Fast Inference Beamforming Prediction using Machine Learning
2023 IEEE International Symposium on Antennas and Propagation and USNC-URSI Radio Science Meeting (USNC-URSI)(2023)
摘要
This work introduces an efficient, novel framework using a machine learning (ML) model to estimate beamforming weights on a Raspberry pi microcontroller. Tracking fast-moving targets and dynamically adjusting the weights of each antenna element in a phased array to transfer maximum energy to the target is desired in many beamforming applications. This work exploits ML models' fast output predicting nature to calculate optimum weights. A KNeighborsRegressor (KNR) is embedded into the proposed beamforming framework, which can predict the appropriate beam pattern of a 3×1 fractal array antenna. The process carried out on Raspberry pi has successfully calculated beamforming weights for 100 random targets within 62.8 seconds.
更多查看译文
关键词
KNeighborsRegressor,Machine Learning,Raspberry pi,Fractal Antenna,Beamforming weights
AI 理解论文
溯源树
样例
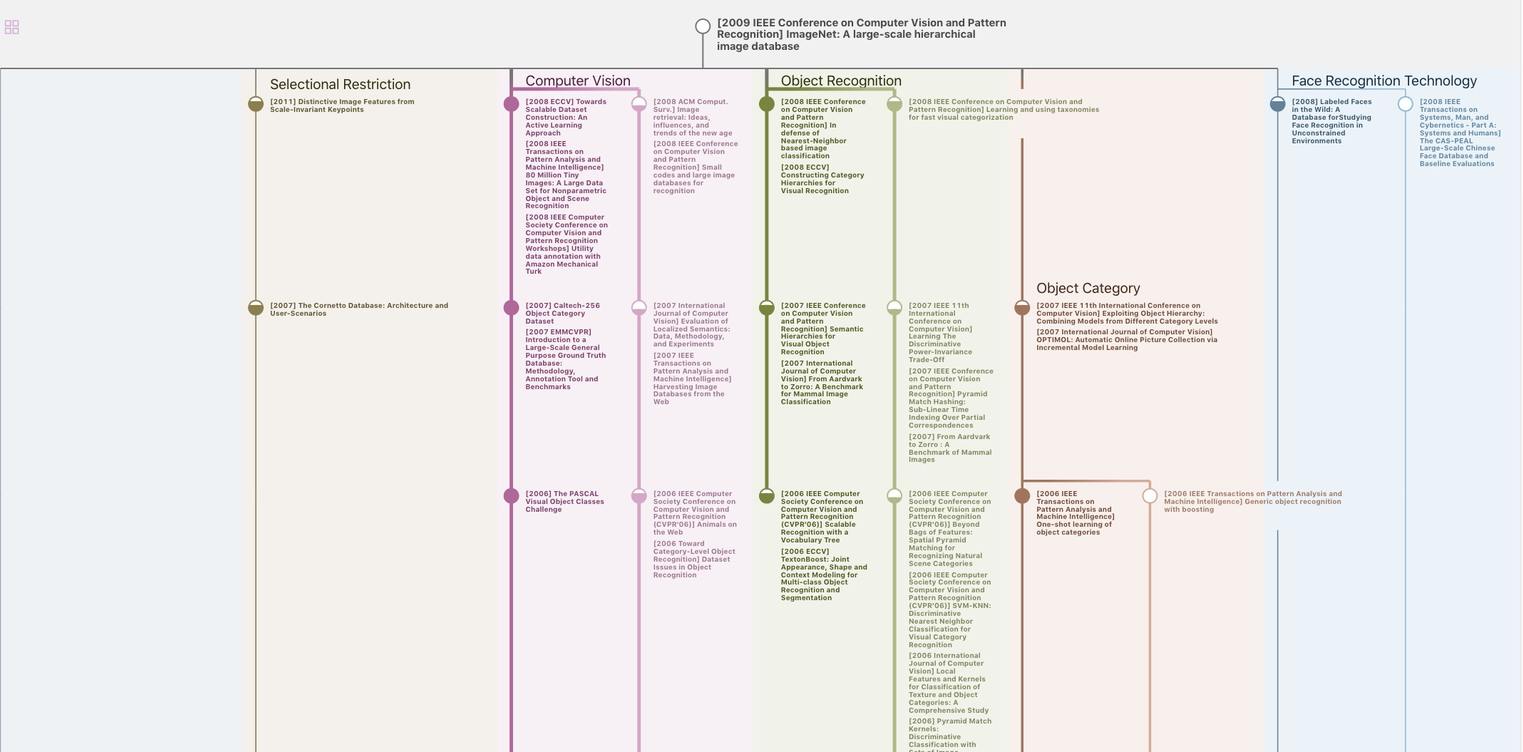
生成溯源树,研究论文发展脉络
Chat Paper
正在生成论文摘要