Using LightGBM with SHAP for predicting and analyzing traffic accidents severity
2023 7th International Conference on Transportation Information and Safety (ICTIS)(2023)
摘要
This paper applied the LightGBM, a machine learning algorithm, to predict traffic accident severity and interpreted the results with the SHAP method. The traffic accident records of a city in Guangdong Province, China in 2016 was used in model training and testing. It employed four indexes, i.e., accuracy, precision, recall, and F1, to evaluate the performance of the LightGBM. The SHAP method was used to explain the influence of individual and interactive influences of some independent variables on the model prediction. The results showed that the LightGBM performed better than other machine learning algorithms, including the support vector machine, naive Bayes, random forest and Xgboost. It was found that visibility, road physics isolation and road alignment are the three most important factors affecting accident severity. Finally, based on the results of the SHAP interpretations, some suggestions of reducing accident severity were provided.
更多查看译文
关键词
Accident severity,LightGBM,SHAP,Machine learning
AI 理解论文
溯源树
样例
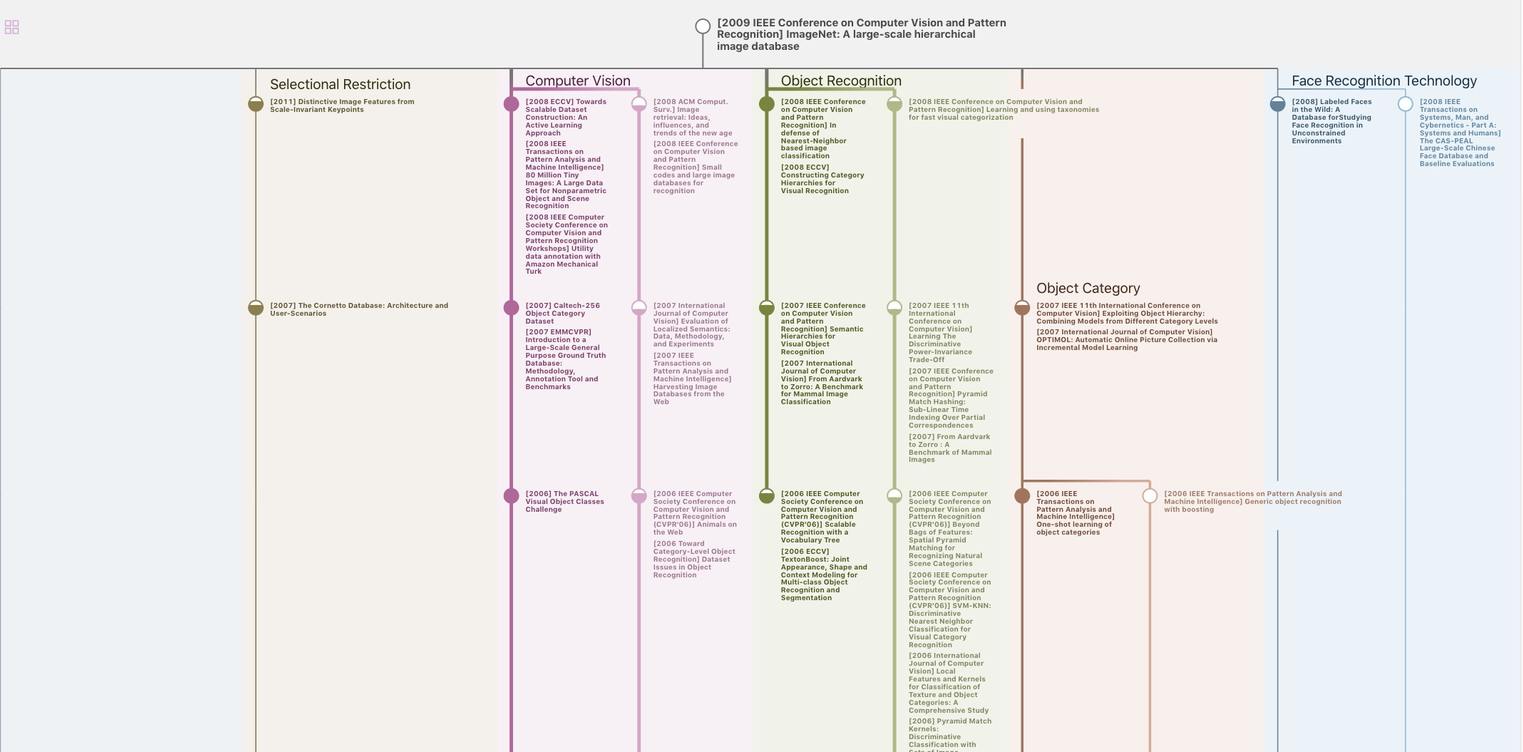
生成溯源树,研究论文发展脉络
Chat Paper
正在生成论文摘要