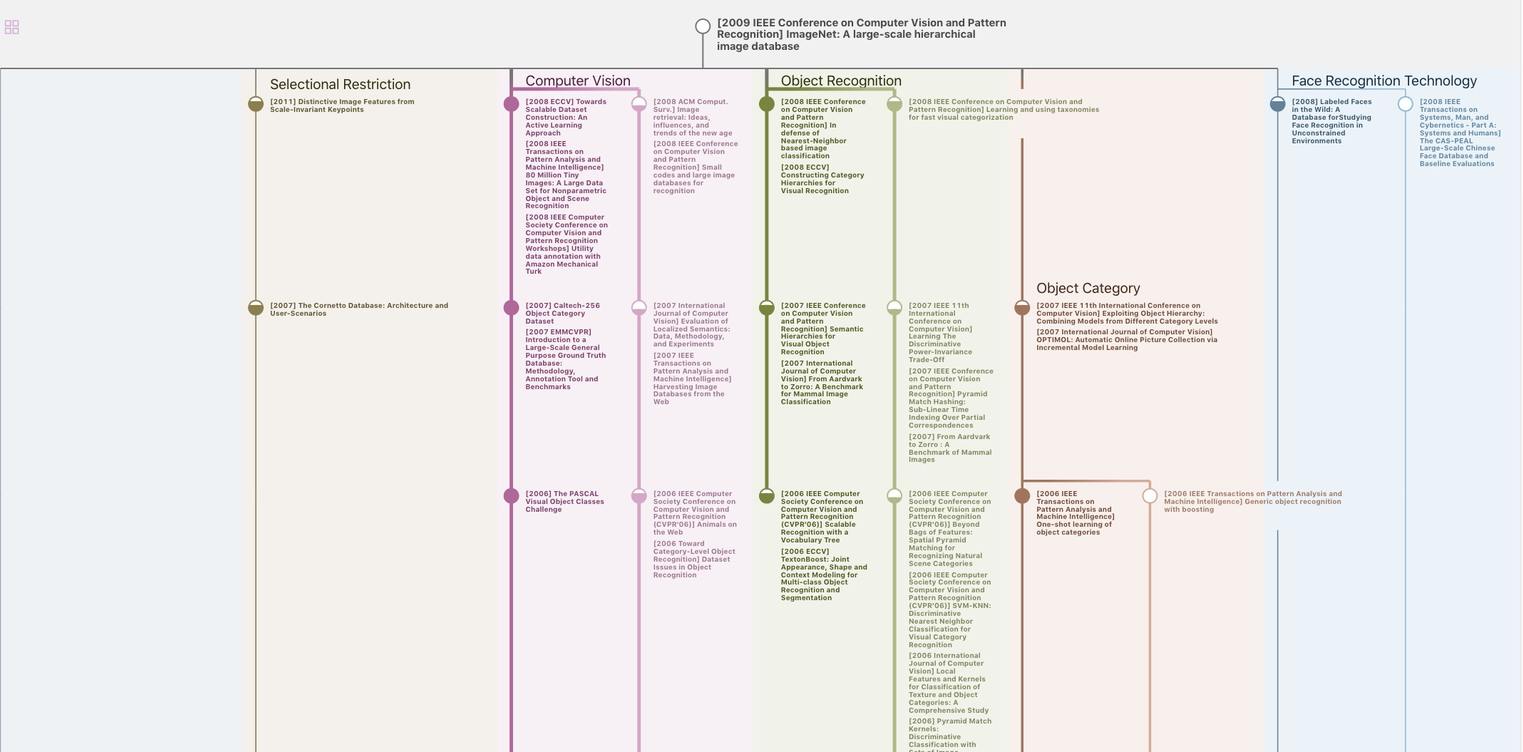
生成溯源树,研究论文发展脉络
Chat Paper
正在生成论文摘要