Learning Power System Dynamics with Nearly-Hamiltonian Neural Network
2023 IEEE Power & Energy Society General Meeting (PESGM)(2023)
摘要
The ability to learn power system dynamic model and predict transient trajectories using data is crucial to realizing closed-loop control of the system with artificial intelligence. This paper proposes a Nearly-Hamiltonian neural network to predict transient trajectories and dynamic parameters of the power system by embedding energy conservation laws in the proposed neural network architecture. This inductive bias empowers the proposed model to learn the power system dynamics without explicitly using the exact functional form of the power system dynamic equations. The numerical study results on the single machine infinite bus system show that the proposed model produces accurate system trajectories and damping coefficient predictions. Furthermore, the proposed model significantly outperforms the baseline and Hamiltonian neural network.
更多查看译文
关键词
Hamiltonian system, Nearly-Hamiltonian neural network, power system dynamics
AI 理解论文
溯源树
样例
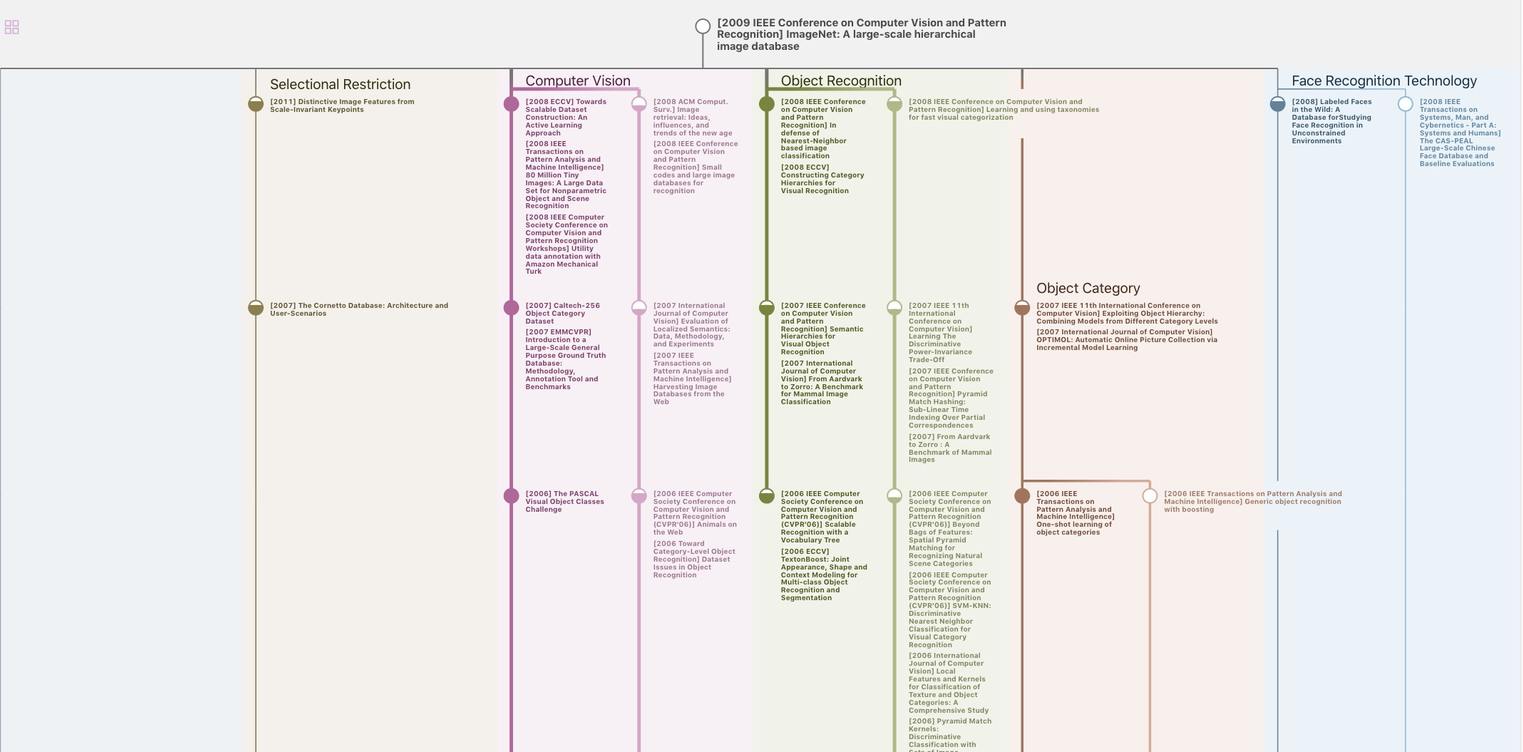
生成溯源树,研究论文发展脉络
Chat Paper
正在生成论文摘要