Text Summarization from Judicial Records using Deep Neural Machines
2022 International Conference on Electrical, Computer, Communications and Mechatronics Engineering (ICECCME)(2022)
摘要
Courts are generating a large amount of data as legal proceedings. In Pakistan, the ratio of cases that are registered every year and the judgments made is very high mainly due to the time it takes to prepare for a trial. Text Summarization is one of the applications of Natural Language Processing (NLP) that can be used to provide a brief overview of the judgment to both the lawyers and the judges which will help save a lot of their precious time, and hence speedy justice can be provided to the people. Transformer-based models in NLP are a benchmark in solving sequence-to-sequence modeling problems. A downside of these powerful machines is that training a model demands high computation power. We have shown that fine-tuning a pre-trained legal Longformer Encoder-Decoder (LED) transformer model on a downstream task provides better accuracy scores on Australian judgments and our prepared datasets from the Supreme Court of Pakistan (SCP) and Islamabad High Court of Pakistan (IHCP). ROUGE, a commonly used metric in sequence modeling, is used to evaluate the trained model. For the Australian judgments, our approach exhibited a significant improvement for ROUGE-1 and ROUGE-2 scores of 37.97% and 20.04%. For our prepared dataset, our approach produced a ROUGE-1 score of 53.11%, a ROUGE-2 score of 32.12%, and a ROUGE-L score of 34.09%.
更多查看译文
关键词
judicial records,text
AI 理解论文
溯源树
样例
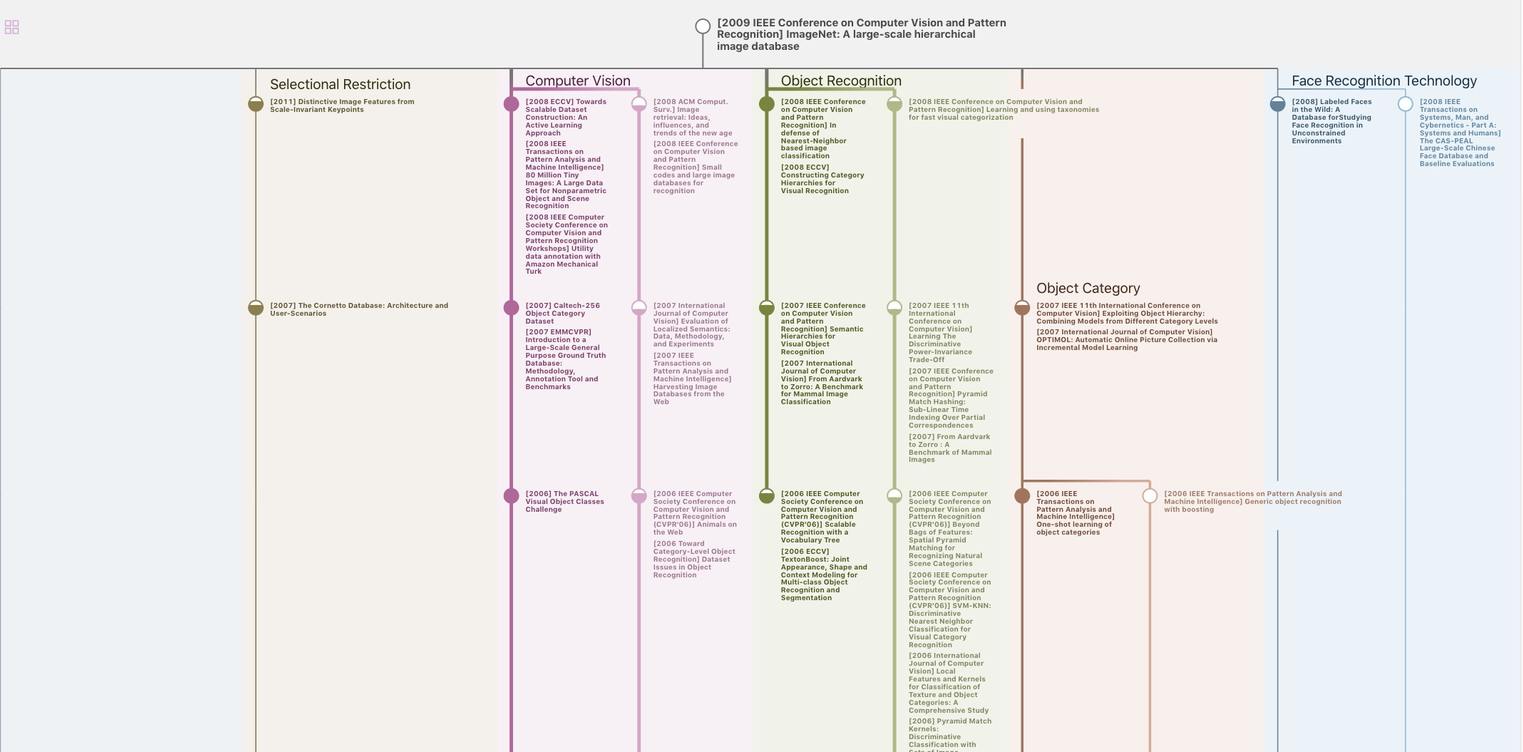
生成溯源树,研究论文发展脉络
Chat Paper
正在生成论文摘要