Autonomous Navigation of QUAVs Under 3D Environments Based on Hierarchical Reinforcement Learning
2023 42nd Chinese Control Conference (CCC)(2023)
摘要
An RL-based hierarchical architecture for QUAV autonomous navigation under 3D environments is presented in this paper. The model consists of three attitude controllers, three position controllers, and one path planner, which are trained separately under the small angle restriction. After training, the QUAV can autonomously reach the target in an end-to-end fashion. To address data imbalance, we use a technique called state saturation, clipping the state if the target is far away from the vehicle. Experiments show that with a training time of 191.6 minutes, the model successfully navigates the QUAV to the reference position without colliding with any obstacles. Moreover, saturation proves an effective way to increase path quality and reduce training time.
更多查看译文
关键词
Quadrotor Unmanned Aerial Vehicle (QUAV),Autonomous Navigation,Hierarchical Reinforcement Learning
AI 理解论文
溯源树
样例
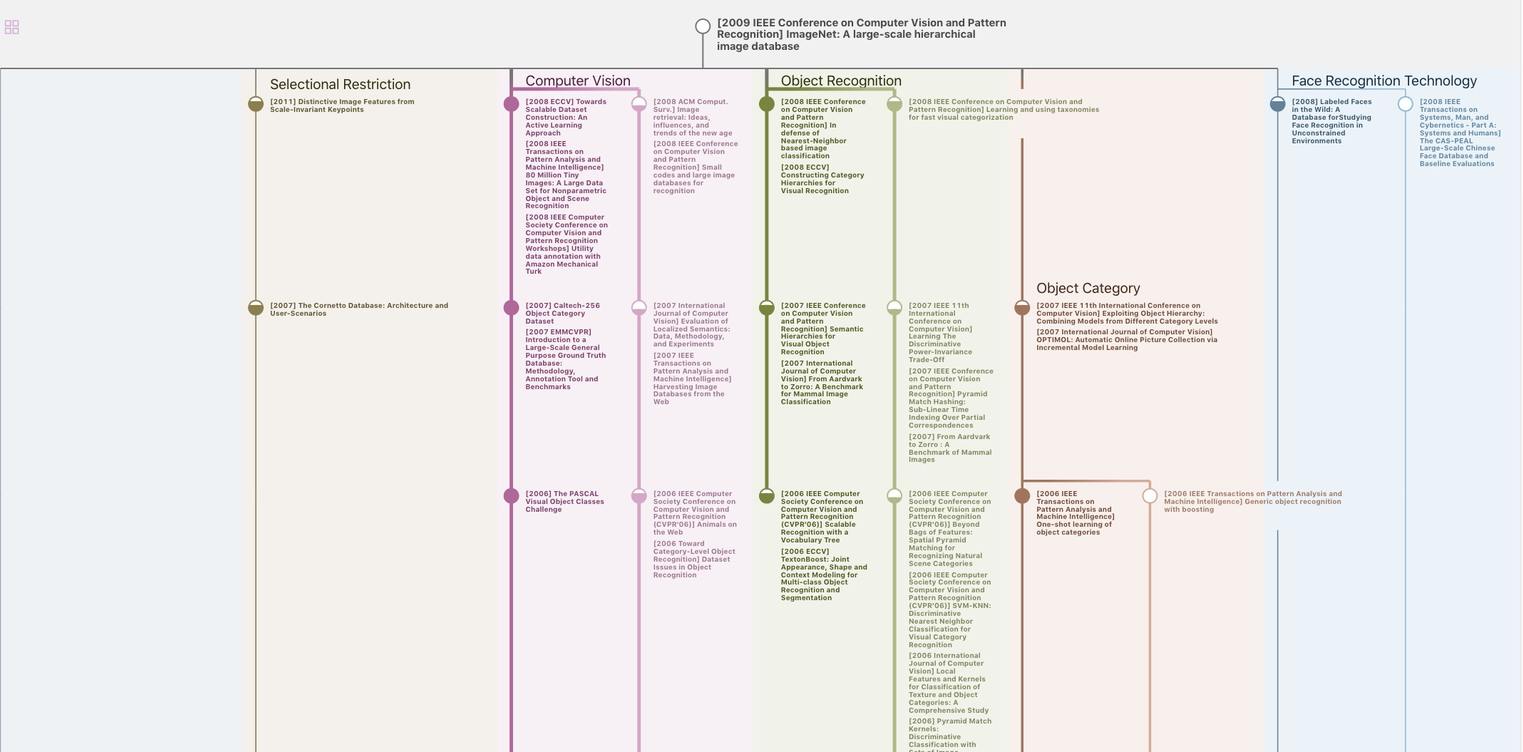
生成溯源树,研究论文发展脉络
Chat Paper
正在生成论文摘要