Growing Like a Tree: Finding Trunks From Graph Skeleton Trees
IEEE TRANSACTIONS ON PATTERN ANALYSIS AND MACHINE INTELLIGENCE(2024)
摘要
The message-passing paradigm has served as the foundation of graph neural networks (GNNs) for years, making them achieve great success in a wide range of applications. Despite its elegance, this paradigm presents several unexpected challenges for graph-level tasks, such as the long-range problem, information bottleneck, over-squashing phenomenon, and limited expressivity. In this study, we aim to overcome these major challenges and break the conventional "node- and edge-centric" mindset in graph-level tasks. To this end, we provide an in-depth theoretical analysis of the causes of the information bottleneck from the perspective of information influence. Building on the theoretical results, we offer unique insights to break this bottleneck and suggest extracting a skeleton tree from the original graph, followed by propagating information in a distinctive manner on this tree. Drawing inspiration from natural trees, we further propose to find trunks from graph skeleton trees to create powerful graph representations and develop the corresponding framework for graph-level tasks. Extensive experiments on multiple real-world datasets demonstrate the superiority of our model. Comprehensive experimental analyses further highlight its capability of capturing long-range dependencies and alleviating the over-squashing problem, thereby providing novel insights into graph-level tasks.
更多查看译文
关键词
Graph neural networks,graph-level tasks,long-range patterns,over-squashing,skeleton trees,trunks
AI 理解论文
溯源树
样例
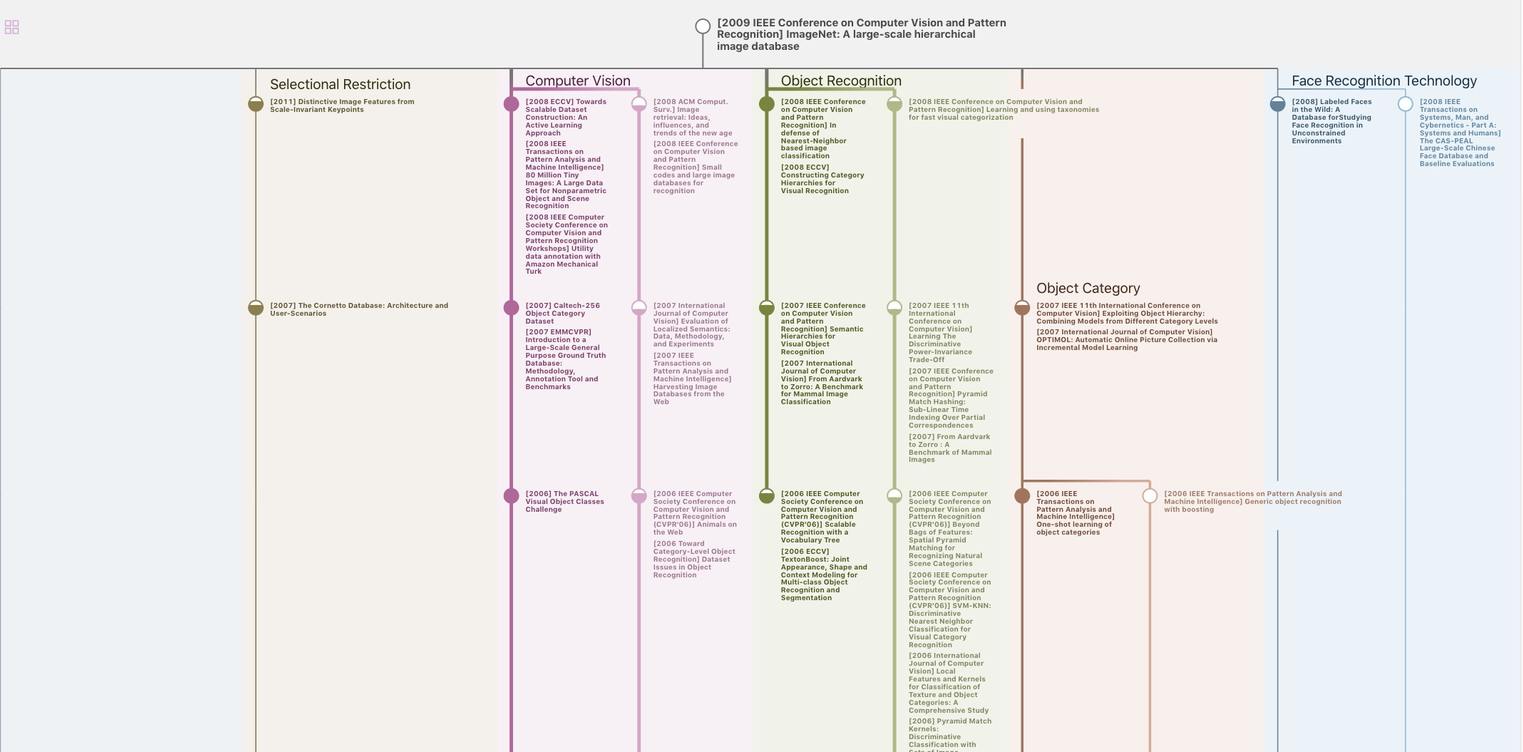
生成溯源树,研究论文发展脉络
Chat Paper
正在生成论文摘要