Deep unsupervised hashing with semantic consistency learning
2023 IEEE INTERNATIONAL CONFERENCE ON IMAGE PROCESSING, ICIP(2023)
摘要
Hashing method has attracted more attention in recent years because of its low storage consumption and high retrieval performance. Most unsupervised hashing methods first construct local similarity structure in high-dimensional feature space, and then learn binary hash codes which maintain similarity structure information. However, this local structure based on pairwise distance will bring false guidance and misguide the hashing model. Besides, previous methods rarely consider the robustness of the hashing model, resulting in the unstable hash codes generated under perturbation. Toward these issues, we propose a novel Semantic Consistency Hashing (SCH). Specifically, to avoid misguidance caused by local similarity structure, SCH converts the similarity structure into the probability distribution and preserves semantic information from the perspective of global data distribution. In addition, to improve the robustness of hash codes, we introduce transformation consistency learning to maximize the similarity of hash codes under different transformations of the same image. Experiments on three popular datasets show that SCH outperforms the state-of-the-art methods.
更多查看译文
关键词
Deep hashing,unsupervised learning,image retrieval
AI 理解论文
溯源树
样例
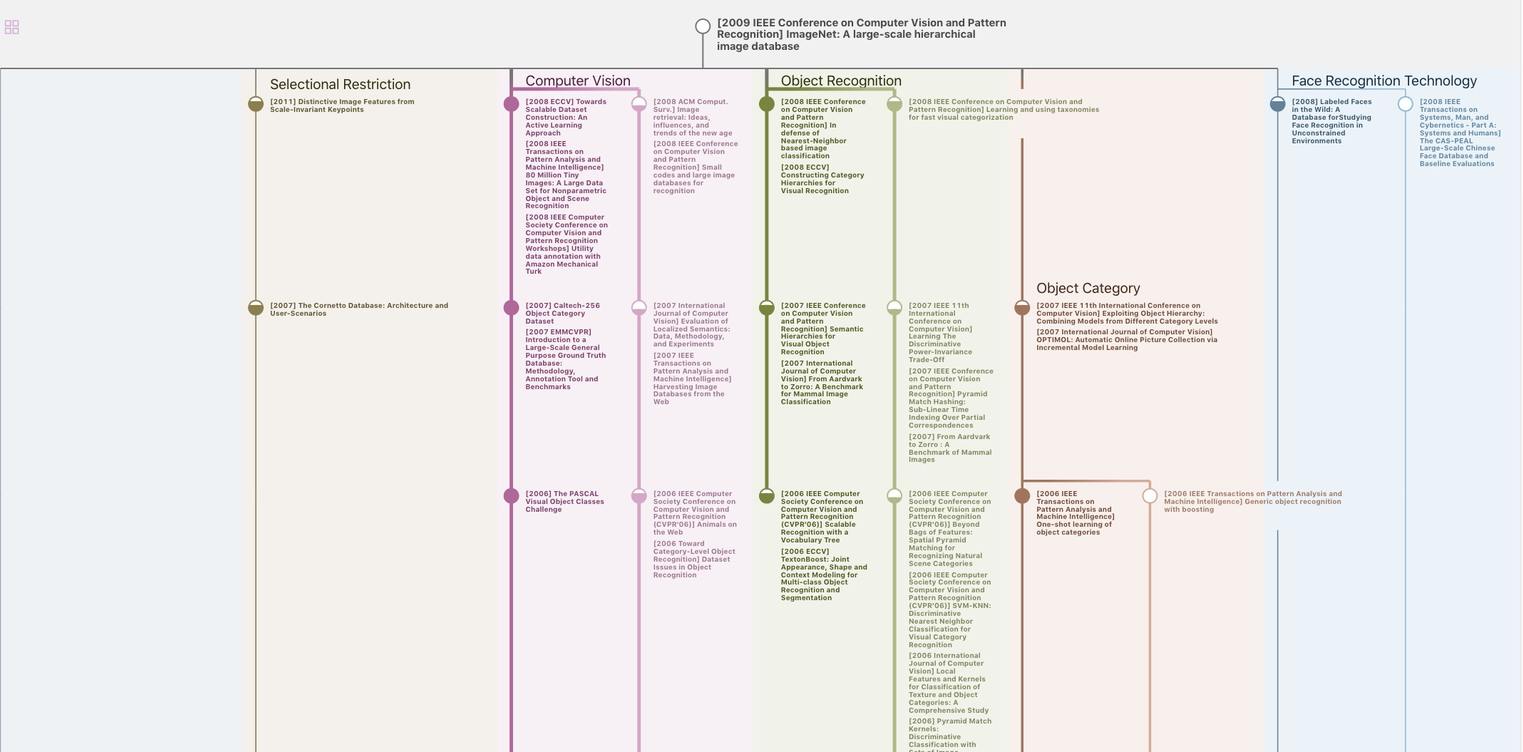
生成溯源树,研究论文发展脉络
Chat Paper
正在生成论文摘要