Multicenter evaluation of the Vetscan Imagyst system using Ocus 40 and EasyScan One scanners to detect gastrointestinal parasites in feces of dogs and cats
JOURNAL OF VETERINARY DIAGNOSTIC INVESTIGATION(2024)
摘要
The Vetscan Imagyst system (Zoetis) is a novel, artificial intelligence-driven detection tool that can assist veterinarians in the identification of enteric parasites in dogs and cats. This system consists of a sample preparation device, an automated digital microscope scanner, and a deep-learning algorithm. The EasyScan One scanner (Motic) has had good diagnostic performance compared with manual examinations by experts; however, there are drawbacks when used in veterinary practices in which space for equipment is often limited. To improve the usability of this system, we evaluated an additional scanner, the Ocus 40 (Grundium). Our objectives were to 1) qualitatively evaluate the performance of the Vetscan Imagyst system with the Ocus 40 scanner for identifying Ancylostoma, Toxocara, and Trichuris eggs, Cystoisospora oocysts, and Giardia cysts in canine and feline fecal samples, and 2) expand the assessment of the performance of the Vetscan Imagyst system paired with either the Ocus 40 or EasyScan One scanner to include a larger dataset of 2,191 fecal samples obtained from 4 geographic regions of the United States. When tested with 852 canine and feline fecal samples collected from different geographic regions, the performance of the Vetscan Imagyst system combined with the Ocus 40 scanner was correlated closely with manual evaluations by experts. Sensitivities were 80.0-97.0% and specificities were 93.7-100.0% across the targeted parasites. When tested with 1,339 fecal samples, the Vetscan Imagyst system paired with the EasyScan One scanner successfully identified the targeted parasite stages; sensitivities were 73.6-96.4% and specificities were 79.7-100.0%.
更多查看译文
关键词
artificial intelligence,cats,detection,dogs,intestinal parasites,parasitology
AI 理解论文
溯源树
样例
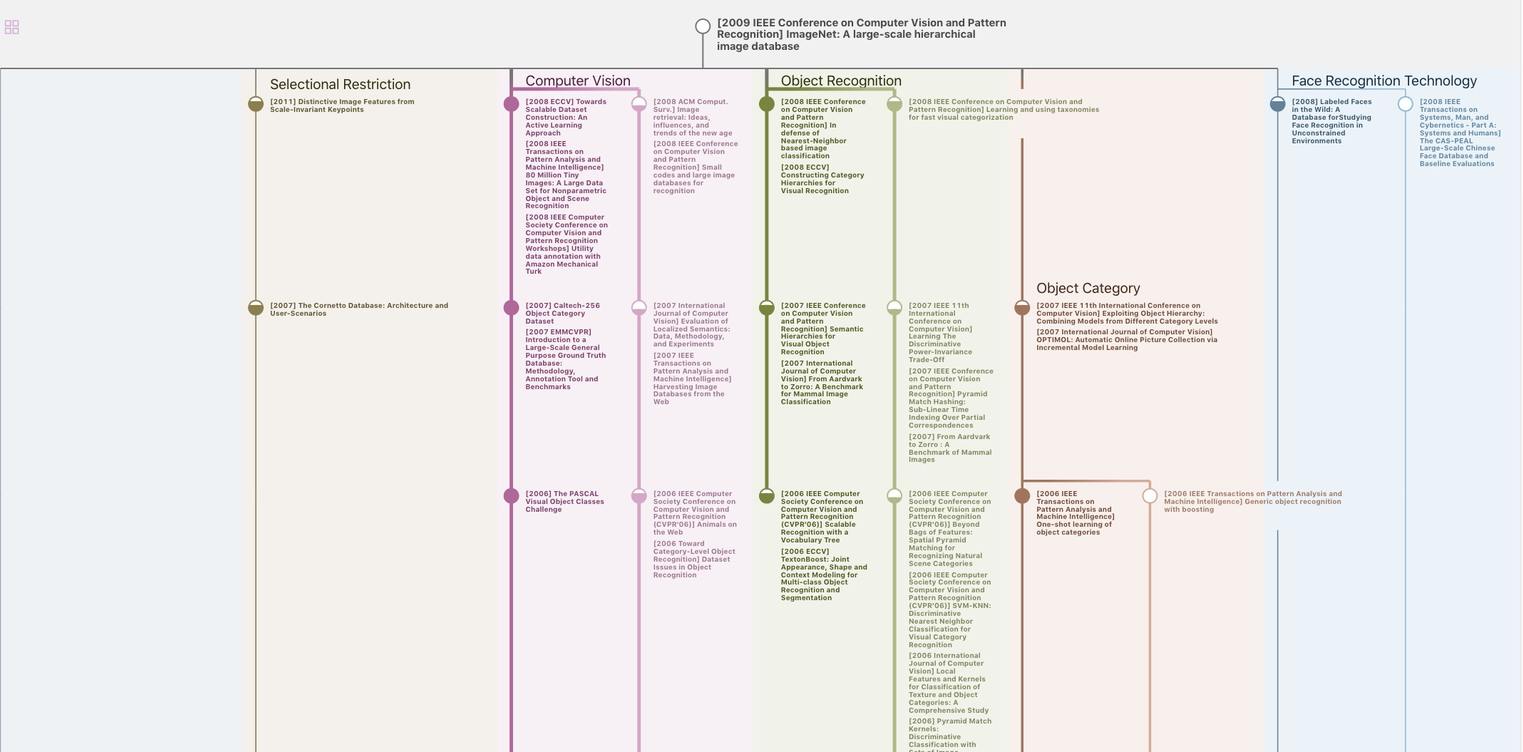
生成溯源树,研究论文发展脉络
Chat Paper
正在生成论文摘要