Weed detection based on improved Yolov5
2023 8th International Conference on Intelligent Computing and Signal Processing (ICSP)(2023)
摘要
The scale of grain production affects human life and development. With the continuous expansion of cultivated land, the reproductive ability of weeds to mutiply gradually increases, which affects the growth of crops. If weeds are not treated properly managed, they can also allow spread indiscriminately in the field and reduce crop yields. The growth ability of weeds is stronger than that of crops, and there are many types of weeds with a wide of species diversity. To solve the problem that the existing weed detection methods cannot detect and classify weeds accurately and quickly, a deep learning method based on improved Yolov5 was designed for weed detection. By replacing the 3 × 3 convolution with multi-head self-attention (MHSA) in the Yolov5's backbone, the accuracy of weed detection is improved. The experimental results show that the improved Yolov5 weed detection algorithm achieves 51.4% accuracy. Compared with the original Yolov5 model, the calculation amount is 3 points less than the original, and total time required is slightly shorter, which improves the accuracy of weed classification and positioning.
更多查看译文
关键词
weed detection,Yolov5,deep learning,multi-head self-Attention
AI 理解论文
溯源树
样例
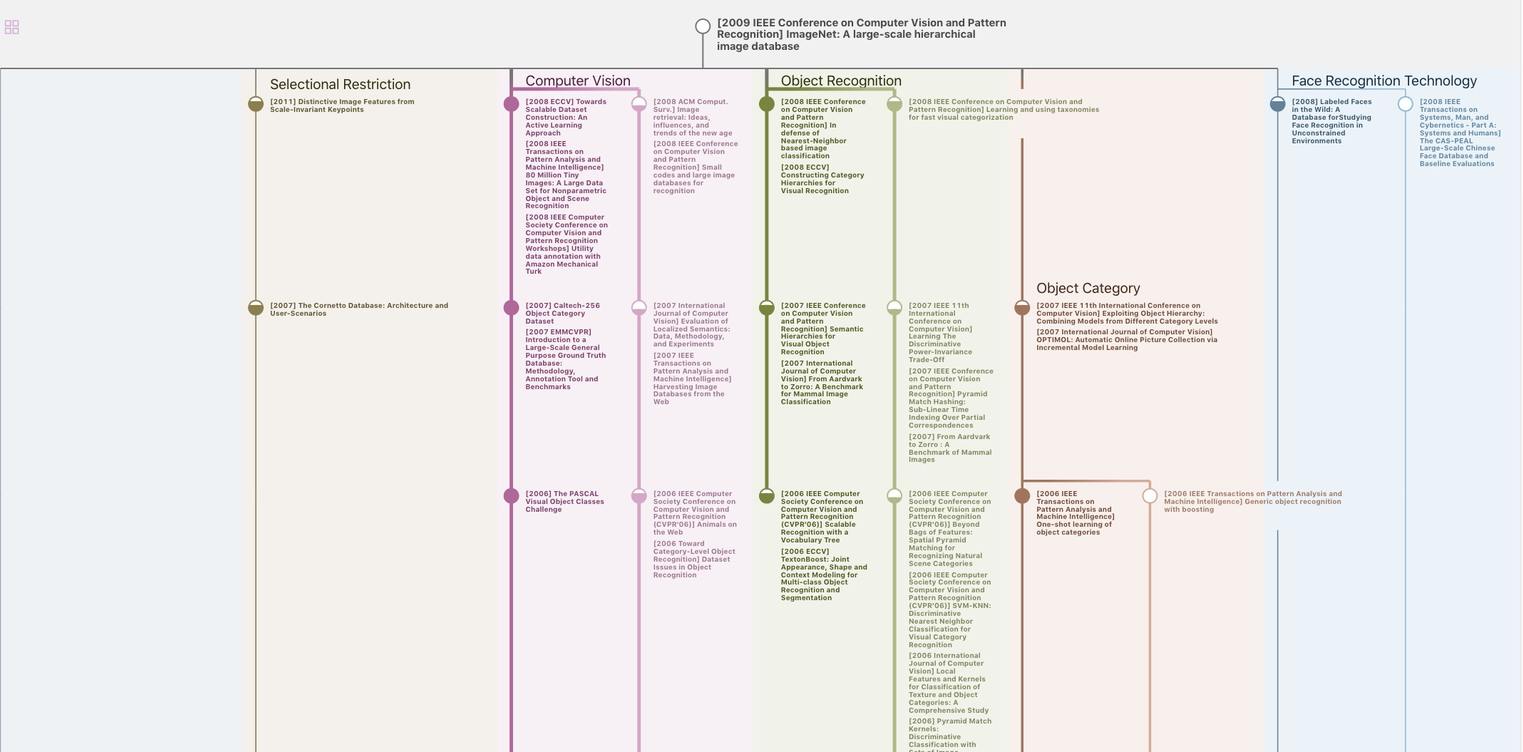
生成溯源树,研究论文发展脉络
Chat Paper
正在生成论文摘要