Attentive deep k-svd network for patch correlated image denoising
2023 IEEE INTERNATIONAL CONFERENCE ON IMAGE PROCESSING, ICIP(2023)
摘要
Techniques of dictionary learning and sparse representation are popular in recent study on image denoising, including classic K-SVD and its variants. The extension of K-SVD to its deep structure learned in an end-to-end way shows the state-of-the-art denoising performance with a great computation efficiency. However, we notice that the current learning framework takes images patches as independent samples, which ignores the inherent correlation among the patches. In this paper, we propose a deep K-SVD denoising network with attention mechanism to enhance the correlation within and among the patches. We impose the two-dimensional correlation on the intermediate parameters during the sparse representation procedure to achieve more smoothing and localstructure enhanced image features. Extensive numerical experiments using public data are conducted. The results on two datasets show that the proposed network achieves an average improvement of 0.81dB in peak signal-to-noise ratio (PSNR), 1.66% in the structural similarity (SSIM) and more than 90% in the convergence rate comparing to its counterpart, which demonstrate the efficiency and the competitive performance of our proposed network.
更多查看译文
关键词
Image denoising,channel and spacial attention,deep learning,sparse representation
AI 理解论文
溯源树
样例
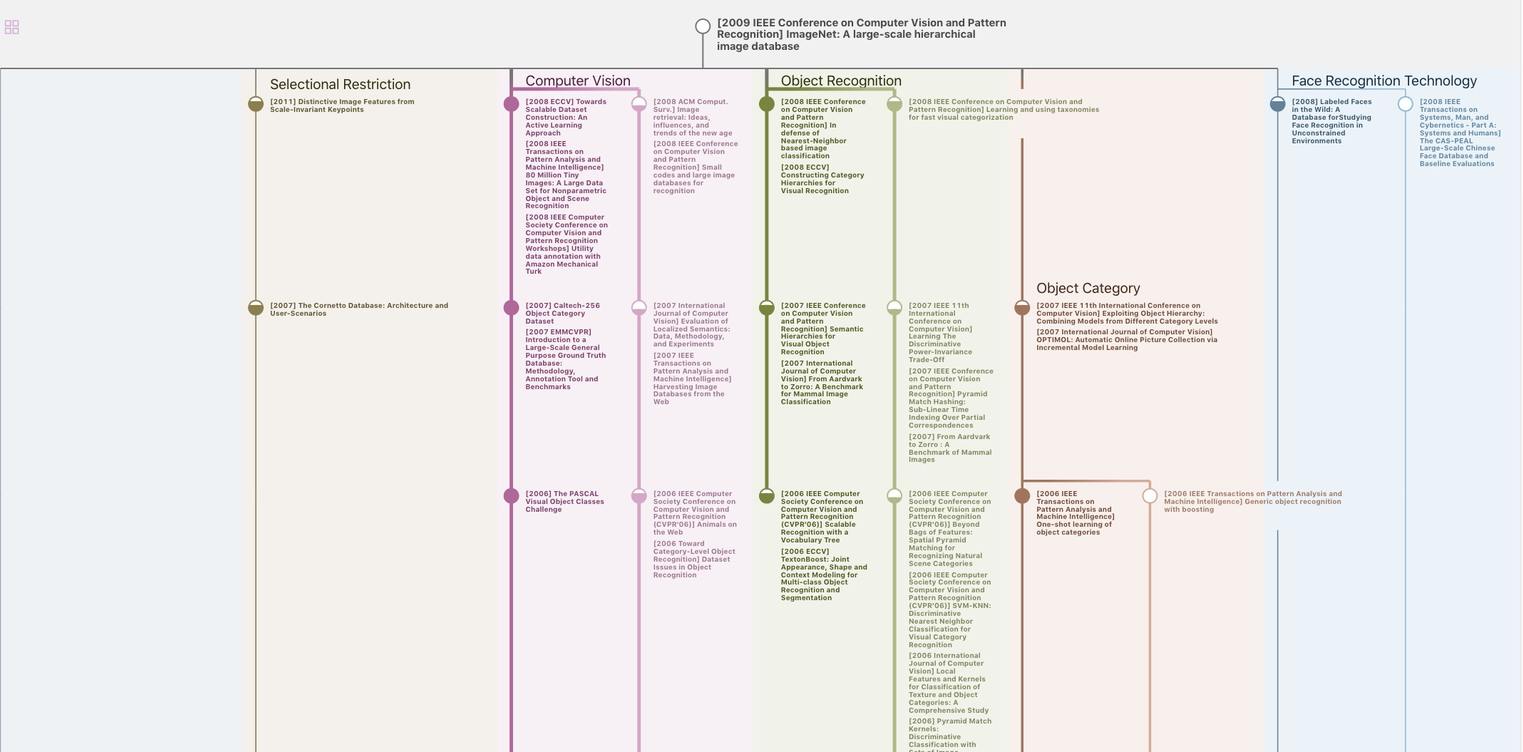
生成溯源树,研究论文发展脉络
Chat Paper
正在生成论文摘要