Skin Lesion Segmentation Based on Modified U-NET Architecture
2023 IEEE International Conference on Advanced Systems and Emergent Technologies (IC_ASET)(2023)
摘要
Skin tumor presents supreme pathologies worldwide. Premature discovery of some diseases is important act to rescue the human life. Through medical inspections, due to the existing similarity between lesions, it is not a simple duty to examine and visualize such lesions. Scientific progresses in the practice of deep learning approaches have employed for identifying skin harms. In recent decades, convolutional neural networks “CNN” has establish encouraging procedures to accomplish current condition of knowledge results in diver's applications of clinical imaging. Yet, owing to the need of understanding of long distance spatial relations situated in used images, CNN competences are deemed limited. For image classification, the lately projected Vision Transformer used a completely self-attention focused architecture that picks up long-term spatial relations to emphasis on the image's appropriate portions. To attain improved performance, current transformer-focused networks necessitate large-scale images. Since medical imaging databases are little, implementing unadulterated transformers to medical image examination is complicated. To expand the restoration of comprehensive skin lesion information, numerous segmentation techniques based on (ISIC 2018) datasets have lately been j oint with CNN methods in the usual image field. This work offers a computerized based on Trans- U-Net model to segment skin lesion, which will help in the skin cancer diagnosis. The results demonstrate that the combined Trans-U -Net surpasses other testing methods with a Recall of 92.34 %, Accuracy of 91.52 %, Precison of 90.54 % and dice coefficient of 90.74 %.
更多查看译文
关键词
Skin Lesion,Trans-U-Net,dermoscopic images,Transformers,Segmentation
AI 理解论文
溯源树
样例
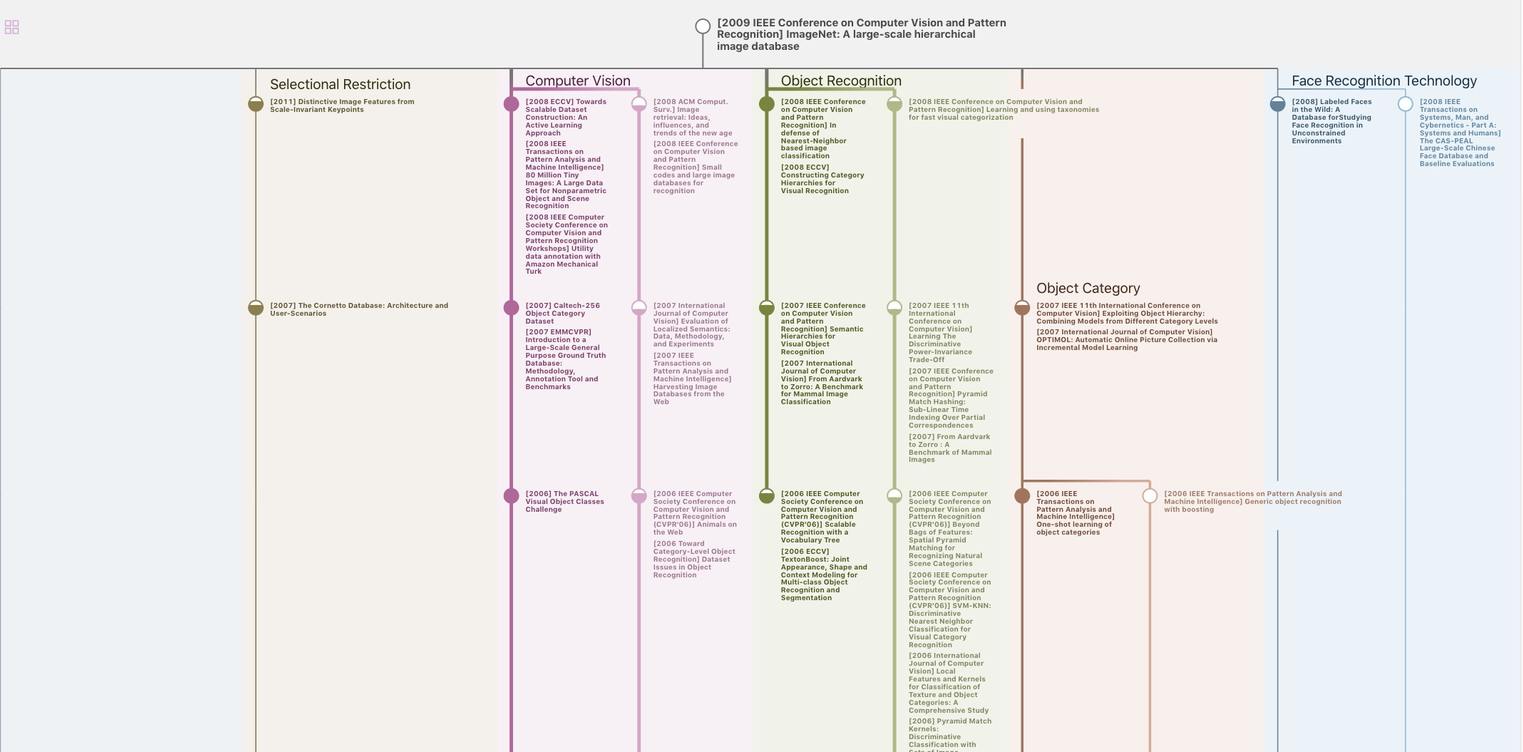
生成溯源树,研究论文发展脉络
Chat Paper
正在生成论文摘要