Detecting Sea Surface Slicks using Automated Machine Learning
OCEANS 2023 - Limerick(2023)
摘要
Sea surface slicks naturally occur on the ocean. They have important physical, biogeochemical and ecological functionalities. The automatic detection of slicks on images is useful in many scenarios, e.g., to evaluate long time series of images. However, to the best of our knowledge, no methods for the automatic recognition of sea surface slicks on images exist. In this work, a binary classifier is developed that recognises if a slick is present on an image with the help of Automated Machine Learning (AutoML). AutoML automatically finds a machine learning pipeline for a given problem. Two AutoML approaches are compared: auto-sklearn and AutoKeras. Images from seven sites were available from the North and Baltic Seas. Initially, one site was used for training and testing. It was found that the AutoKeras method demonstrated superior performance compared to the auto-sklearn approach in both f1-score and balanced accuracy. After further optimising the model found by AutoKeras and training it on data from the additional sites, the final model reaches an f1-score of 0.924 and a balanced accuracy of 0.954 on the test dataset. Furthermore, the model achieved an f1-score of 0.710 and a balanced accuracy of 0.925 on a holdout dataset only containing images from a site which was excluded from the training. This shows that the model is not only able to reliably recognise sea surface slicks but it also generalises fairly well to unseen data.
更多查看译文
关键词
Automated Machine Learning,AutoML,balanced accuracy,biogeochemical functionalities,ecological functionalities,important physical functionalities,machine learning pipeline,recognise sea surface slicks,slick
AI 理解论文
溯源树
样例
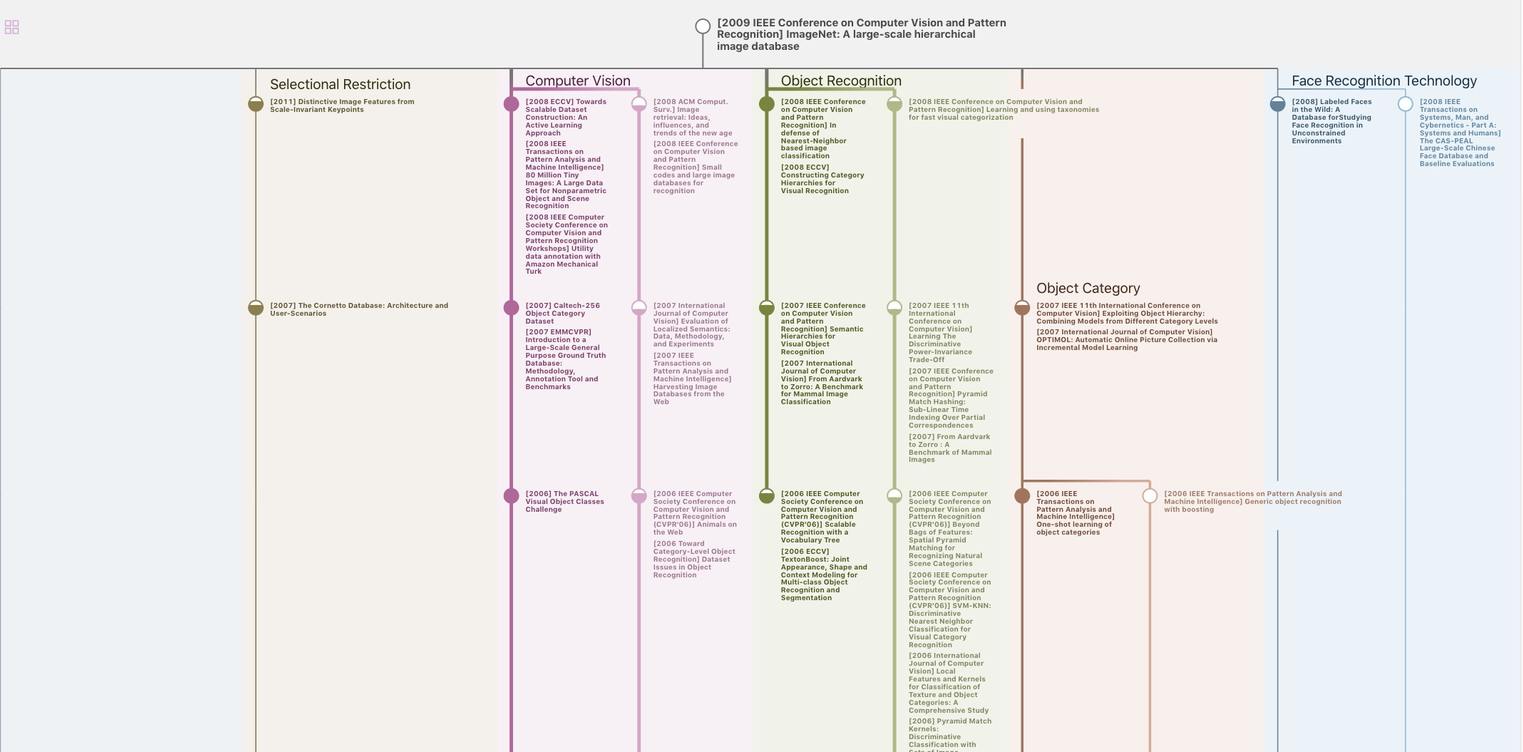
生成溯源树,研究论文发展脉络
Chat Paper
正在生成论文摘要