Reinforcement Learning with thermal fluctuations at the nano-scale
arxiv(2023)
摘要
Reinforcement Learning offers a framework to learn to choose actions in order to achieve some goal. However, at the nano-scale, thermal fluctuations hamper the learning process. We show that in this regime, while optimal actions should bring an improvement proportional to the small ratio of the applied force times a length-scale over the temperature, the learned improvement is smaller and proportional to the square of this small ratio. Consequently, the efficiency of learning, which compares the learning improvement to the theoretical optimal improvement, drops to zero. Nevertheless, we show how to circumvent these limitations by using actions learned at a lower temperature. Our results are illustrated with simulations of the control of small particle clusters, and should apply to a wide class of other problems that can also be formulated as a Markov Decision Processes such as nano-navigation and nano-machine actuation.
更多查看译文
AI 理解论文
溯源树
样例
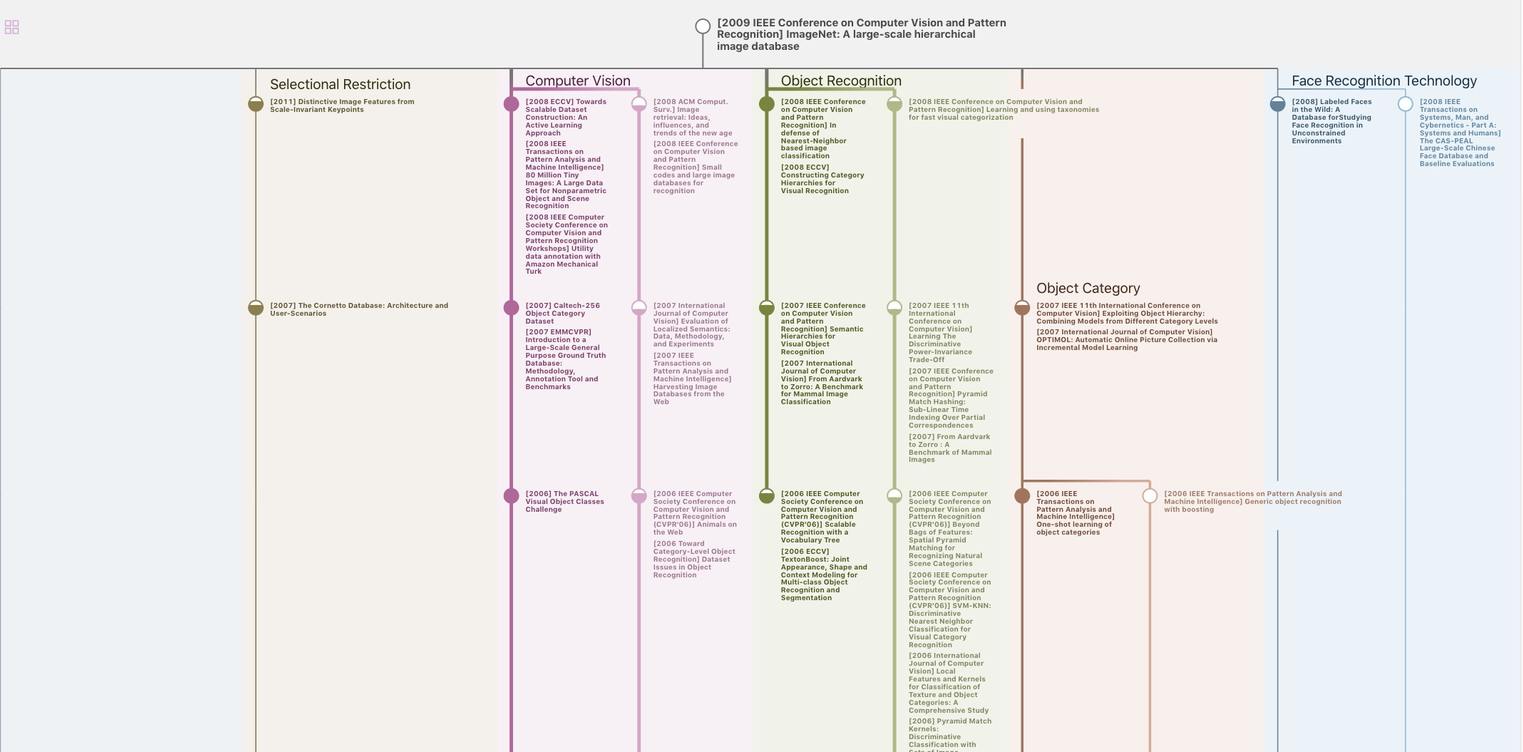
生成溯源树,研究论文发展脉络
Chat Paper
正在生成论文摘要