Learnable snake r-cnn for instance-level biomedical image segmentation
2023 IEEE INTERNATIONAL CONFERENCE ON IMAGE PROCESSING, ICIP(2023)
摘要
Precisely knowing each instance's position and extents is a critical first step in many biological applications. State-of-the-art techniques rely either on deep learning models designed to predict segmentation masks on each Region of Interest (RoI) or on classic active contour methods. The former struggles to precisely delineating boundaries and tends to output masks at low resolutions when the cells/nuclei are very irregular while the latter often needs good initialization and manual setting of parameters, thus limiting their usefulness. To bridge this gap, we introduce Snake R-CNN, a new level of the learnable active contour model that predict boundary on each RoI in a sequent way. To do so, for each RoI, we reformulate the contour deformation task in terms of a hidden state evolution problem and update the evolution process using energy minimization. We learn snake parameterizations per instance in an end-to-end manner, and demonstrate its effectiveness for contour inferences of various cell/nucleus types where consistently higher performances were obtained for comparison against state-of-the-arts.
更多查看译文
关键词
Biomedical segmentation,Deep learning,Active contour,Recurrent neural network,Instance segmentation
AI 理解论文
溯源树
样例
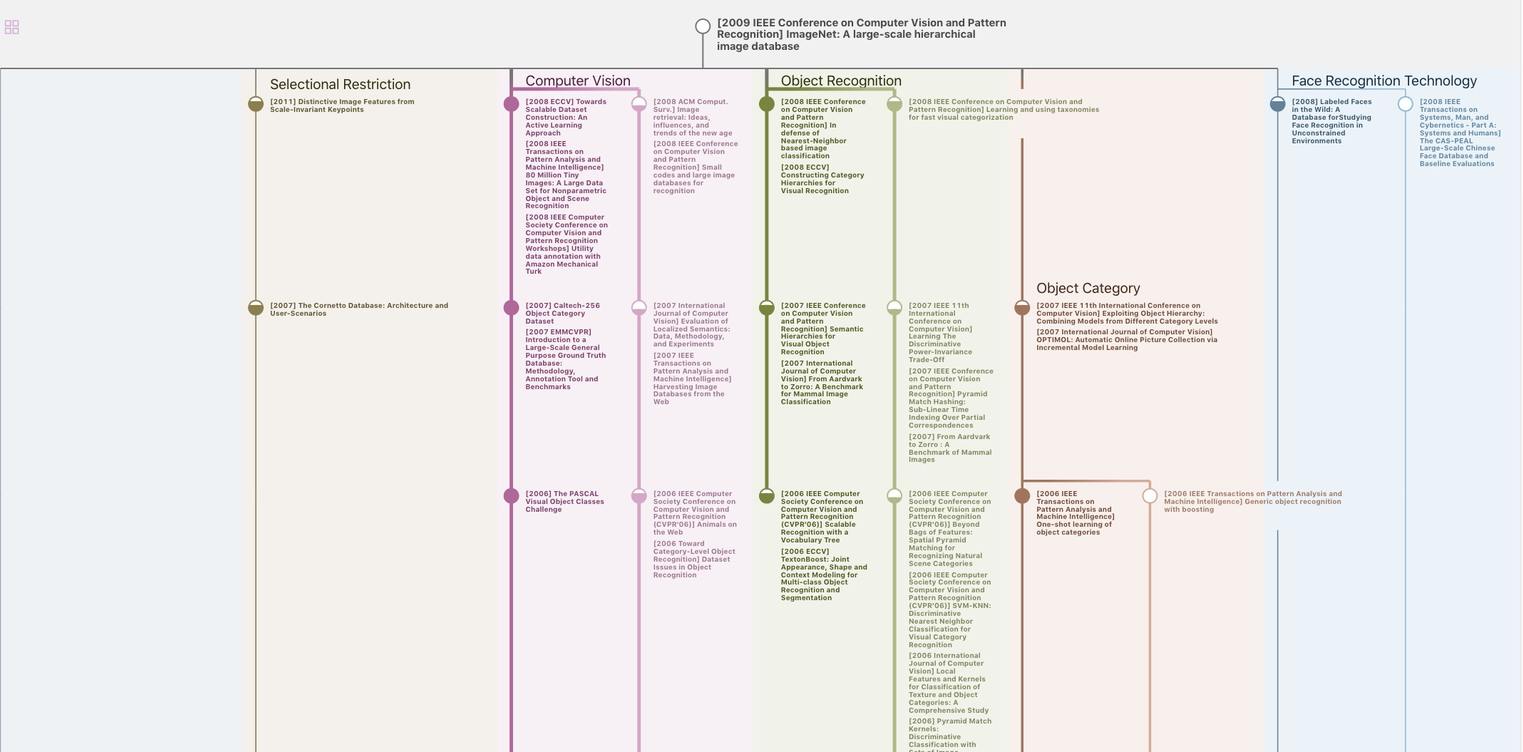
生成溯源树,研究论文发展脉络
Chat Paper
正在生成论文摘要