Development and Field-Testing of a Non-intrusive Classroom Attention Tracking System (NiCATS) for Tracking Student Attention in CS Classrooms
2022 IEEE Frontiers in Education Conference (FIE)(2022)
摘要
This Research to Practice Full Paper presents our Non-intrusive Classroom Attention Tracking System (NiCATS) and discusses the data collected through it. Academic instructors and institutions desire the ability to accurately and autonomously measure students' attentiveness in the classroom. Generally, college departments use unreliable direct communication from students, observational sit-ins, and end-of-semester surveys to collect feedback regarding their courses. Each of these methods of collecting feedback is useful but does not provide automatic feedback regarding the pace and direction of lectures. It has been widely reported that attention levels during passive classroom lectures generally drop after about ten to thirty minutes and can be restored to normal levels with regular breaks, novel activities, mini-lectures, case studies, or videos. Tracking these “drops” in attention can be crucial for the accurate timing of these change-ups in activities. This allows for maximal attention and a greater amount of deeply learned material. Autonomously collected data can also be used either in real-time or post-hoc to alter the design and presentation of lectures. Keeping track of student attention is vital to having confidence in delivering material. Even if lectures do not break up presentation slides with attention-raising activities, they can still show more important information during periods of high attention and less important information during periods of low attention. This area of research has applications both in in-person classrooms and online learning environments. The long-term goals of this research can prove invaluable for large in-person classrooms or classrooms where students’ faces are obscured, such as behind computer monitors.
更多查看译文
关键词
tracking student attention,classroom,field-testing,non-intrusive
AI 理解论文
溯源树
样例
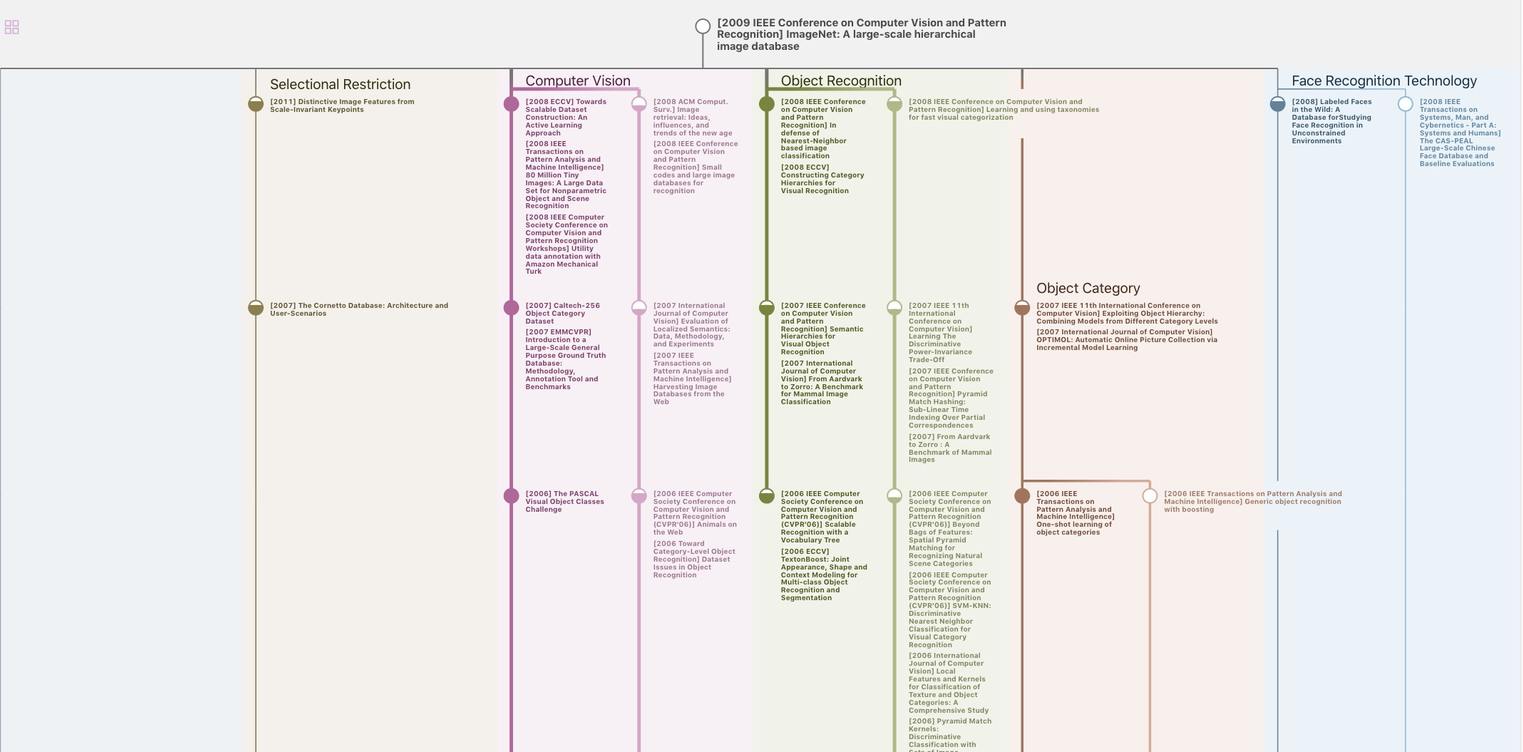
生成溯源树,研究论文发展脉络
Chat Paper
正在生成论文摘要