Explainability of Machine Learning Models for Hydrological Time Series Forecasting: The Case of Neuro-Fuzzy Approaches
2023 3rd International Conference on Electrical, Computer, Communications and Mechatronics Engineering (ICECCME)(2023)
摘要
This research evaluates the explainability of rules obtained in Neuro-Fuzzy (NF) models in hydrological time series forecasting. Thus, three NF models (Adaptive Network-based Fuzzy Inference System (ANFIS) and two versions of the Self-Identification Neuro-Fuzzy Inference Model (SINFIM)) were developed, considering the rain-runoff modeling as a particular case. The ANFIS model had the lowest performance, with many fuzzy rules challenging to interpret. The SINFIM 01 model performed best, but not all fuzzy rules were well explained. However, the SINFIM 02 model had a lower performance than the SINFIM 01 model but with more explainable fuzzy rules. With the examples developed, it is highlighted that within the NF models, it is necessary to focus on their performance during the forecast and look for a trade-off with explainability, thus taking advantage of their characteristic of more transparency than the black-box models.
更多查看译文
关键词
Explainable Machine Learning,Neuro-Fuzzy models,Hydrological time series forecasting
AI 理解论文
溯源树
样例
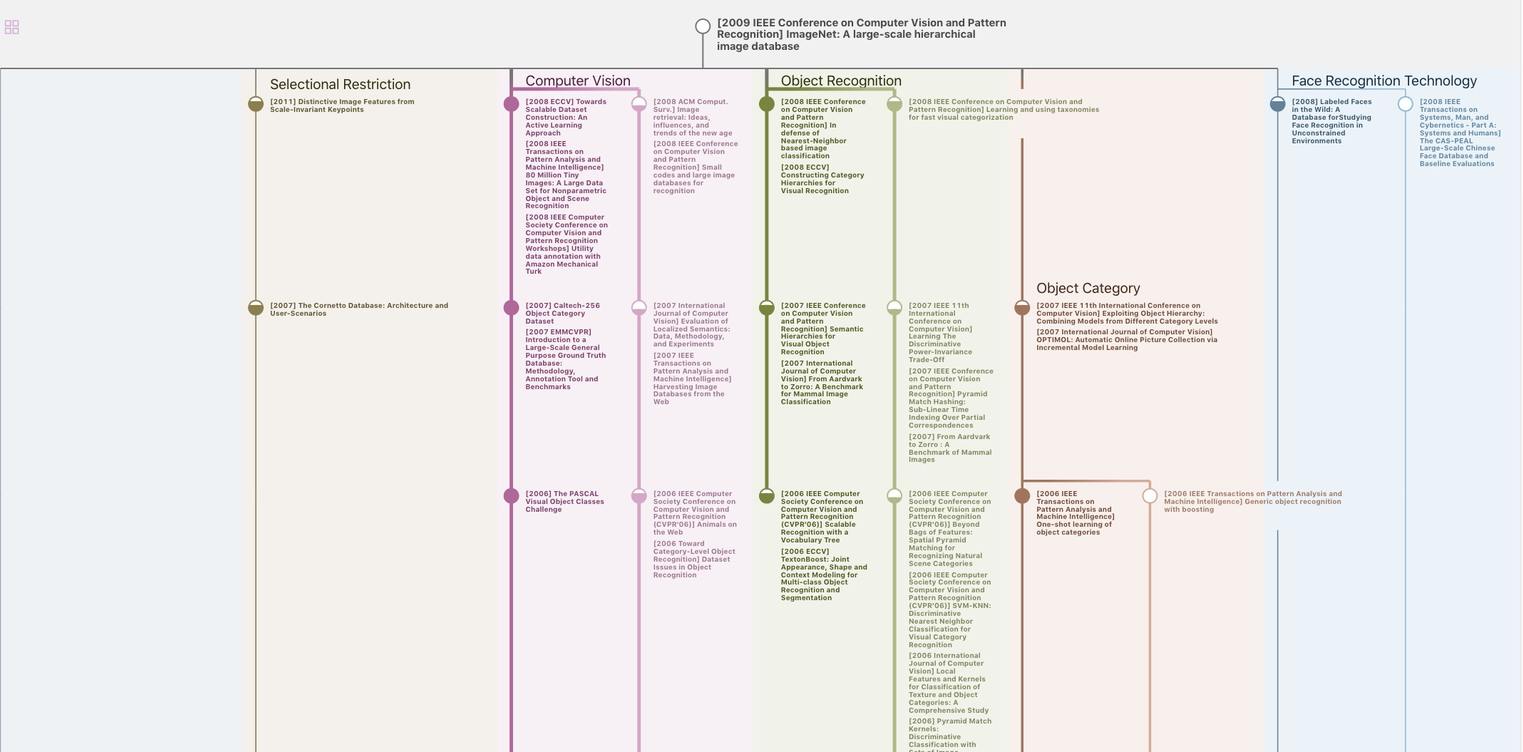
生成溯源树,研究论文发展脉络
Chat Paper
正在生成论文摘要