Data-driven optimization of FePt heat-assisted magnetic recording media accelerated by deep learning TEM image segmentation
2023 IEEE International Magnetic Conference - Short Papers (INTERMAG Short Papers)(2023)
摘要
Areal density increase of heat-assisted magnetic recording (HAMR) media to 4 Tbit/in
2
requires further optimization of microstructure and magnetic properties of FePt-X granular media. This has been hindered due to the large space of processing parameters and selection of optimum segregant. In this study, we demonstrate how data-driven approach can accelerate development of media with desired microstructure and magnetic properties. This approach consists of automatic harvesting of microstructural data from transmission electron microscopy images, and training regressor models capable of predicting optimum processing conditions leading to the desired combination of microstructure and magnetic properties in the FePt granular media. The feasibility of this routine is demonstrated on a series of FePt-C media samples and optimized processing conditions proposed by machine learning were verified experimentally. Such a data-driven workflow offers a promising way to realize 4 Tbit/in
2
areal density in hard disk drives using HAMR.
更多查看译文
关键词
deep learning,heat - assisted magnetic recording,image segmentation,machine learning
AI 理解论文
溯源树
样例
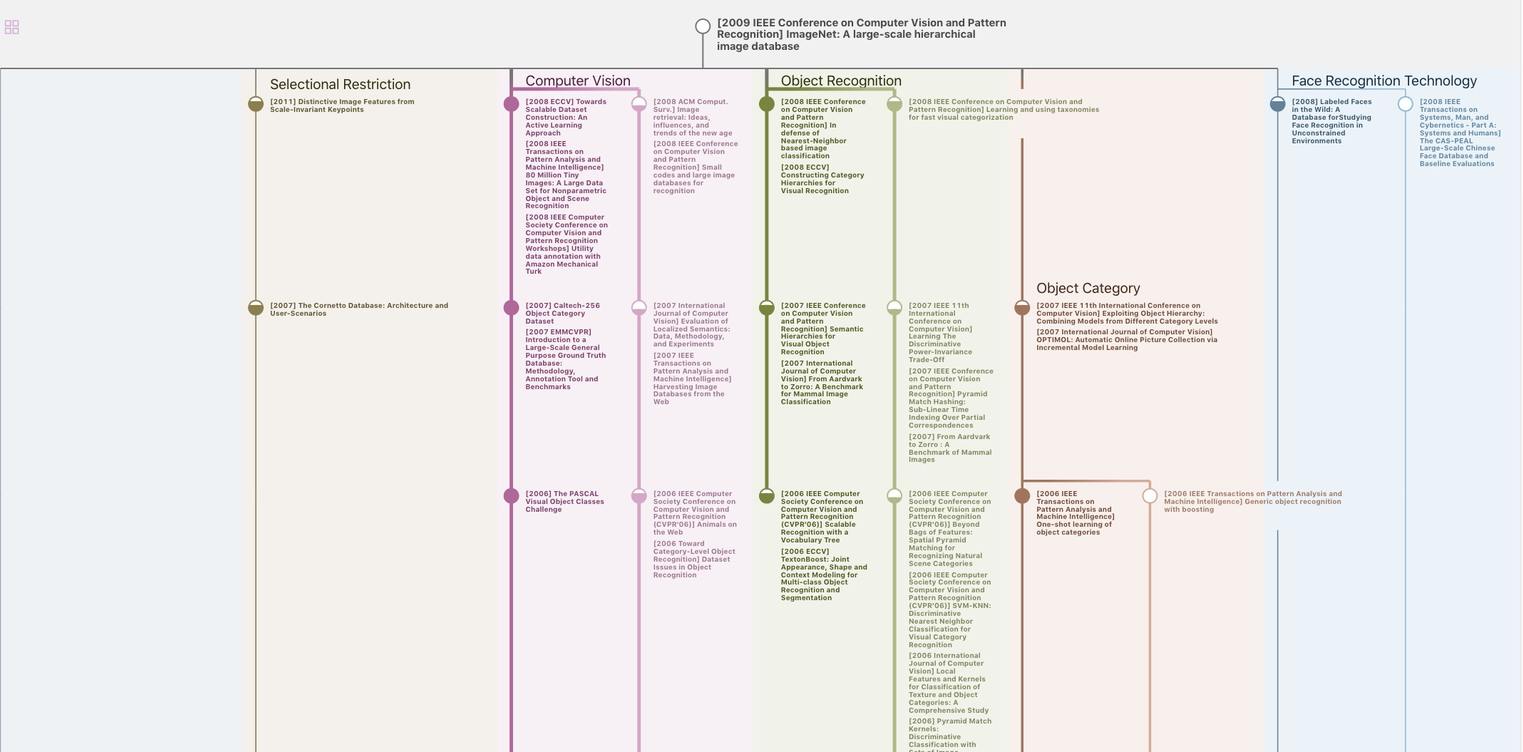
生成溯源树,研究论文发展脉络
Chat Paper
正在生成论文摘要