Neural Texture Puppeteer: A Framework for Neural Geometry and Texture Rendering of Articulated Shapes, Enabling Re-Identification at Interactive Speed
2024 IEEE/CVF Winter Conference on Applications of Computer Vision Workshops (WACVW)(2023)
摘要
In this paper, we present a neural rendering pipeline for textured articulated shapes that we call Neural Texture Puppeteer. Our method separates geometry and texture encoding. The geometry pipeline learns to capture spatial relationships on the surface of the articulated shape from ground truth data that provides this geometric information. A texture auto-encoder makes use of this information to encode textured images into a global latent code. This global texture embedding can be efficiently trained separately from the geometry, and used in a downstream task to identify individuals. The neural texture rendering and the identification of individuals run at interactive speeds. To the best of our knowledge, we are the first to offer a promising alternative to CNN- or transformer-based approaches for re-identification of articulated individuals based on neural rendering. Realistic looking novel view and pose synthesis for different synthetic cow textures further demonstrate the quality of our method. Restricted by the availability of ground truth data for the articulated shape's geometry, the quality for real-world data synthesis is reduced. We further demonstrate the flexibility of our model for real-world data by applying a synthetic to real-world texture domain shift where we reconstruct the texture from a real-world 2D RGB image. Thus, our method can be applied to endangered species where data is limited. Our novel synthetic texture dataset NePuMoo is publicly available to inspire further development in the field of neural rendering-based re-identification.
更多查看译文
关键词
Neural Texture,Developments In The Field,Real-world Data,RGB Images,Geometric Information,Real-world Images,Latent Code,Global Code,Functional Form,Local Features,Input Image,Single Image,Rigid Body,Object Motion,Variational Autoencoder,Camera Position,Camera View,Real-world Examples,Vision Transformer,Tracking Scenarios,Texture Map,Keypoint Locations,Self-supervised Manner,Tracking Applications,Qualitative Examples
AI 理解论文
溯源树
样例
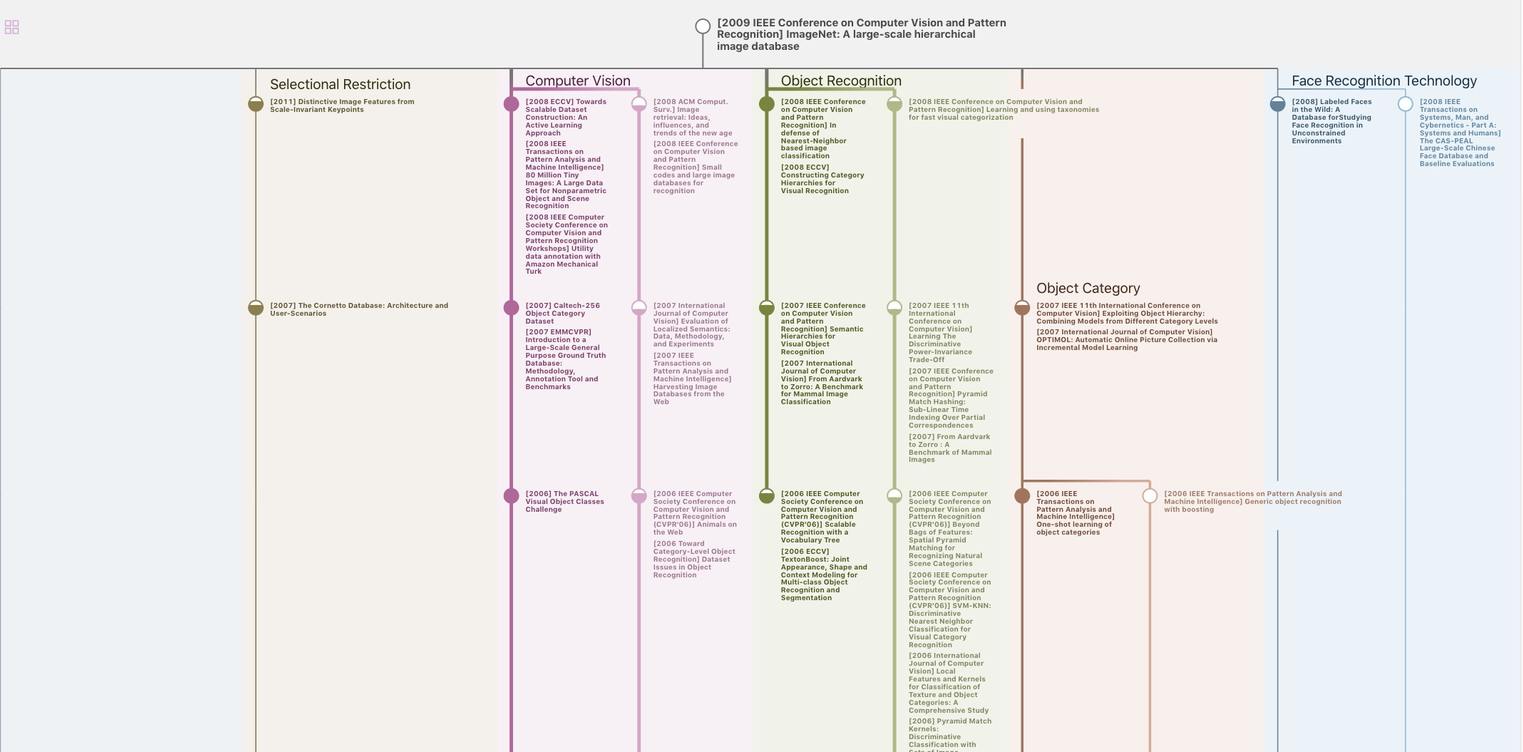
生成溯源树,研究论文发展脉络
Chat Paper
正在生成论文摘要