3D HIPPOCAMPUS SEGMENTATION USING A HOG BASED LOSS FUNCTION WITH MAJORITY POOLING
2023 IEEE INTERNATIONAL CONFERENCE ON IMAGE PROCESSING, ICIP(2023)
摘要
Hippocampus (HC) segmentation plays a key role in diagnosis of predominant neuro-degenerative diseases like Alzheimer's, Parkinson's and common neurological disorders like Epilepsy. In this paper, we propose a solution to the 3D HC segmentation problem from the MRI data using shape driven loss function and attention Unet. In particular, a Histogram of Oriented Gradients (HOG) based formulation is developed to extract shape features. We suggest a pooling technique as a substitute to the histogram calculation for HOG. This is to address the problem that histogram is not derivable thereby making the error in a loss function from histogram unsuitable for back propagation in deep learning models. The performance of our proposed model is validated on two publicly available datasets, namely, HarP and Kulaga-Yoskovitz (KY). Our segmentation accuracy with a dice similarity score of 0.947 and 0.923 in HarP and KY respectively is found to outperform the attention UNet model with only Dice loss, and, a number of state-of-the-art approaches.
更多查看译文
关键词
Hippocampus Segmentation,HOG features,Attention Unet,Majority Pooling,Loss function
AI 理解论文
溯源树
样例
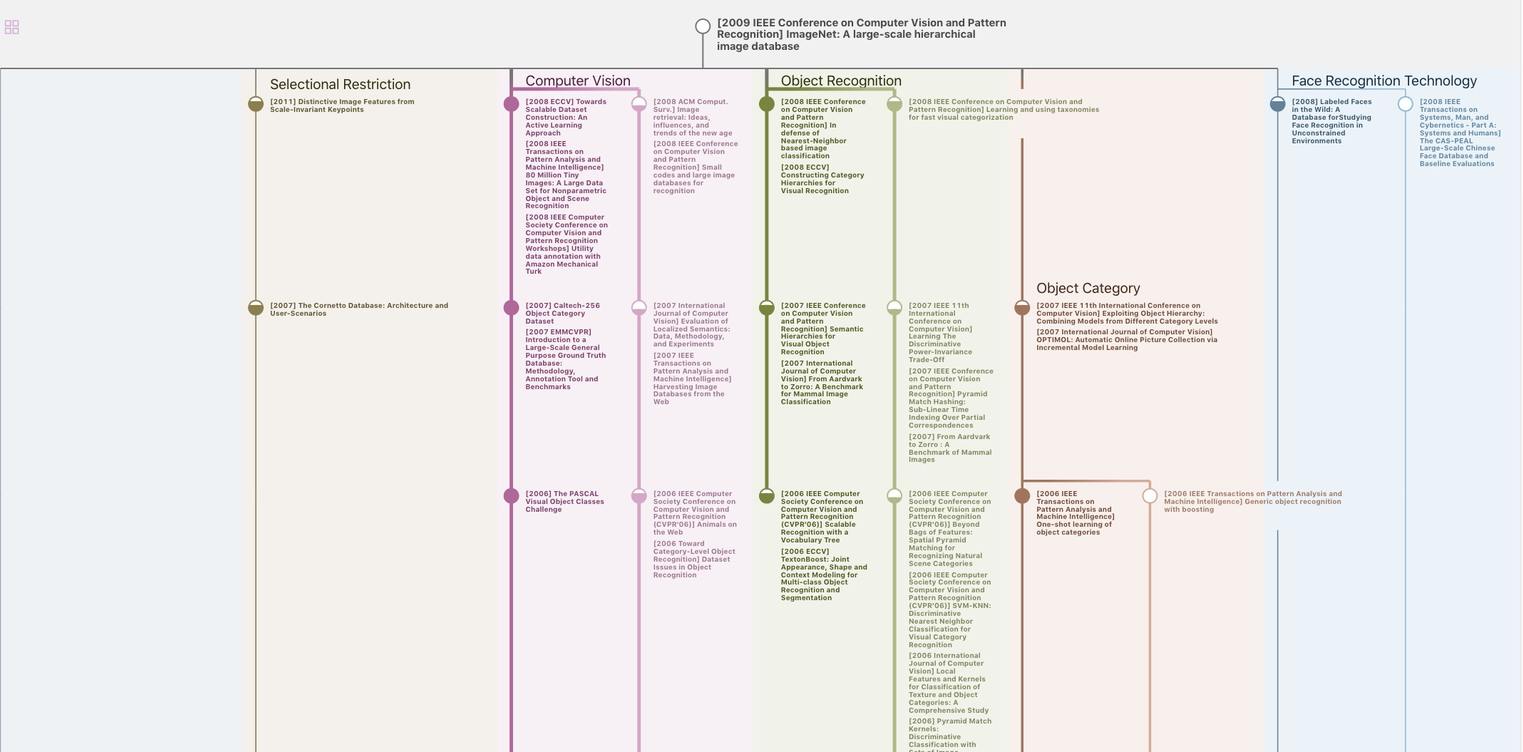
生成溯源树,研究论文发展脉络
Chat Paper
正在生成论文摘要