Focusnet++: Attentive Aggregated Transformations For Efficient And Accurate Medical Image Segmentation
2021 IEEE 18th International Symposium on Biomedical Imaging (ISBI)(2021)
摘要
We propose a new residual block for convolutional neural networks and demonstrate its state-of-the-art performance in medical image segmentation. We combine attention mechanisms with group convolutions to create our group attention mechanism, which forms the fundamental building block of our network, FocusNet++. We employ a hybrid loss based on balanced cross entropy, Tversky loss and the adaptive logarithmic loss to enhance the performance along with fast convergence. Our results show that FocusNet++ achieves state-of-the-art results across various benchmark metrics for the ISIC 2018 melanoma segmentation and the cell nuclei segmentation datasets with fewer parameters and FLOPs.
更多查看译文
关键词
Group Attention,Medical Image Segmentation,Residual Learning
AI 理解论文
溯源树
样例
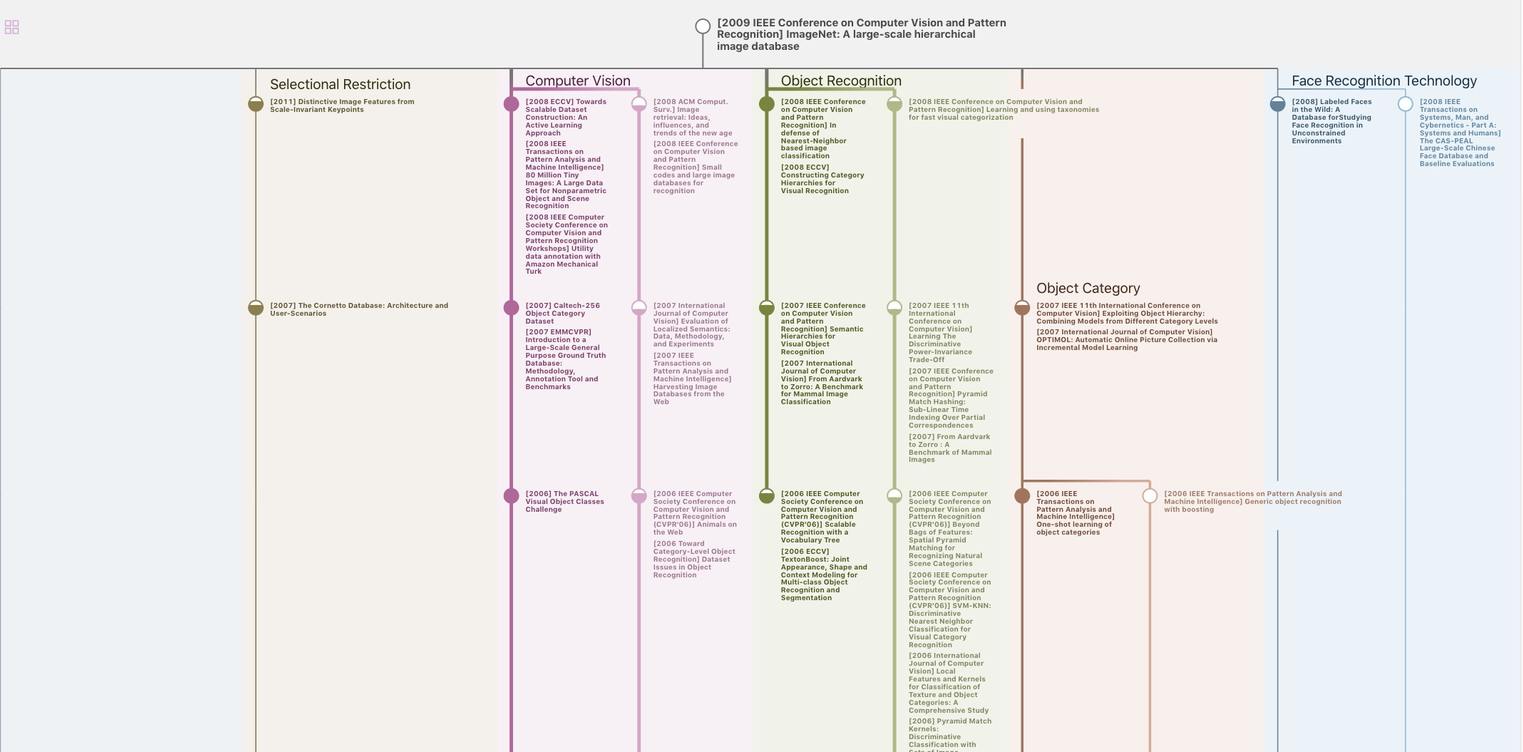
生成溯源树,研究论文发展脉络
Chat Paper
正在生成论文摘要