YOLOv5-CPP: Improved YOLOv5-Based Defect Detection for Photovoltaic Panels
2023 42nd Chinese Control Conference (CCC)(2023)
摘要
The condition monitoring and fault diagnosis of PV modules is the key to ensure the efficient and reliable operation of large PV power plants. Restricted by the inspection efficiency of power plants and the height of IR images taken by UAVs, defective faults often appear in the form of small targets, and in addition, it is difficult for the vision system to quickly locate defective locations due to the problems of complex background, low signal-to-noise ratio and no obvious texture features of defects in IR images. To solve the above two problems, we propose YOLOv5-CPP, which replaces the PANet neck network with CPP-Neck based on YOLOv5. CPP-Neck consists of CBPN and PA structures to enhance the fusion of backbone network feature information while enhancing the localization accuracy of defect bounding boxes in complex backgrounds. Experiments show that compared with the original YOLOv5, the algorithm proposed in this paper obtains a 6.54% improvement in
$\text{mAP}50$
with a lower number of parameters, which exceeds the effect of other target detection models and provides a new idea for a high-efficiency automated visual defect detection system for large PV plants.
更多查看译文
关键词
YOLOv5,deep learning,defect detection,infrared image,photovoltaic panels
AI 理解论文
溯源树
样例
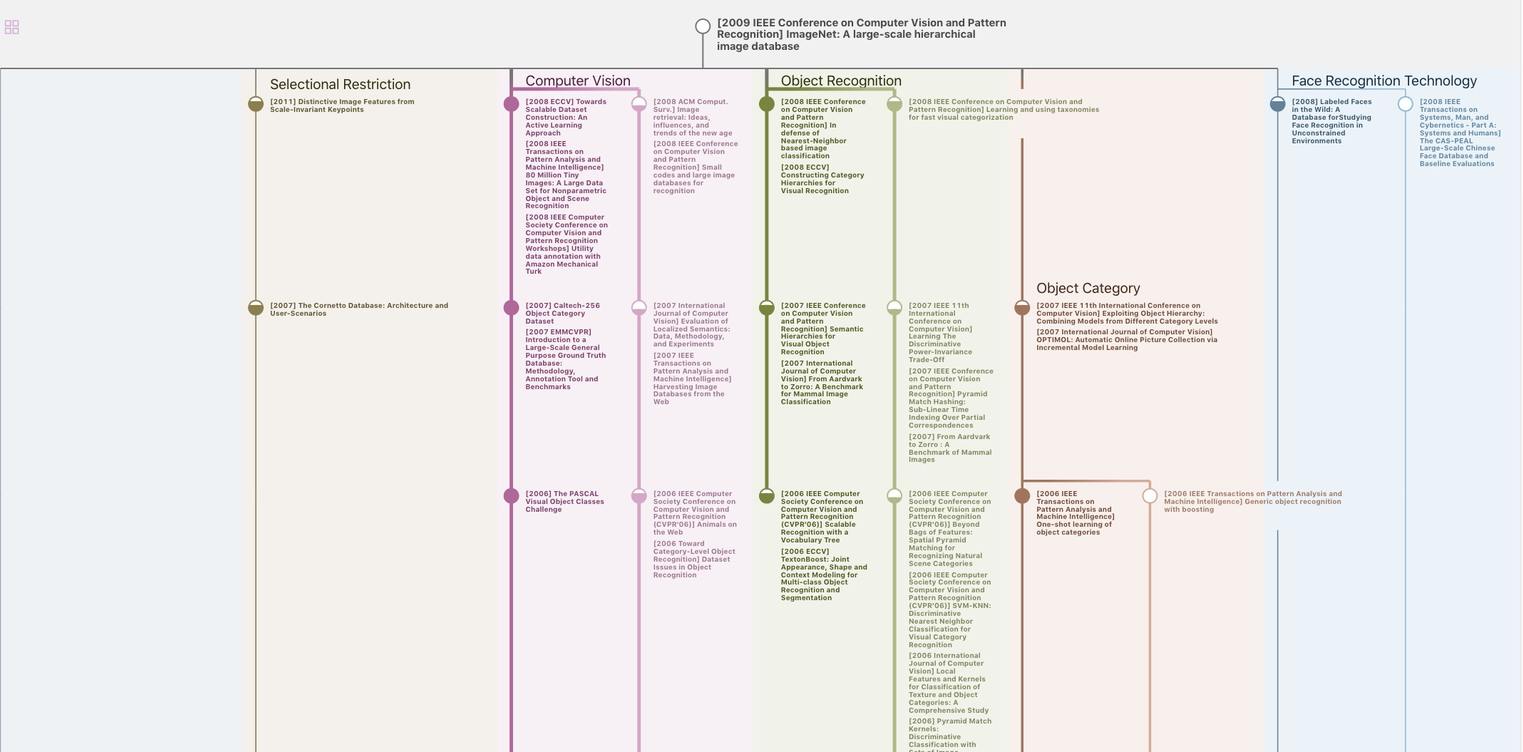
生成溯源树,研究论文发展脉络
Chat Paper
正在生成论文摘要