Tile surface defect detection based on improved YOLOv5
2023 IEEE International Conference on Control, Electronics and Computer Technology (ICCECT)(2023)
摘要
Aiming at the problems of high computational complexity and large number of parameters in traditional ceramic tile defect detection methods, a ceramic tile surface defect detection algorithm based on improved YOLOv5 was proposed. Firstly, the light weight network ShuffleNet was used to reconstruct the Backbone feature extraction network in YOLOv5, and Focus structure was replaced with Conv_BN_ReLU_Maxpool layer. Then, the SE attention mechanism is introduced to improve the feature extraction ability of the model. The improved algorithm mAP reaches 89.4%, the weight file is only 4.76MB, and the number of parameters is 2.64MB, which are reduced by 66% and 63% respectively, and the accuracy rate of defect detection on ceramic tile surface can reach 87.1% or more. Experimental results show that the accuracy is guaranteed and the number of parameters is greatly reduced.
更多查看译文
关键词
defect detection,YOLOv5,ShuffleNet,attentional mechanism
AI 理解论文
溯源树
样例
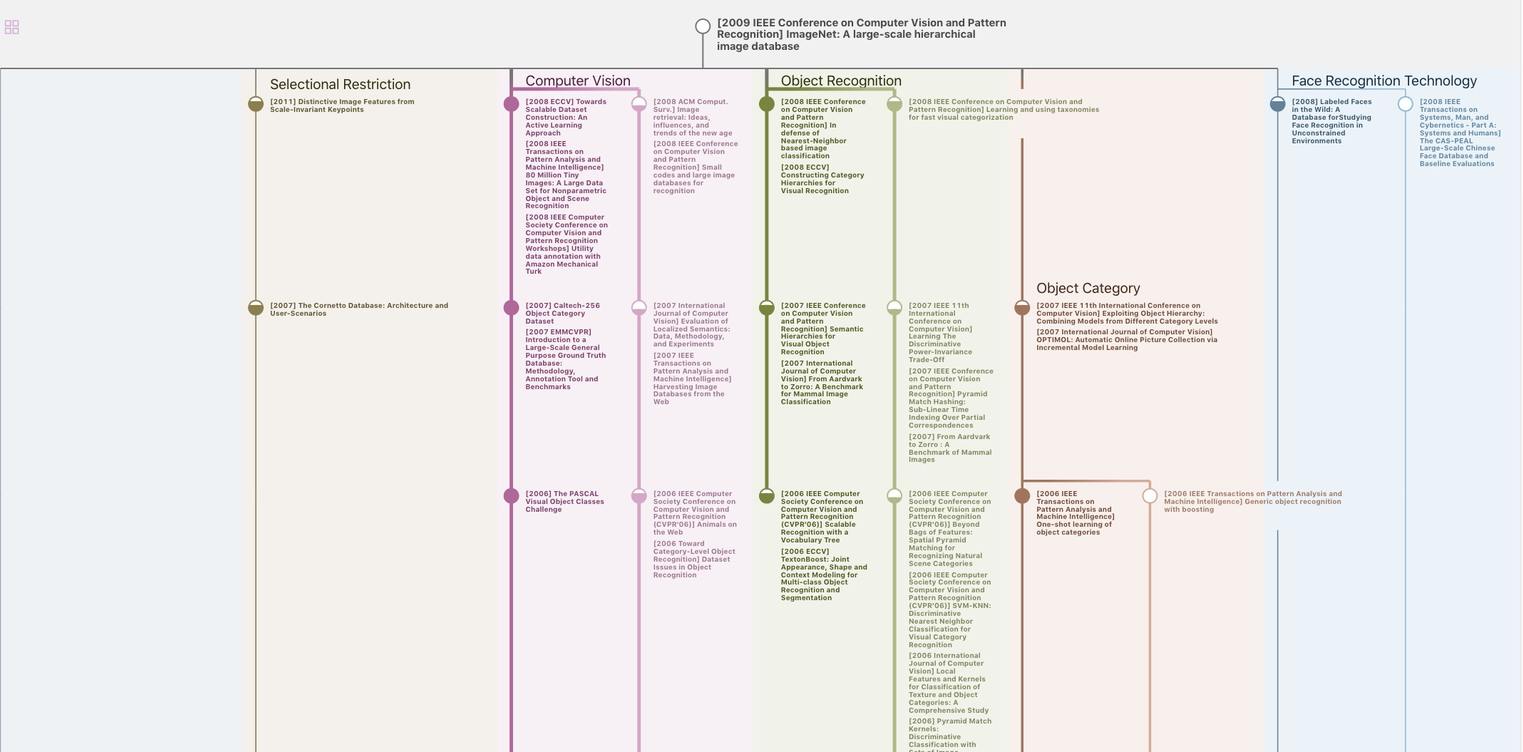
生成溯源树,研究论文发展脉络
Chat Paper
正在生成论文摘要