A Physics-Informed Gaussian Process Regression Algorithm for the Dynamics of the Planar Pendulum
2023 42nd Chinese Control Conference (CCC)(2023)
摘要
Gaussian process regression has received considerable attention due to its performance in solving the problem of learning and predicting the dynamics of certain systems in the machine learning area. However, this data-driven method ignores the prior physical information. A feasible method to tackle this problem is to embed prior dynamics into the Gaussian process regression. This naturally relies on numerical discretizations of continuous-time differential equations that describe the dynamics. However, conventional discretization schemes do not respect the intrinsic geometric structure of the system, which plays an important role when analyzing the properties of the mechanical system. In this work, we develop a physic-informed Gaussian process regression algorithm based on Hamel's formalism and its variational integrator. Computational properties are illustrated by the numerical experiment of learning and predicting the dynamics of a planar pendulum.
更多查看译文
关键词
Gaussian process regression,Hamel's formalism,physics-informed learning,variational integrator
AI 理解论文
溯源树
样例
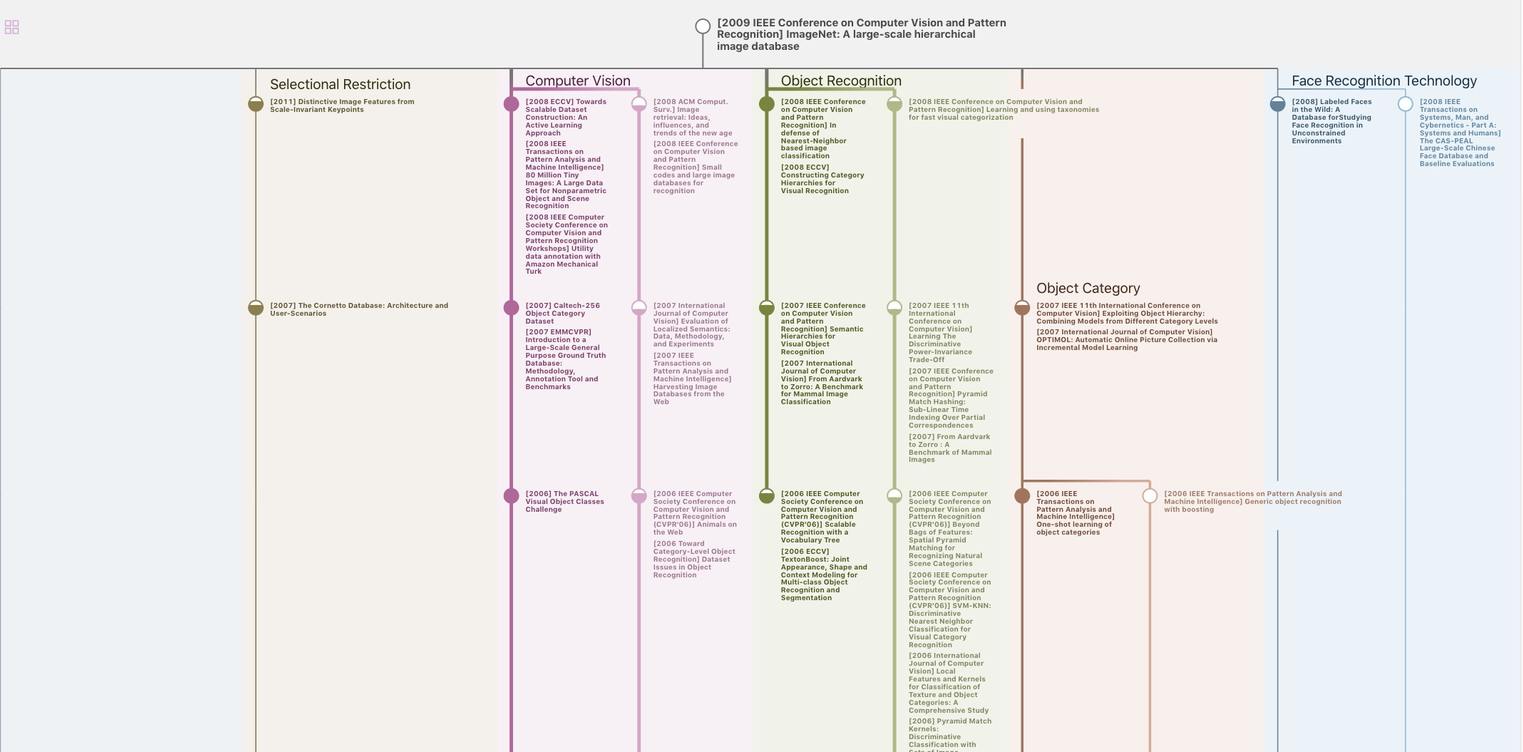
生成溯源树,研究论文发展脉络
Chat Paper
正在生成论文摘要