A Proximal Gradient Method for Regularized Deep Neural Networks
2023 42nd Chinese Control Conference (CCC)(2023)
摘要
In this work, a regularized minimization problem is proposed for training the DNNs with a cross entropy loss function. We adopte an hnorm penalty to it when the activation functions are convex and non-smooth. We prove that the optimization problem can act as an exact penalty model whose global minimizers are also solutions of the original regularized model. Due to the convexity and boundedness of the feasible set of the optimization problem, a proximal gradient algorithm is adopted to solve it. Specifically, a smoothing technique is applied to the activation function when it is non-smooth, e.g., ReLU and leaky ReLU. Compared with the existing widely used SGD methods, its efficiency and robustness is illustrated through comprehensive numerical experiments.
更多查看译文
关键词
Deep neural network,cross entropy,penalty method,smoothing approximation,proximal gradient descent
AI 理解论文
溯源树
样例
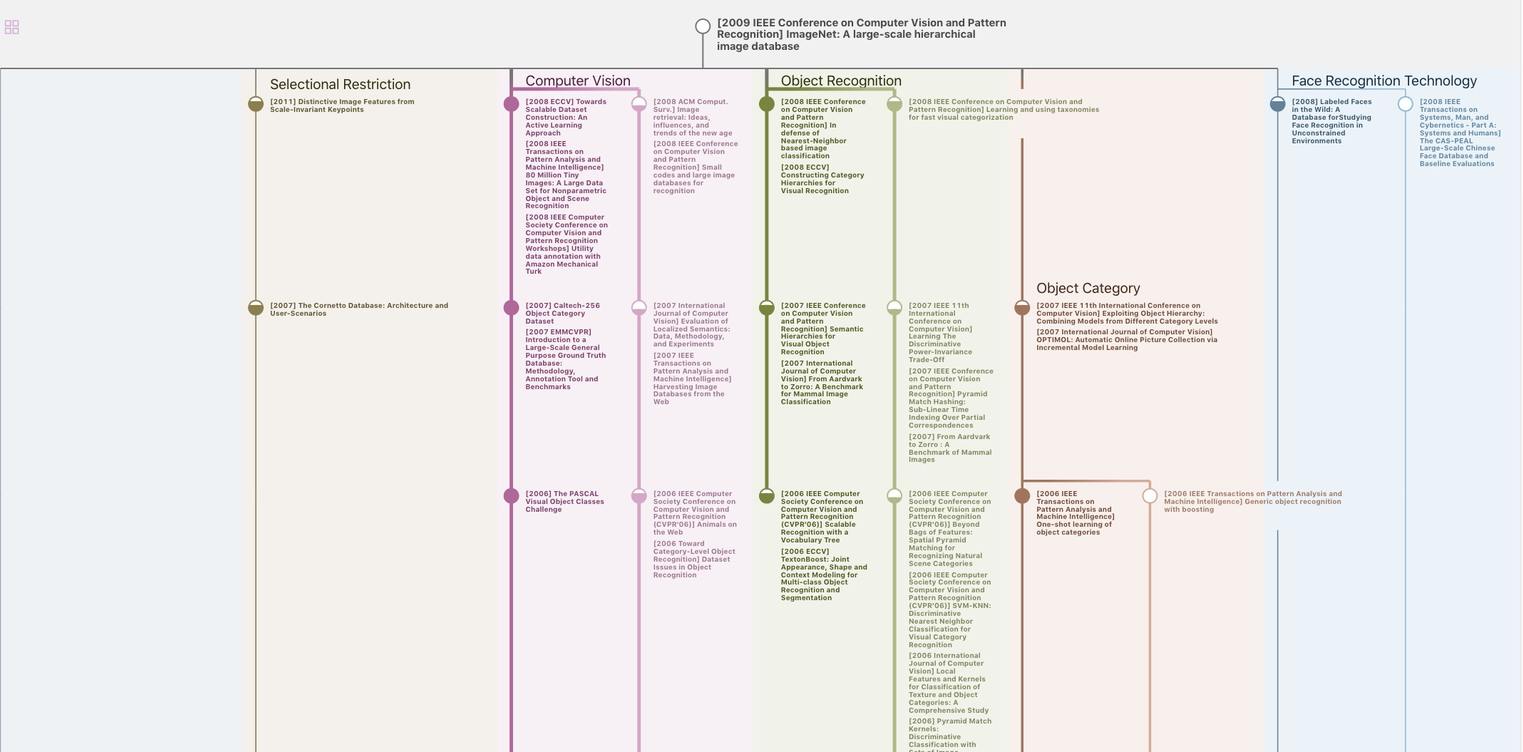
生成溯源树,研究论文发展脉络
Chat Paper
正在生成论文摘要