Teacher-Student Network for Real-World Face Super-Resolution with Progressive Embedding of Edge Information
2023 IEEE International Conference on Image Processing (ICIP)(2023)
摘要
Traditional face super-resolution (FSR) methods trained on synthetic datasets usually have poor generalization ability for real-world face images. Recent work has utilized complex degradation models or training networks to simulate the real degradation process, but this limits the performance of these methods due to the domain differences that still exist between the generated low-resolution images and the real low-resolution images. Moreover, because of the existence of a domain gap, the semantic feature information of the target domain may be affected when synthetic data and real data are utilized to train super-resolution models simultaneously. In this study, a real-world face super-resolution teacher-student model is proposed, which considers the domain gap between real and synthetic data and progressively includes diverse edge information by using the recurrent network’s intermediate outputs. Extensive experiments demonstrate that our proposed approach surpasses state-of-the-art methods in obtaining high-quality face images for real-world FSR.
更多查看译文
关键词
Super-resolution,Face hallucination,Facial priors,techer-student network,edge prior
AI 理解论文
溯源树
样例
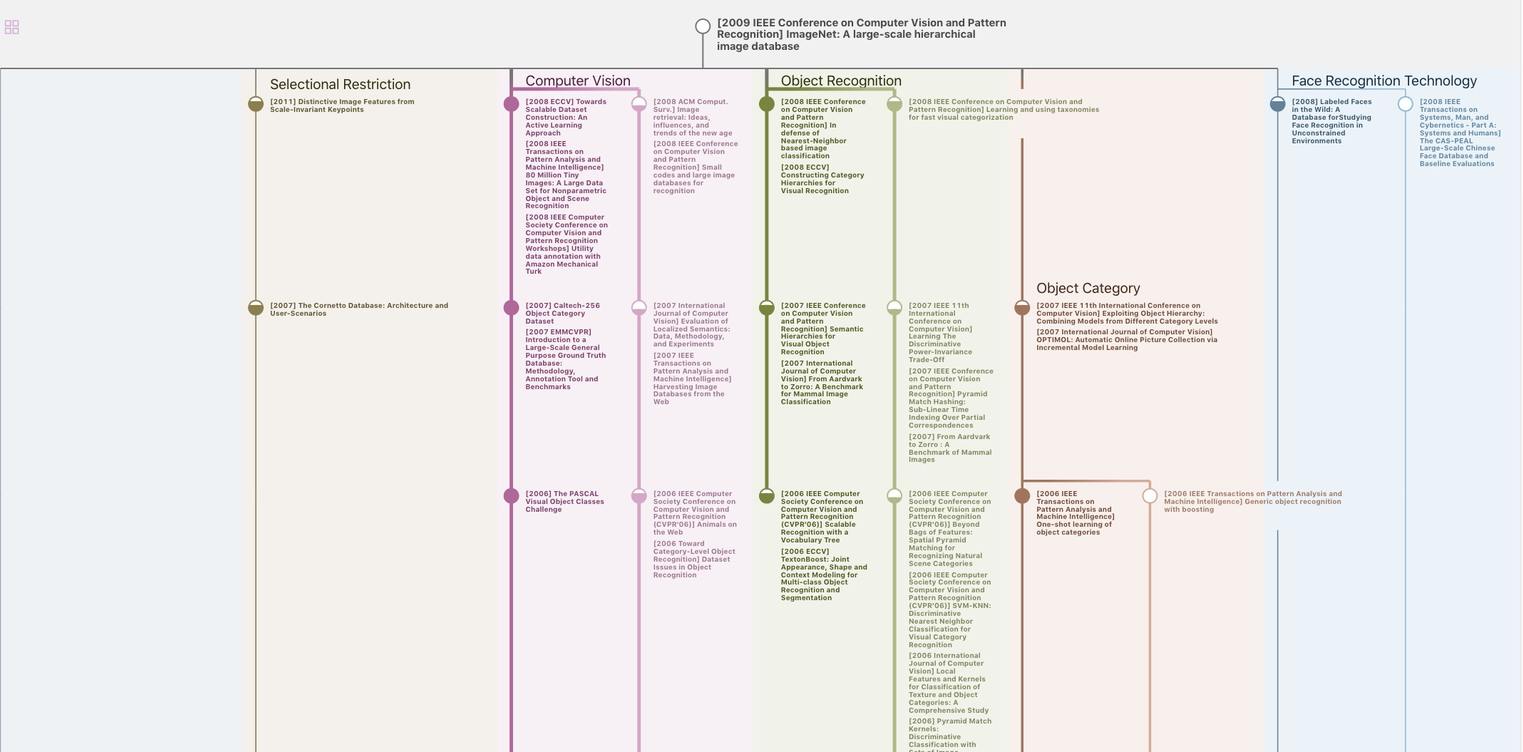
生成溯源树,研究论文发展脉络
Chat Paper
正在生成论文摘要