Improving the Hand Movement Classification Accuracy through EMG based on Histogram Feature Enhancement from Time Domain Representation
2023 Second International Conference on Electrical, Electronics, Information and Communication Technologies (ICEEICT)(2023)
摘要
In order to control a prosthetic hand, electromyography (EMG) signals are essential sources of nervous information. A variety of factors have contributed to changes in the EMG signals' characteristics over time. These factors, such as stress, exhaustion, muscular dystrophy, shifting electrode locations, etc., when using high-density surface electromyography (HD-sEMG) electrodes, reduced the effectiveness of the myoelectric prosthesis and made it uncertain over time, between subjects, as well as during sessions. In this paper, in order to enhance myoelectric prostheses' functionality with regard to the non-stationary properties of EMG signals over time, HD-sEMG electrode technology is combined with histogram features. Ninapro database2 used for classifying the hand movements of five subjects through EMG signals generated from forearm muscle with linear discriminant analysis (LDA) classifier based on the proposed features. The classification performance based upon proposed histogram features achieved classification accuracies of 75.78 % for subject1, 73.53% for subject2, about 93.85% for subject3, 90.1% for subject4 and 86.71% for subject5 respectively. The findings establish that the time domain representation has a smaller impact on classification accuracy than do the histogram features.
更多查看译文
关键词
Classifier,EMG,Histogram,LDA,Time domain
AI 理解论文
溯源树
样例
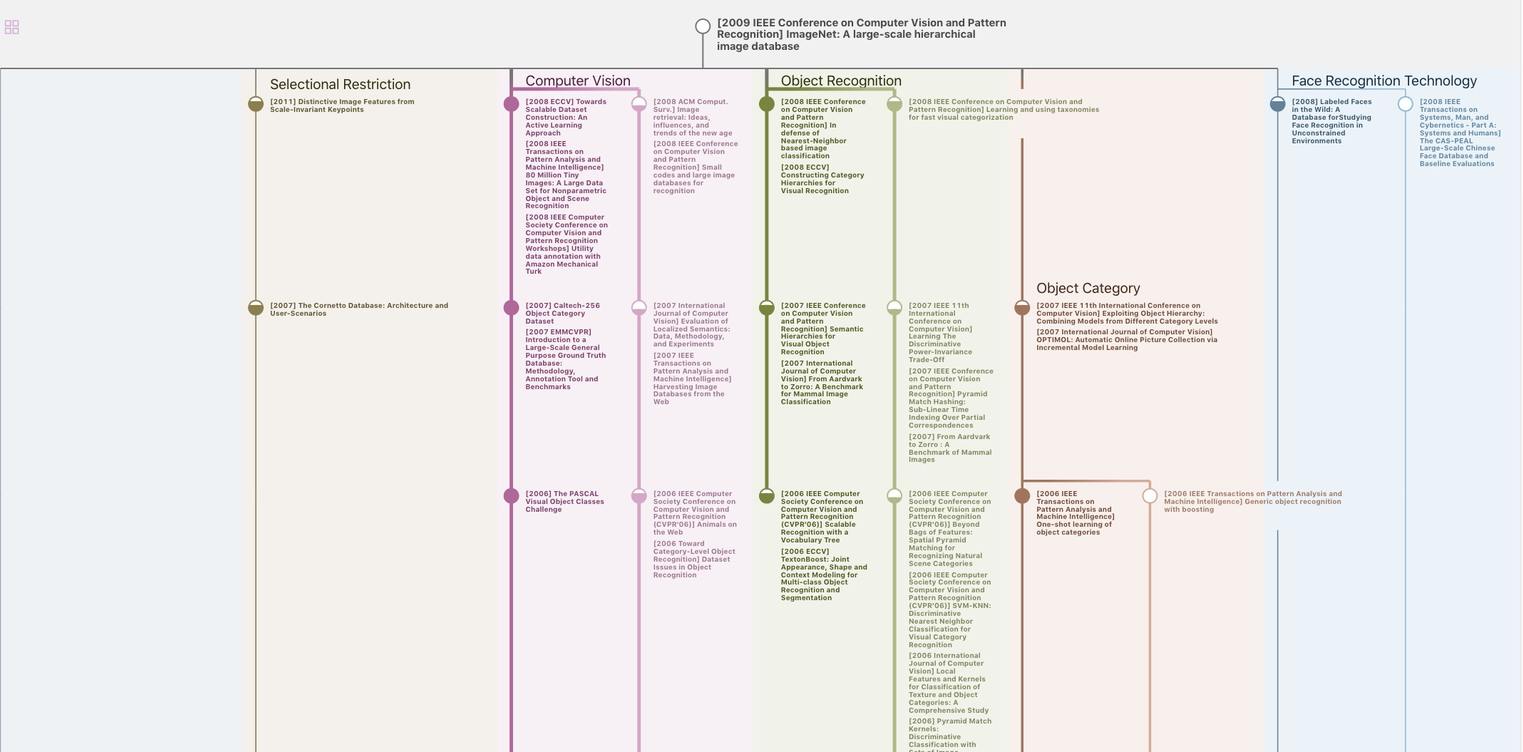
生成溯源树,研究论文发展脉络
Chat Paper
正在生成论文摘要