Semantic Segmentation of Remote Sensing Images with Multi-scale Features and Attention Mechanism
2023 IEEE 18th Conference on Industrial Electronics and Applications (ICIEA)(2023)
摘要
The performance of existing semantic segmentation algorithms for optical remote sensing images is often affected by the issues of real-world datasets, such as the complexity of ground object categories, the dimensional changes between different classes, and the unbalanced proportion of foreground and background. To address this problem, DRCNet, a semantic segmentation model based on attention mechanism and multi-scale fusion is proposed in this paper. The DRCNet is built upon the DeepLab v3+, which introduces a dual attention module to enhance useful features, thereby alleviating the imbalance of foreground-background labels in the datasets. Meanwhile, a residual dense atrous spatial pyramid pooling model based on residual connection is designed to address the checkerboard effect caused by continuous stacking of dilated convolutions. Furthermore, the accuracy of segmentation for different-sized targets is also improved through the cross-layer interaction of multi-scale feature maps. Extensive experimental results demonstrate that the proposed DRCNet achieves an MIoU of 70.176% and a MPA of 88.203% on the Postdam dataset, which represents improvements of 1.676% and 0.339% compared to the DeepLab v3+ model, respectively.
更多查看译文
关键词
Semantic segmentation,Attention mechanism,Multi-scale fusion
AI 理解论文
溯源树
样例
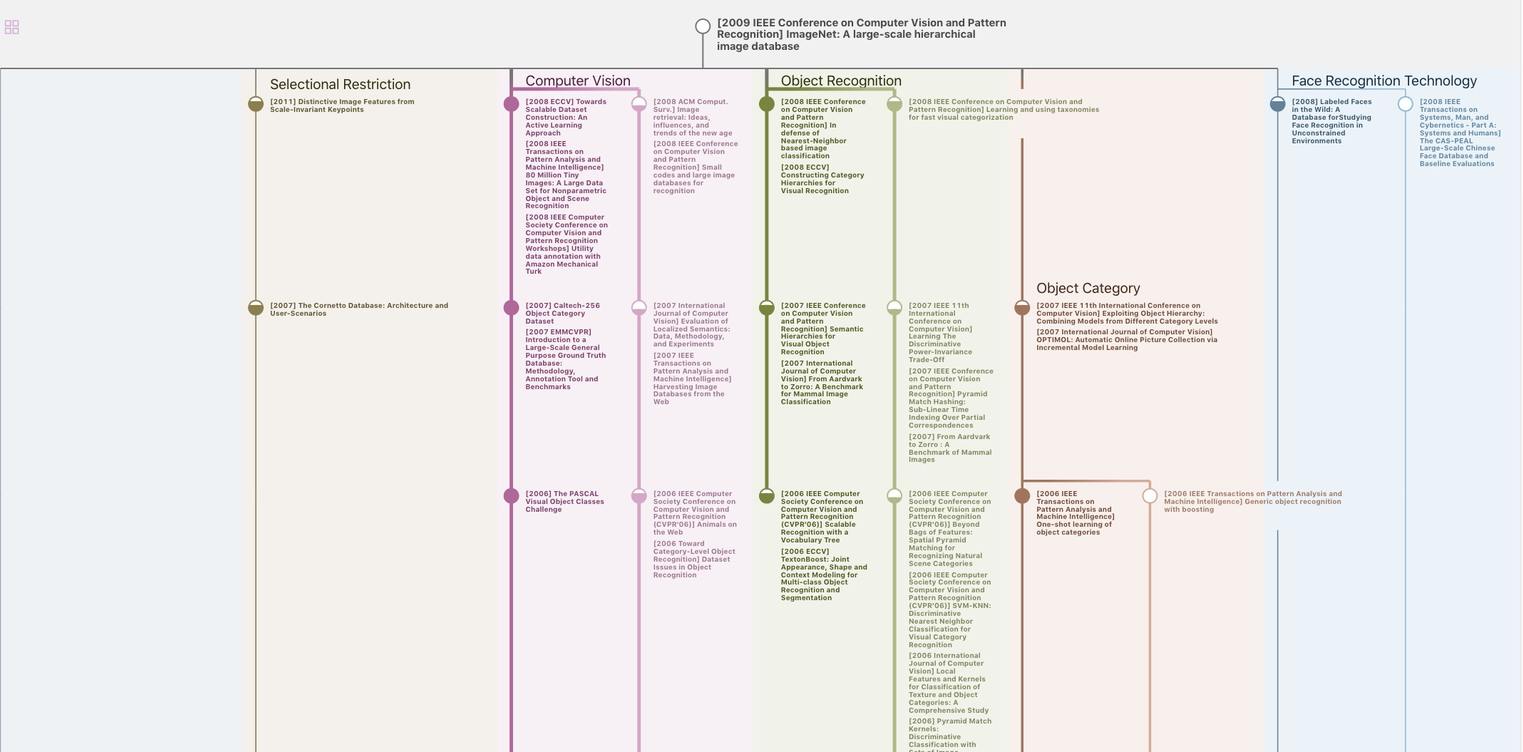
生成溯源树,研究论文发展脉络
Chat Paper
正在生成论文摘要