A Computing-in-Memory-based One-Class Hyperdimensional Computing Model for Outlier Detection
CoRR(2023)
摘要
In this work, we present ODHD, an algorithm for outlier detection based on
hyperdimensional computing (HDC), a non-classical learning paradigm. Along with
the HDC-based algorithm, we propose IM-ODHD, a computing-in-memory (CiM)
implementation based on hardware/software (HW/SW) codesign for improved latency
and energy efficiency. The training and testing phases of ODHD may be performed
with conventional CPU/GPU hardware or our IM-ODHD, SRAM-based CiM architecture
using the proposed HW/SW codesign techniques. We evaluate the performance of
ODHD on six datasets from different application domains using three metrics,
namely accuracy, F1 score, and ROC-AUC, and compare it with multiple baseline
methods such as OCSVM, isolation forest, and autoencoder. The experimental
results indicate that ODHD outperforms all the baseline methods in terms of
these three metrics on every dataset for both CPU/GPU and CiM implementations.
Furthermore, we perform an extensive design space exploration to demonstrate
the tradeoff between delay, energy efficiency, and performance of ODHD. We
demonstrate that the HW/SW codesign implementation of the outlier detection on
IM-ODHD is able to outperform the GPU-based implementation of ODHD by at least
331.5x/889x in terms of training/testing latency (and on average 14.0x/36.9x in
terms of training/testing energy consumption.
更多查看译文
关键词
Hyperdimensional Computing,Outlier Detection,Computing-in-Memory,Hardware/Software Codesign
AI 理解论文
溯源树
样例
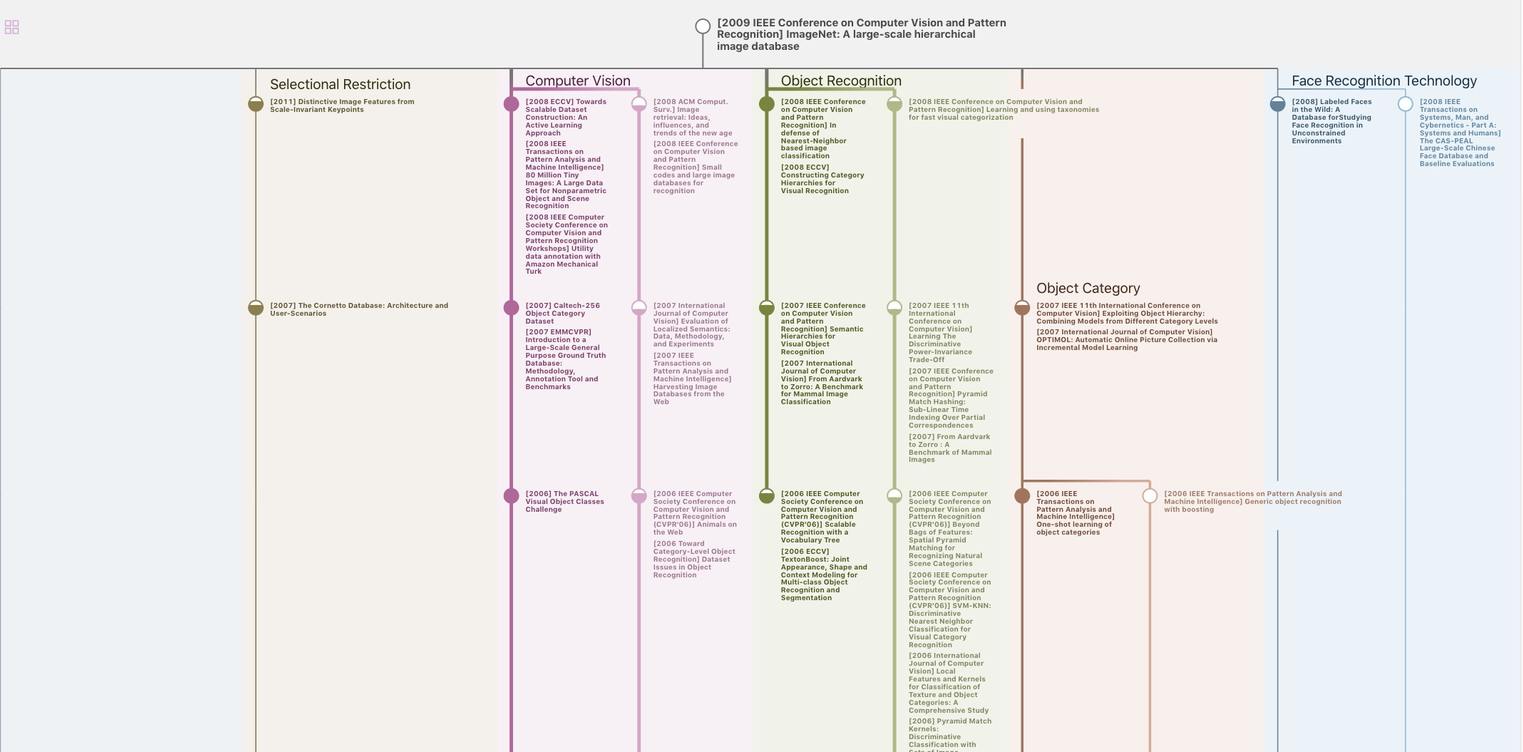
生成溯源树,研究论文发展脉络
Chat Paper
正在生成论文摘要